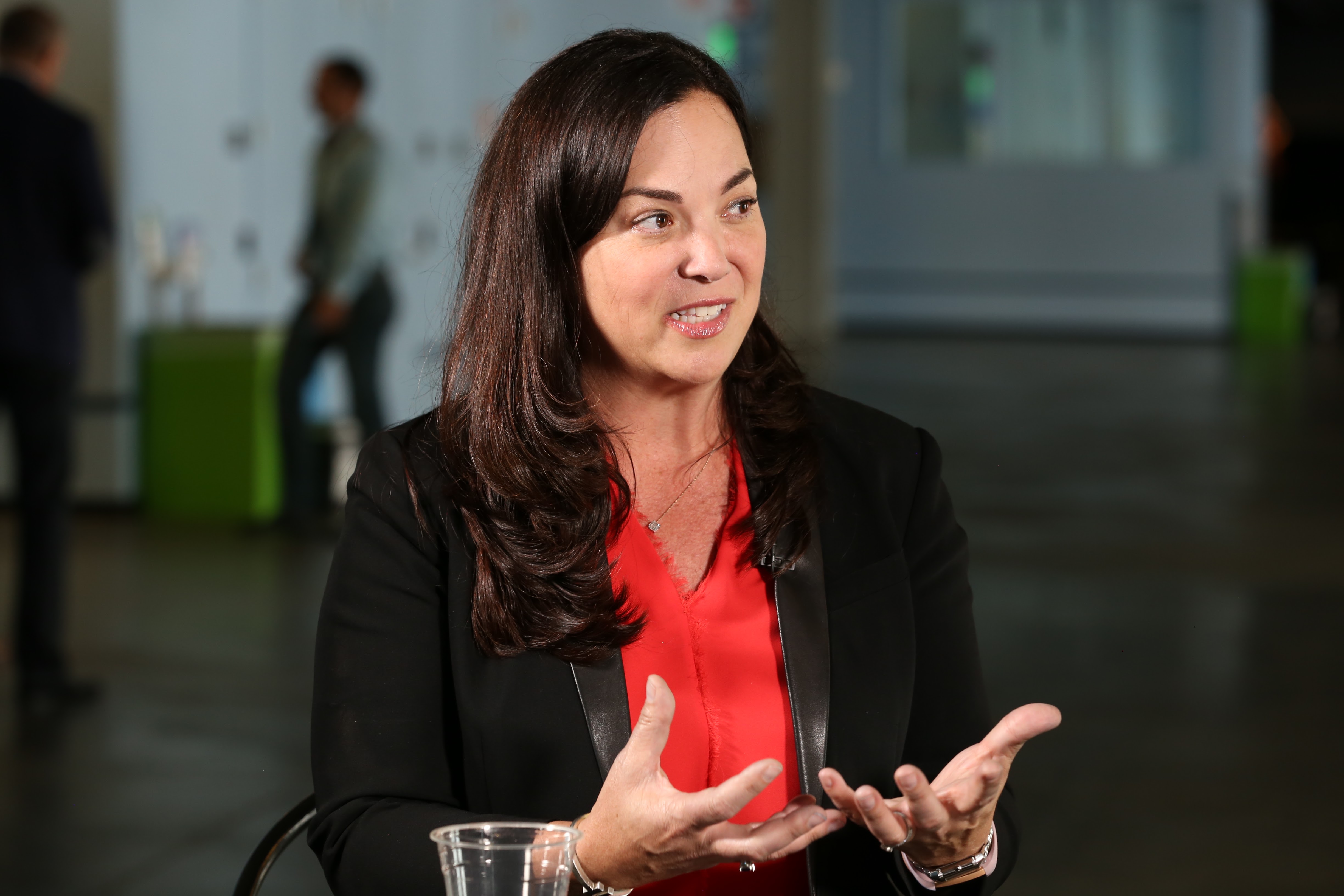
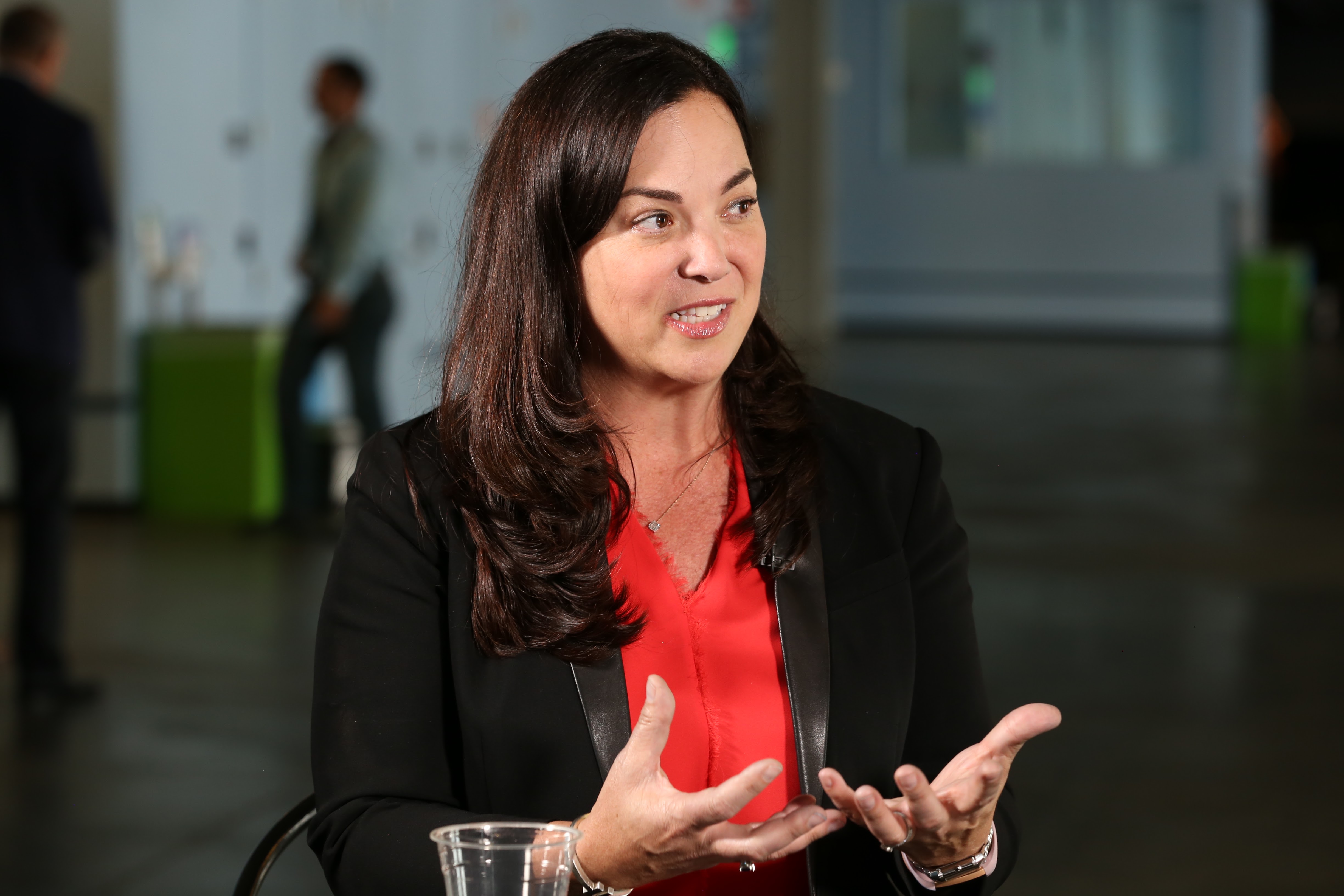
As internally generated data becomes increasingly abundant, companies look at ways to improve internal organizational structure and operations. Jennifer Tejada (pictured), chief executive officer of PagerDuty Inc., a digital operations management platform for businesses, explained how her young company is able to leverage data inputs from their customer base of roughly 10,000 companies to generate valuable insights into workflow optimization.
“The biggest opportunity with machine learning for us is that over the last eight years we’ve been collecting a tremendous amount of data. [Artificial intelligence] and machine learning are only as good as the data they sit on top of,” Tejada said.
Tejada spoke with Jeff Frick (@jefffrick), host of theCUBE, SiliconANGLE Media’s mobile livestreaming studio, during the second annual PagerDuty Summit in San Francisco, California. They discussed PagerDuty’s newest functions and toolsets providing workflow insights through the power of AI. (* Disclosure below.)
In addition to the hard data generated by machine instrumentation, ticketing platforms and software applications, PagerDuty also integrates with data sourced from workflows and human resources. These three types of data sets get fed into a machine learning and analytics engine that provides insight into workflow optimization.
“We have information around the workflow, like what works best for most of our customers and what doesn’t work,” Tejada said. Boosted by cognitive computing, PagerDuty is able to determine the “best agile-centric DevOps-related workflow that enables ultimate response, ultimate availability and ultimate resilience for customers,” she added.
Looking beyond log data, PagerDuty enhances DevOps insight at the human level, sussing out the employees that work the hardest and have the subject expertise proven most useful when “things aren’t working the way they should,” Tejada noted.
She also detailed how these insights into workflows can effectively shorten the time between a problem or opportunity presenting itself and an action being taken by the most capable available personnel.
“You bring all of that together and you build a model that starts to learn, which immediately means you automate a lot of manual process. … An example would be, if an incident pops up, we see it in the form of a signal or a set of events and our machine learning will actually recognize that we’ve seen those events before,” said Tejada.
She also discussed a typical scenario from PagerDuty’s insight solutions: “The last time this happened, here’s what the outcome was, here’s what went well and not so well, here’s how you fixed it and here’s the person who was on top of it, here’s the expert you need to call,” she explained.
Watch the complete video interview below, and be sure to check out more of SiliconANGLE’s and theCUBE’s coverage of PagerDuty Summit. (* Disclosure: PagerDuty Inc. sponsored this segment on SiliconANGLE Media’s theCUBE. Neither PagerDuty nor other sponsors have editorial control over content on theCUBE or SiliconANGLE.)
THANK YOU