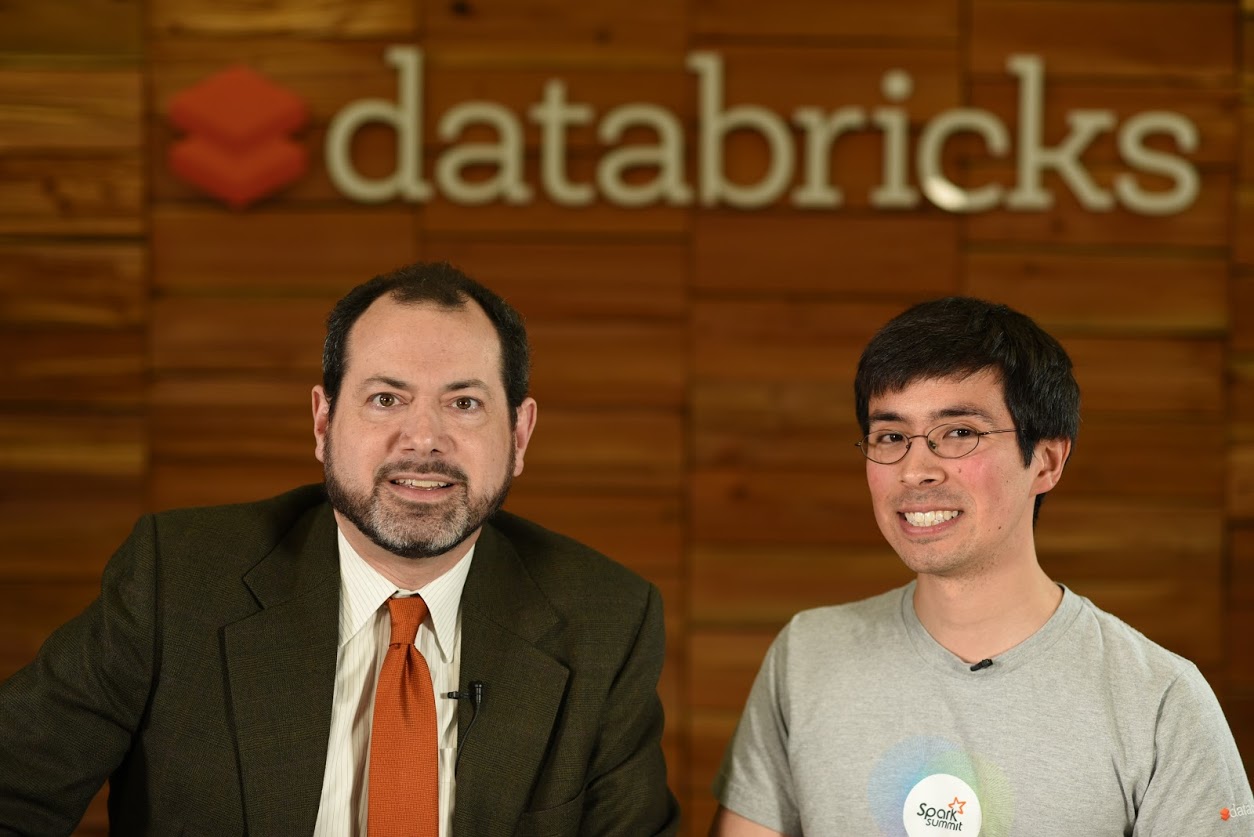
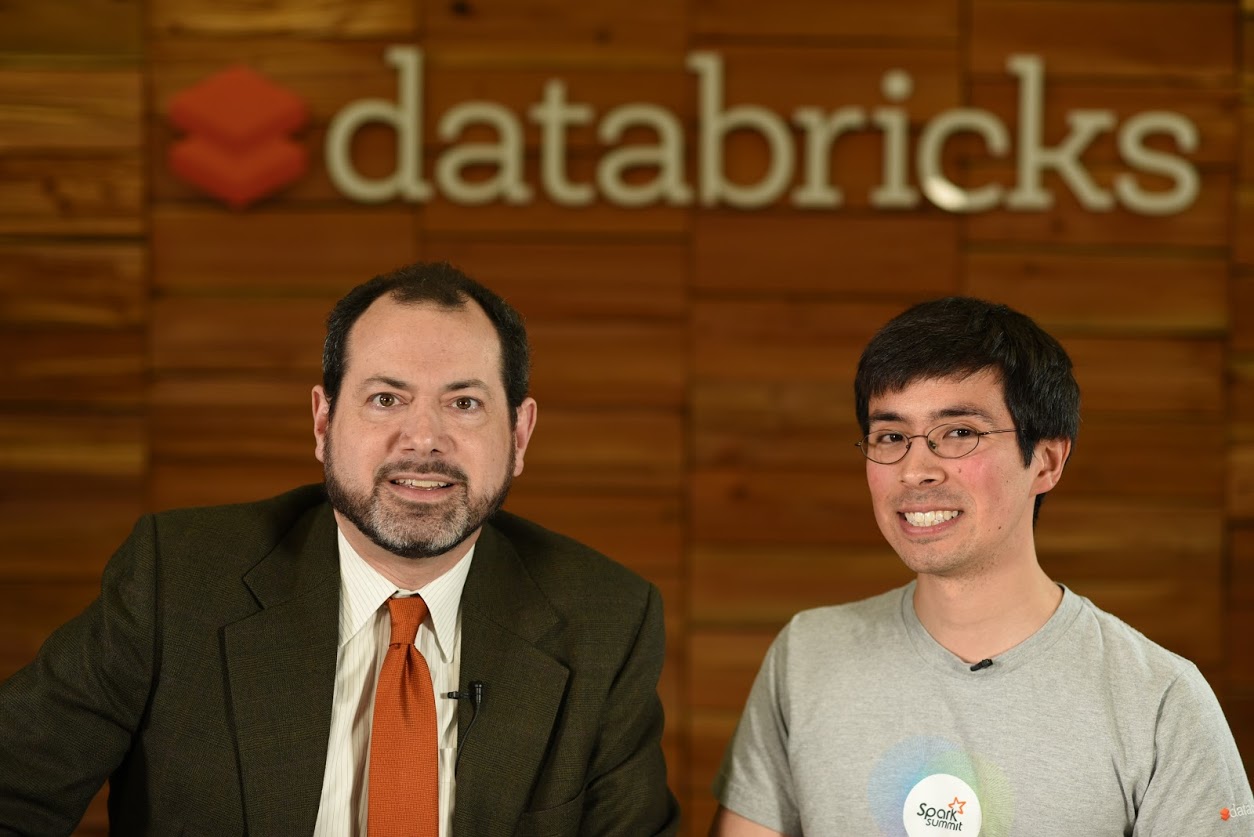
Data is rapidly simplifying, being democratized in part due to the work of open-source platform Apache Spark and its new release, Spark 2.0. Could the minds behind Spark’s data solutions make machine learning tasks just as manageable and intuitive for business environments?
Joseph Bradley, Databricks, Inc. software engineer, said that Spark has a lot going for it in the machine learning field. He told George Gilbert, host of theCUBE, from the SiliconANGLE Media team, its biggest differentiator is scalability.
“Traditional machine learning libraries of course tend to be built often even for a single core from the beginning, whereas with Apache Spark’s library, it was designed for distributed computing,” he said.
Bradley said another asset Spark can offer machine-learning applications is “it is meant to offer the same implementations and APIs and algorithms for multiple languages.” He explained, “I think this really has been one of the big barriers in machine learning.”
Joseph also stated that right now Spark’s Structured Streaming can only apply to batch for learning tasks. Predictions can be made later using Structured Streaming, of course, but Spark 2.0’s touted continuous streaming app capabilities have yet to expand to training machine learning models.
Watch the complete video interviews below, and be sure to check out more of SiliconANGLE and theCUBE’s coverage of Innovation Day at Databricks.
THANK YOU