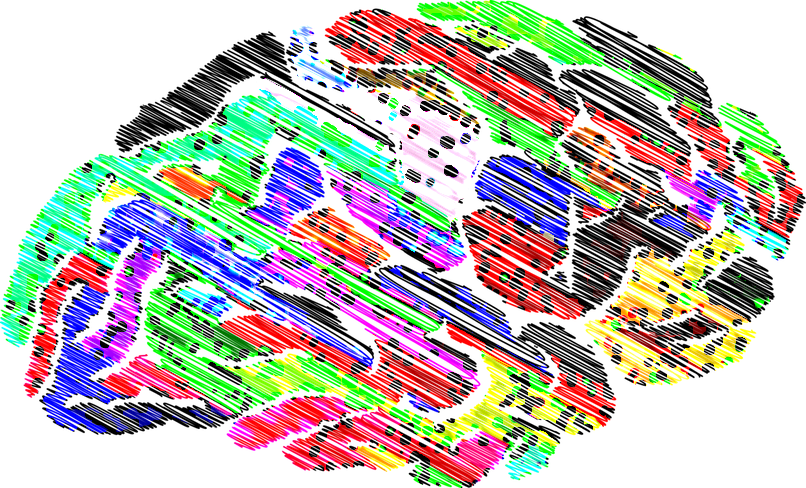
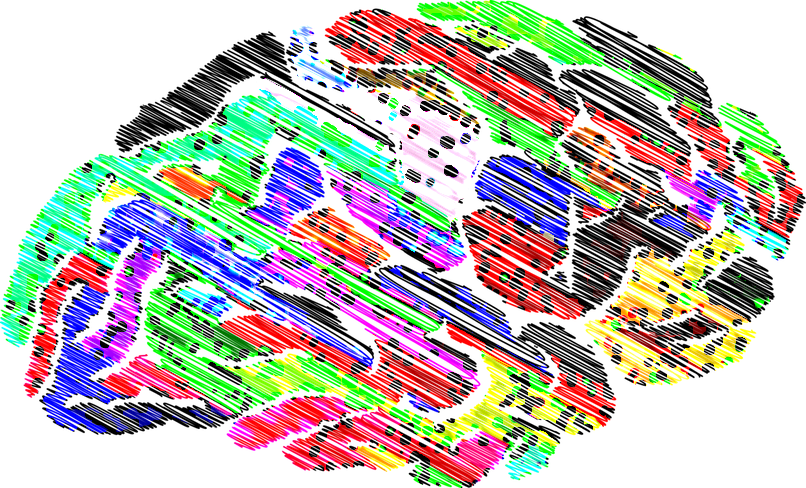
The basic framework for artificial intelligence has existed since the 1940s, and organizations have been innovating atop AI advancements ever since. In recent years, big data and advanced deep learning models have pushed AI into the spotlight like never before. Will these new technological ingredients finally produce the intelligent machines envisaged in science fiction, or are current AI trends just the same wine in a fancier bottle?
“It’s actually new wine, but there’s various bottles and you have different vintages,” said James Kobielus (@jameskobielus, below, left), Wikibon.com’s lead analyst for data science, deep learning and application development.
Actually, much of the old wine is still quite palatable; the new iterations of AI use and build upon methods that have come before, Kobielus added. The technology in Apache’s big data framework Hadoop comes to mind, for example.
Today’s mania around AI, however, is due to certain developments that erstwhile AI hopefuls lacked. They bring us that much closer to machines that seem to actually “think” like humans, according to Kobielus. The most important of these is big data, he said in a conversation at theCUBE’s studio in Marlborough, Massachusetts.
Why has big data sparked renewed interest in AI? It’s a massive help in training deep learning models, which can make more human-like inferences. Kobielus broke down the state of the art in AI and machine intelligence with Dave Vellante (@dvellante, below, right), Wikibon chief analyst and co-host of theCUBE, SiliconANGLE Media’s livestreaming studio.
The pace at which AI is taking over technology conversations mirrors its meteoric revenue growth. The market for AI software was $1.4 billion in 2016 and will rocket to $59.8 billion by 2025, according to research from Tractica LLC.
“Artificial intelligence has applications and use cases in almost every industry vertical and is considered the next big technological shift, similar to past shifts like the industrial revolution, the computer age and the smartphone revolution,” said Tractica Research Director Aditya Kaul. A few of those verticals include finance, advertising, healthcare, aerospace and consumer sectors.
AI software as the next industrial revolution may come across like the fantasy of an over-imaginative nerd. But the sentiment is spreading even outside of Silicon Valley. Time magazine recently devoted an entire special edition to the subject, titled “Artificial Intelligence: The Future of Humankind.”
But this vision of AI has been floated for decades in science fiction and the fever swamps of techland. Has the technology evolved so drastically in just the past several years? What can we realistically expect from the AI of today and the foreseeable future?
First, artificial intelligence is a broad label — actually more of a buzz phrase than a precise technical term. AI refers to “any approach that helps machines to think like humans, essentially,” Kobielus said. But isn’t thinking in the strictest sense distinct to organic human brains? Machines can’t really think, can they? It depends. If one synonym for think is infer, then machines might be said to have parity with brains.
When people discuss AI today, they are usually talking about AI’s most popular approach — machine learning. This is the application of mathematics to infer patterns from data sets.
“Inferring patterns from data has been done for a long time with software,” Kobielus said. Some established inference methods include support vector machines, Bayesian logic and decision trees. These have not gone away and remain in use in the growing universe of AI approaches.
A machine learning model or algorithm trained on data makes its own inferences — often called the outputs or insights of AI. An inference does not have to be pre-programmed into a machine, only the model itself.
In a way quite analogous to the process of human comprehension, machine learning models infer based on statistical likelihood. Such inferences from data can come in the form of predictions, correlations, categorization, classification, anomaly or trend recognition, etc.
For machines, learning happens in layers. By layering data classifiers, called perceptrons, an artificial neural network is formed. This neural relationship between perceptrons activates their functions, including nonlinear ones such as tangents. Through this neural process, the answer or output of one layer becomes the input for the next. At the final layer, intelligence surfaces.
Deep learning networks are artificial neural networks with a high number of perceptron layers. The more layers a network has, the deeper it is. The extra layers ask more questions, process more inputs, and produce more outputs to abstract higher-level phenomena from data.
Facebook’s automated face recognition technology is powered by deep learning networks. Combining more layers could give a richer description of an image. “It’s not just, is this a face? But if it’s a scene-recognition deep learning network, it might recognize this is a face that corresponds to a person named Dave who also happens to be the father in the particular family scene,” Kobielus said.
There are now neural networks with 1,000 of perceptron layers, and software developers are still discovering what deeper neural networks can accomplish.
The face-detection software in the latest Apple iPhones rely on convolutional neural networks with 20-some layers. And in 2015, Microsoft Corp. researchers won the ImageNet computer vision competition with a 152-layer deep residual network.
Thanks to a design that prevents data dilution from layer to layer, the network can glean more information from images than typical 20- or 30-layer varieties, according to Peter Lee, head of research at Microsoft. “There is a lot more subtlety that can be learned,” he said.
Aside from image processing, novel AI and deep learning use cases are popping up in areas from law enforcement to genomics. In a study from last year, researchers used AI to predict the verdict in hundreds of cases in the European Court of Human Rights. They predicted human judges’ decisions correctly in 79 percent of cases.
With the ability to “think” at warp speed and with an abundance of resources, there’s even cases of machines reaching more accurate conclusions than people. Stanford University researchers’ deep learning algorithm recently proved better at diagnosing pneumonia than human radiologists. The algorithm called CheXNet uses a 121-layer convolutional neural network trained on a set of over 100,000 chest X-ray images.
This underlines a crucial point about deep learning: The algorithms are as good as the data they train on. Their predictions are basically accurate in proportion to the size of the datasets that inform them. And this training process requires expert supervision.
“You need teams of data scientists and other developers who are adept at statistical modeling, who are adept at acquiring the training data, at labeling it (labeling is an important function there), and who are adept at basically developing and deploying one model after another in an iterative fashion through [developer operations],” he said.
Labeling data for machine learning models is indeed crucial, and human eyes are still the best tools for the job. IBM Corp. said last year that it was already hiring lots of people just to label data for AI uses.
University of Toronto researchers Parham Aarabi and Wenzhi Guo demonstrated how well human brains and neural nets go together. They developed an algorithm that learns from explicit human instructions, instead of a set of examples. In image recognition, trainers may tell the algorithm that skies are usually blue and located at the top of a picture. Their method worked 16o percent better than conventional training of neural networks.
“Without training the algorithms, you don’t know if the algorithm’s effective for its intended purpose,” Kobielus said.
Much training will take place in a cloud or other centralized environments, while dispersed “internet of things” devices (think autonomous vehicles) will make the on-the-spot decisions, Kobielus concluded.
Watch the complete interview below:
THANK YOU