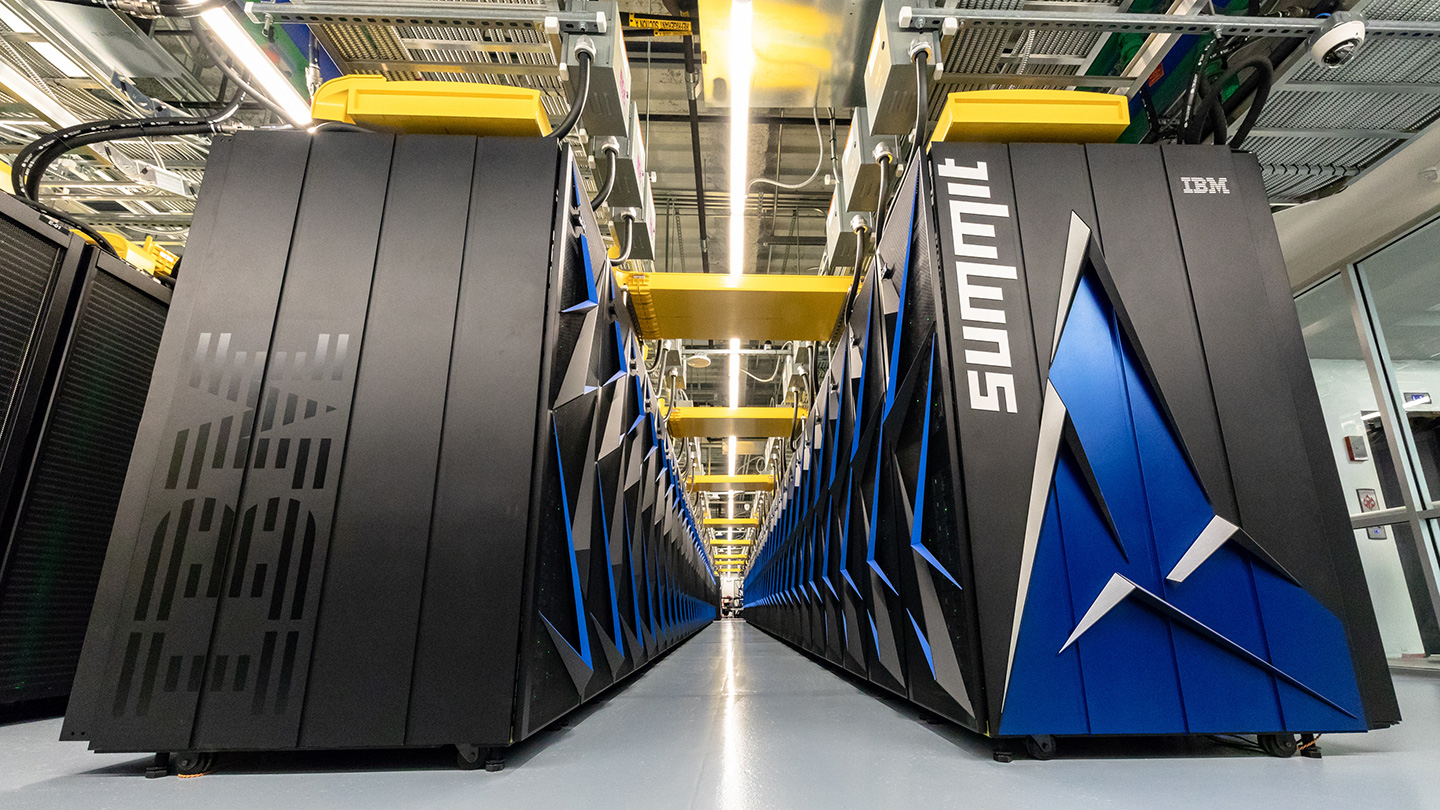
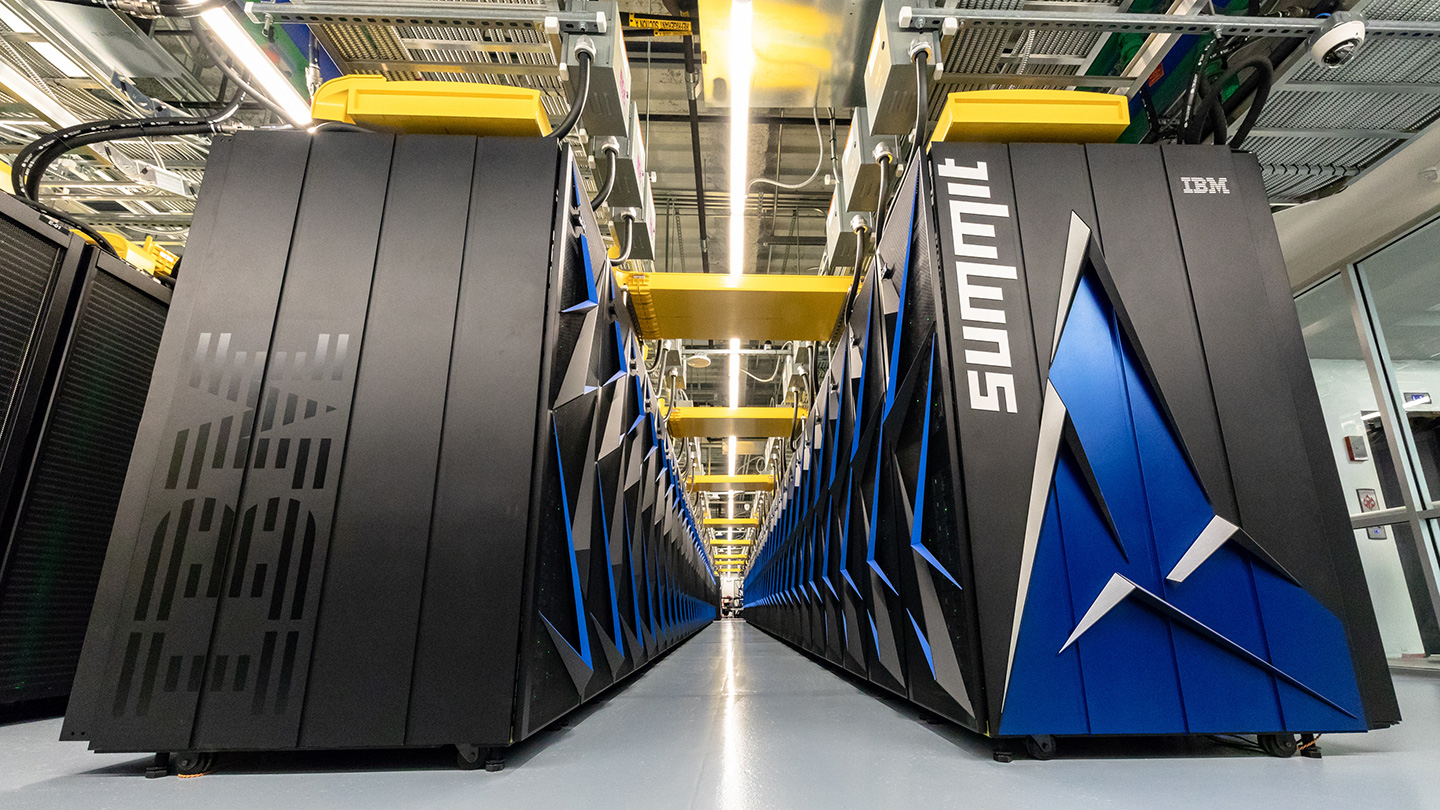
There’s a new No. 1 supercomputer in the world, as the United States has captured the lead from China for the first time in more than five years.
The Summit supercomputer (pictured), built by IBM Corp. at the Oak Ridge National Laboratory in Tennessee, captured the top spot in the twice-a-year list released early Monday by the TOP500 project, which anoints the 500 most powerful nondistributed computers in the world. The news was announced at the annual International Supercomputer Conference opening this week in Frankfurt, Germany.
Notable besides the competition between the U.S. and China is the outsized presence of graphics processing units from Nvidia Corp. that were created originally to speed up graphics for video games. Now, five of the seven fastest supercomputers are using Nvidia’s Tensor Core GPUs, including the Summit.
The massive use of GPUs in what are viewed as the most powerful computers in the world is a stark sign of their rise as go-to commercial chips for both high-performance computing and artificial intelligence, thanks to their ability to process huge amounts of data in parallel. Their rate of improvement is making up for the slowdown in Moore’s Law, the truism that chip density has doubled every couple of years for decades. But recently the rate has noticeably slowed down, probably for good as the limits of traditional processors and manufacturing processes become more apparent.
That said, Intel Corp.’s mainstream Xeon processors still anchor nearly all the supercomputers on the list, powering 95 percent of the top 500 and 97 percent of the new systems on the list.
Still, the GPU’s rise is obvious in the share of computing power the chips claim in these supercomputers. Nvidia said its share of new floating-point operations per second, a standard unit of computing power, on the top 500 systems: It’s now 56 percent with Nvidia’s latest Tesla V100, up from 25 percent with its previous-generation Tesla P100 last year and 11 percent with its Tesla K80 in 2015. With the Summit supercomputer, GPUs account for a full 95 percent of the computing power.
The Summit’s achievement isn’t entirely a surprise, since the TOP500 website earlier this month had reported that it reached a peak speed of 200 petaflops, a unit of computing speed equal to a quadrillion floating-point operations per second. As it turns out, according to the official TOP500 measure, it clocks in at 120 petaflops.
But that’s enough to beat the previous TOP500 champion and now No. 2, China’s Sunway TaihuLight, which can do 93 petaflops. Coming in third was the Sierra, built by IBM at the Department of Energy’s Lawrence Livermore National Laboratory.
The Summit uses an almost unimaginable 27,648 Tensor Core GPUs, which are especially useful for AI, machine learning and deep learning neural networks, in addition to 9,216 IBM processors. GPUs also power 17 of the top 500 supercomputers, including the Sierra and the fastest supercomputers in Japan and Europe.
Supercomputers are used for the world’s most demanding problems, from predicting weather to analyzing materials that might make better superconductors to gauging opioid sensitivity based on various genes.
“This is a very exciting time for high-performance computing,” Ian Buck, Nvidia’s vice president and general manager of accelerated computing, said on a press call Friday. “These new AI supercomputers will redefine the future of computing.”
Despite the U.S. taking the top spot, it’s still losing ground overall, at least in the number of systems in the top 500. It claims only 124 systems on the list, which the TOP500 said was a new low, down from 145 just six months ago. At the same time, China improved its overall position to 206, up slightly from 202 on the last list.
Nvidia also announced that it has tripled the number of software containers available in its GPU Cloud service, to 35, since they launched last year. Containers are a method of packaging up applications so they can run on many kinds of computers and operating systems in private data centers and in the cloud. The containers make working with deep learning frameworks such as TensorFlow for designing and training neural networks faster and easier, Nvidia said.
THANK YOU