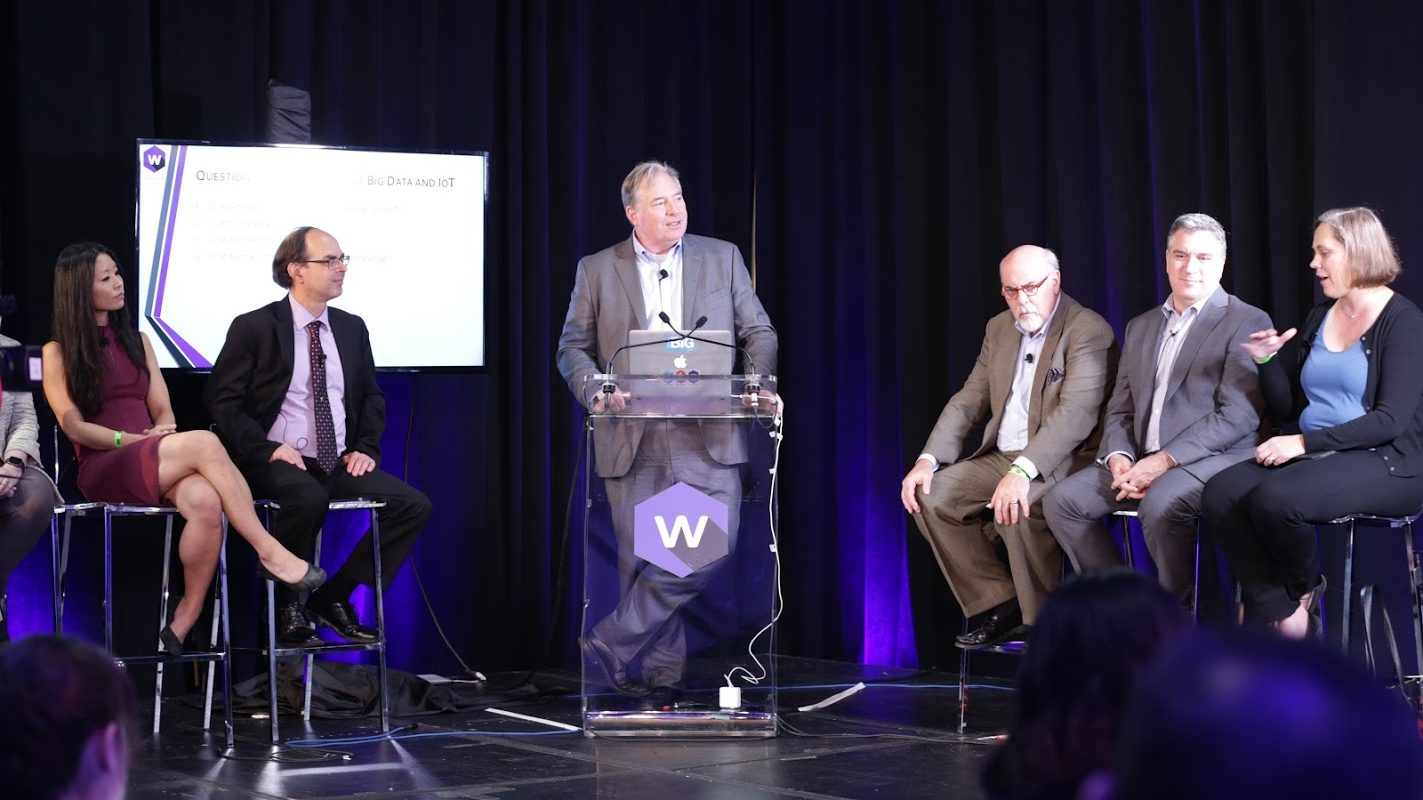
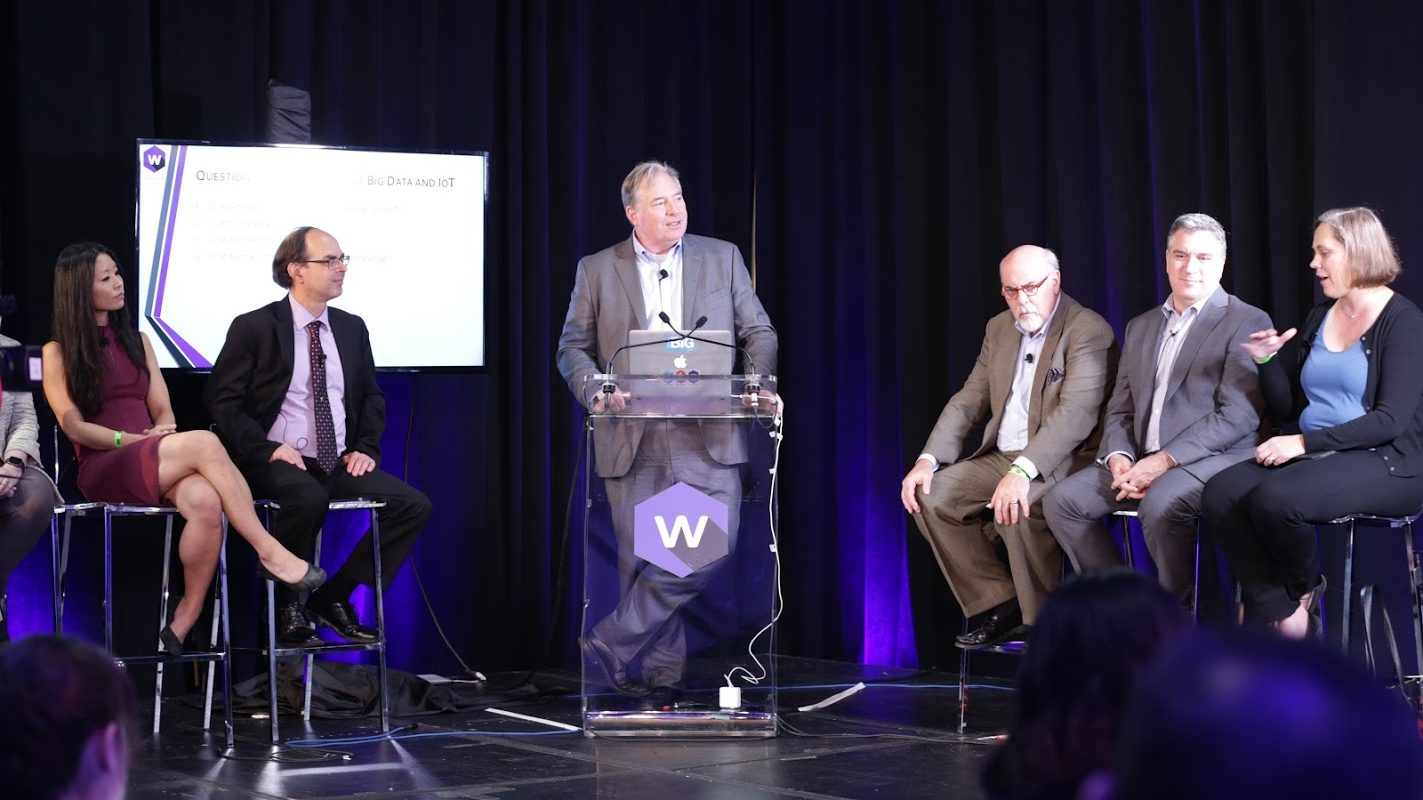
Data is what separates business disruptors from the disrupted in the Digital Age. It has made millionaires and billionaires already, and the game has just teed up. More data means heftier profits — but only if it’s refined and delivered to end users on time and piping hot.
Artificial intelligence can speed up data’s ingestion-to-insight cycle, but training AI models is super compute-heavy — generally too heavy for “internet of things” edge devices, where data is increasingly taking up residence. How will digital businesses squeeze the AI camel through the internet of things needle’s eye?
“The difference between Uber and a taxi cab company is data.” With this statement, Peter Burris (@plburris) (pictured, center), head of research at Wikibon Inc. and host of theCUBE, SiliconANGLE Media’s mobile livestreaming studio, set the tone for Wikibon’s Presentation and Panel discussion during this week’s BigData NYC conference.
Big data is a big subject and, potentially, a big headache. Wikibon researchers have analyzed how cloud infrastructure, AI, deep learning analytics and internet of things must work together to crank profit out of big data. There is no question that businesses, if they wish to survive, will have to develop a data strategy, according to Burris. “We’ve made the observation that the difference between business and digital business, essentially, is one thing — that’s data,” he said. Sitting on the sidelines while competitors develop new ways to cater to consumers with data could prove lethal to today’s businesses.
Whizzing big data around networks — to data lakes or analytics engines and back to end users — is not a piece of cake. Numerous snags — latency, economics, intellectual property control and regulatory compliance — can hinder the data’s movement from the internet of things edge to a central location and back. Cost is perhaps the most prohibitive of these. Wikibon analyst and Chief Technology Officer David Floyer has researched the cost of a hybrid internet of things model versus one that streams all data back to a central location. Floyer’s analysis shows that, over a three-year period, a hybrid model, which keeps more data at the edge, can save 85 percent compared to one that sends it all to cloud. In light of this, Floyer predicted that within a decade, 99 percent of internet of things data will live and die at the edge.
“A strategy to move all internet of things data to the ‘cloud’ will be long-term economic suicide for most enterprises,” Floyer wrote in his analysis.
This is why Wikibon believes true private cloud and architectures that move cloud to data rather than vice versa will grow in coming years.
How does one move cloud — and big data analytics and AI — to the edge? Wikibon invited a number of data experts and practitioners to the panel portion of the Wikibon presentation to pick apart this question.
“The devices at the edge are not very powerful, and they don’t have a lot of memory,” said Neil Raden (@NeilRaden) (third from right), contributing research analyst at Wikibon. This means that the machine learning models that turn data into insight cannot be trained at the edge. “And that’s OK,” Raden said. “Machine learning algorithm development is actually slow and painful.” It required “gobs of data,” skilled data scientists and sophisticated testing, all of which is much better suited to some central location, be it the cloud or an on-premises data center.
Internet of things devices aren’t that dumb, however. Once AI models are ready for prime time, their makers can push them back out to edge devices. “A fair amount of the more narrowly scoped inferences that drive real-time decision support at the point of action will be done on the device itself,” said Wikibon analyst James Kobielus (@jameskobielus) (third from left). In a sense, the internet of things edge is taking us back in time to when hardware, not software, grabbed all the headlines. This edge hardware is getting quite sophisticated; drones are in fact an edge unto themselves, Kobielus stated.
The processing chips inside edge devices have inferring abilities that can aid machine learning models. We are seeing a growing range of hardware architecture capable of ever more complex inference and automation at the edge. In addition to new central processing units, graphics processing units “are in many ways the core hardware substrate for inference engines in DL [deep learning] so far,” Kobielus said. Their price, however, will have to come down before widespread adoption in internet of things devices is possible, he added.
Field-programmable gate arrays, application-specific integrated circuits and neurosynaptic chips, like IBM True North, are also packing big compute brains into small packages. Internet of things developers are arranging these chips in “various combinations that are automating more and more very complex inferences scenarios at the edge,” Kobielus said.
“It opens up a whole new world for engineers to actually look at data and to actually combine both that hardware side as well as the data that’s being collected from it,” said Jennifer Shin (second from left), founder of 8 Path Solutions LLC.
Can huge data lakes and deep learning algorithms in cloud and smart internet of things edge chips deliver bona fide AI? The term has bounced around for decades without much to ground it in reality. “We got clobbered, because we didn’t have the facilities, we didn’t have the resources, to really do AI,” Raden said, referring to techies who dipped their toes in AI in the 1980s. The technology has leaped forward since then, but is it perfect?
The good news is that internet of things sensor data is the purest data the industry has ever had to feed AI models. “Everything that we’ve ever done in analytics has involved pulling data from some other system that was not designed for analytics. But if you think about sensor data, this is data that we’re actually going to catch for the first time,” Raden said.
However, analytic models — even fairly good ones — can botch good data. “You can have a great correlation that’s garbage if you don’t have the right context,” said Judith Hurwitz (far left), president and chief executive officer at Hurwitz & Associates LLC.
Analytics models are developed and trained by both data and humans in a multitude of ways. “When we talk about the edge, we’re talking about human beings and the role that human beings are going to play both as sensors, or carrying things with them, but also as actuators — actually taking action — which is not a simple thing,” Burris said.
Humans may dumb down big data intelligence through bias or staunch rules around data governance. “The biggest problem or challenge with healthcare is no matter how great of a technology you have, you can’t manage what you can’t measure,” said Joe Caserta (second from right), president of Caserta Concepts LLC. “You’re really not allowed to use a lot of this data, so you can’t measure it.”
Sometimes people simply won’t use artificial intelligence because of that whole artificial part. When Stephanie McReynolds (far right), vice president of marketing at Alation Inc., worked in customer relations management, recommendation engines were all the rage.
“The hardest thing was to get a call center agent to actually read the script that the algorithm was presenting to them,” she said. “The most successful companies that are working with AI are actually incorporating it into solutions. So the best AI solutions are actually the products that you don’t know there’s AI underneath.”
Watch the complete presentation and panel video below, and be sure to check out more of SiliconANGLE’s and theCUBE’s coverage of BigData NYC 2017.
THANK YOU