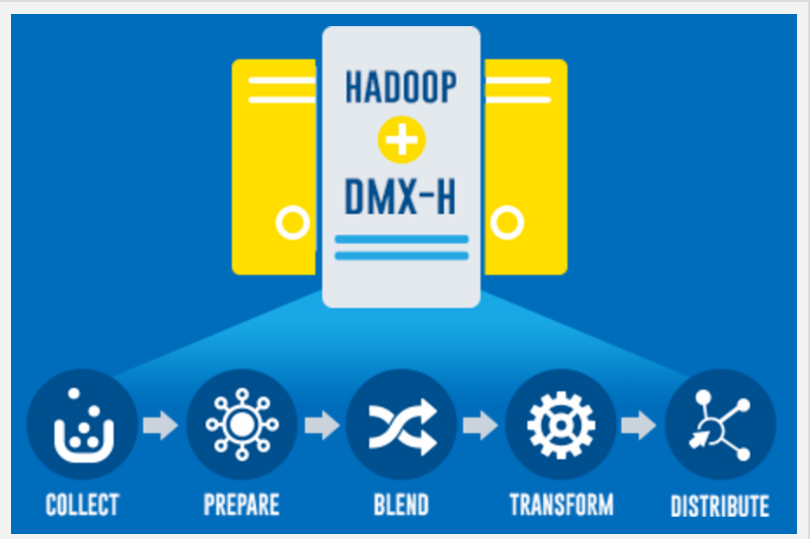
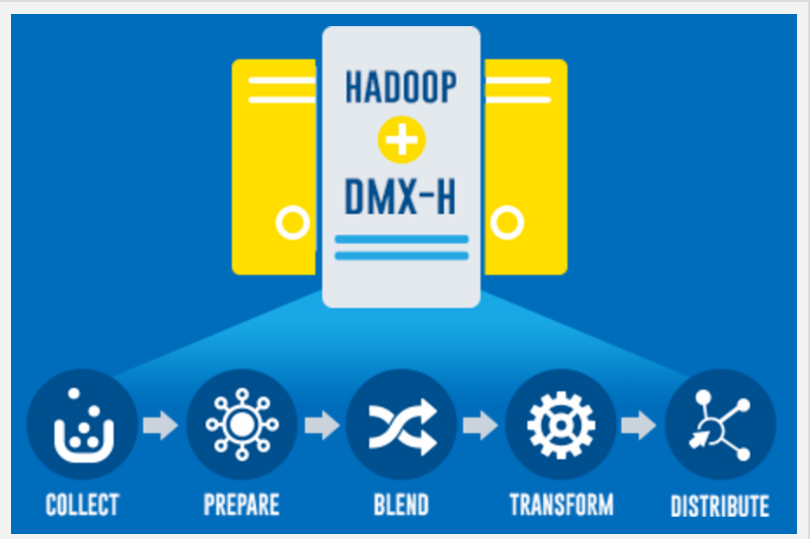
Preparing data for analysis in the age of Big Data, with multiple data types and sources and severe time restraints, are radically different than traditional extract, transform load (ETL) procedures that have existed for a long time. For Big Data, the pipeline becomes collect, prepare, blend, transform and distribute (see figure above). In “Unpacking the New Requirements for Data Prep & Integration”, the first of two Professional Alerts on the subject, Wikibon Big Data & Analytics Analyst George Gilbert discusses these new requirements in detail.
The traditional ETL pipeline was built with the expectation of exactly what data would be loaded, what form it was in, and what questions would be asked in the analysis. That made it efficient but inflexible. Big Data upends that model. The need for ETL grows not just in proportion to the volume of data but also in the variety of machine-generated data that needs to be incorporated.
Gilbert discusses several specific areas in which ETL must change:
In his second Alert, “Informatica & Integration vs Specialization & the Rest”, Gilbert goes into greater depth, providing notes on the leading choices, starting with individual tools such as the Apache Kafka message queue, Waterline Data, Inc., and Alation, Inc. He defines the place of each of these vendors in an overall roll-your-own Big Data pipeline. He then discusses the integrated choices, starting with Informatica Corp., which has the most complete tool for Big Data integration. Its ETL system covers almost exactly the same ground as its traditional ETL for structured data does, providing everything up to analysis. Syncsort Inc. and Talend, Inc., provide less complete but still useful integrated tool sets. Pentaho Corp. provides the most complete stack, from data prep and integration through analytics.
IT practitioners, Gilbert writes, should collect the requirements from each constituency involved in building the new analytic pipeline. If an integrated product such as Informatica or Pentaho does not meet the requirements, they should evaluate the amount of internal integration work they can support and whether the speed of the final pipeline can deliver on its performance goals.
THANK YOU