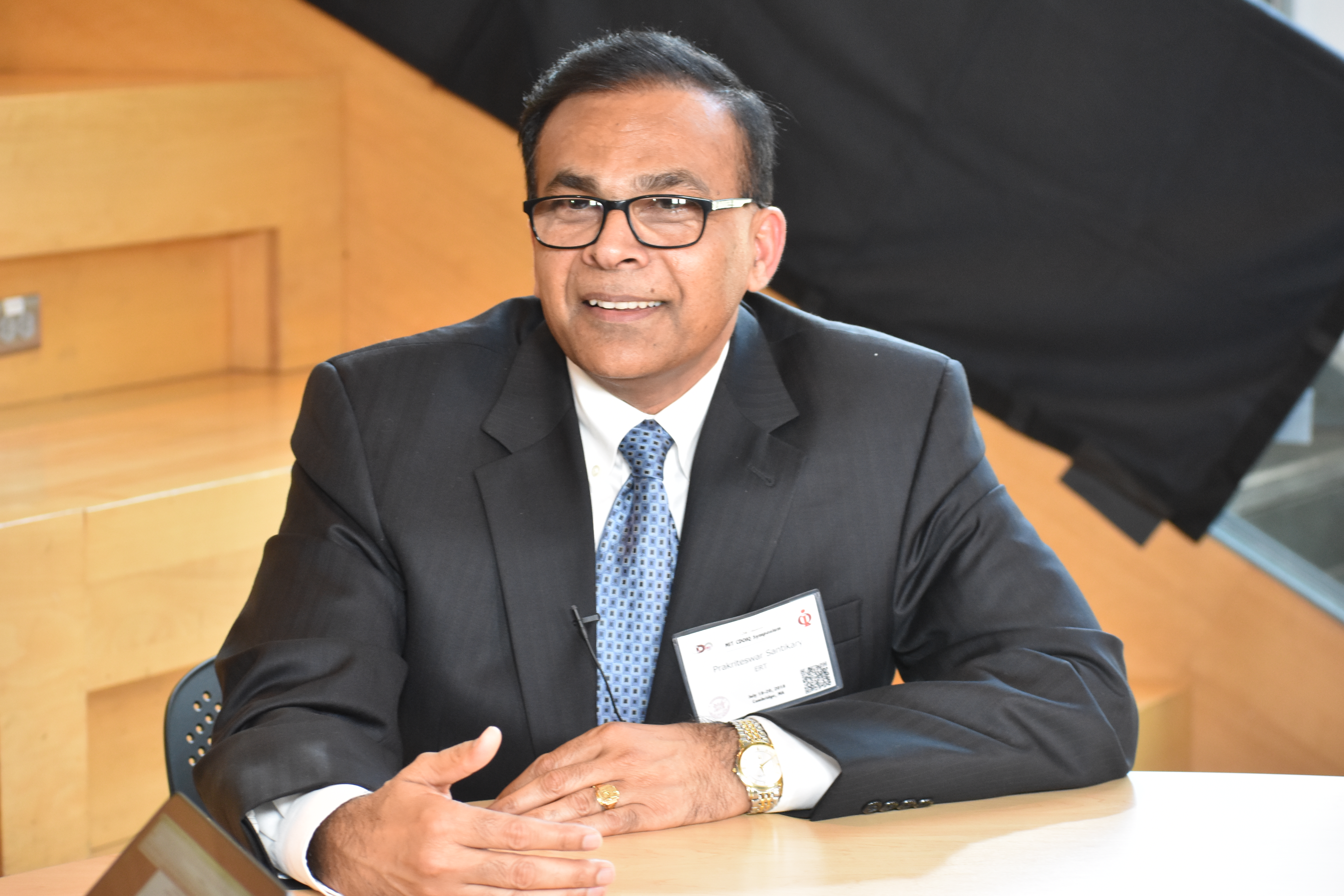
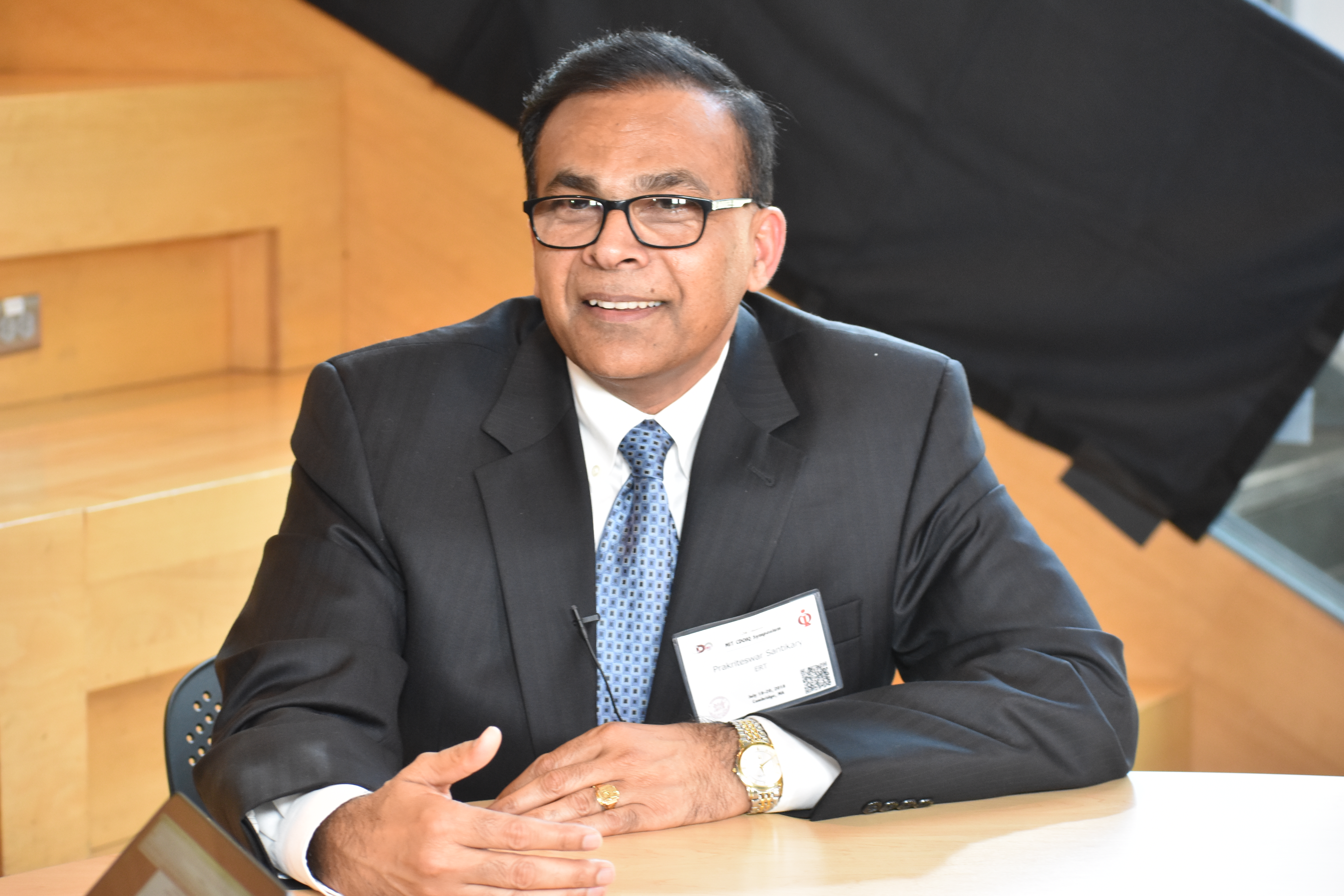
Anybody who’s looked at their medical bills lately knows something deep in the machinery of the healthcare industry is broken. The U.S. has some of the highest drug prices in the world — high enough to prompt President Trump to declare that drug companies are “getting away with murder.” Silicon Valley is exporting cost-cutting solutions to industries far and wide, so what about healthcare? Can someone plug the helium valve inflating prices with all the data analytics and artificial intelligence they keep banging on about?
It takes 10 to 15 years and $2.5 to $3 billion to develop and commercialize the typical pharmaceutical drug, according to Prakriteswar Santikary (pictured), vice president and chief data officer at eResearch Technology Inc. (ERT). The clinical trials that inform drug development are becoming more complex, scaling out globally and requiring larger expenditures, he explained.
“That cost comes down to the consumers — that means patients. So the cost of the healthcare is growing, skyrocketing,” Santikary said. The drug industry is ripe for disruption. Data efficiency and analytics technology could reform many steps on the path to Food and Drug Administration approval. The new set of methods and technologies could more than halve start-to-finish trial times, he added.
Santikary spoke with Rebecca Knight (@knightrm) and Peter Burris (@plburris), co-hosts of theCUBE, SiliconANGLE Media’s mobile livestreaming studio, during the MIT CDOIQ Symposium in Cambridge, Massachusetts. They discussed how technology will reduce inefficiencies and cut wasteful spending in drug development.
Watch Part 1 of theCUBE’s interview with Prakriteswar Santikary below:
Clinical trials have historically been complicated and drawn-out endeavors. Selecting suitable sites and subjects, retaining the subjects, and adhering to various regulatory guidelines can be challenging for trial conductors, according to Santikary. And information may come to light at the eleventh hour that casts doubt on previously workable hypotheses. Technology can cut a lot of fat out of the trial process by basically decentralizing it.
“Instead of patients coming to the clinical trial, the clinical trial is going to the patient,” Santikary stated.
Patients don’t need to report to the site in order for researchers to monitor them anymore. There are now FDA-regulated wearable devices that can collect the needed information on study participants. Instead of organizing huge clinical trials, drug companies can set up a number of micro trials and aggregate all of their data together.
“It still needs to be aggregated, but you can get the early results quicker so that you can decide whether you need to keep investing in the trial or not — instead of waiting 10 years only to find out that your trial is going to fail,” Santikary said.
A smaller trial size also helps home in on how illness effects narrowly defined groups of individuals. It can magnify the particular symptoms and drug responses of sufferers. “You don’t run a trial on breast cancer anymore; you just say breast cancer for this patient,” Santikary said.
Some healthcare organizations — the American Heart Association, for example — have built initiatives around patient data. The AHA’s Precision medicine platform drills deep into individuals’ data to help treat and prevent heart disease. Combining data on a patient’s genes, environment and lifestyle provide a clear picture of his or her health.
“That then results in prevention and treatment that’s catered to you as an individual rather than a one-size-fits-all approach,” Laura Stevens, AHA data scientist, recently told theCUBE.
Researchers expect the global market for precision medicine to reach $88.64 billion by 2022.
Watch Part 2 of theCUBE’s interview with Prakriteswar Santikary below:
Wise handling and analysis of data can also come in handy in the planning and execution of clinical trials. More and more real-world data-based evidence is going into early-stage design of trial protocols, Santikary said. Early-stage and continuous data analysis can warn researchers of gaps or inconsistencies before they’ve thrown away lots of time. “At the end of the day, data quality is vital for the approval of the drug,” Santikary said.
Artificial intelligence and machine learning tools can plow through tons of structured and unstructured data — prescription data, claims data, evidence-based data from real patients, etc. The insights AI and ML render can help researchers design the study, find participants and so forth. “Instead of spending one year to recruit patients, you use AI techniques to get to the right patients in minutes,” Santikary said.
In June ERT added an integrated data platform to its portfolio of technologies geared specifically for clinical trial support. Built on the Amazon Web Services Inc. cloud, the platform ingests and integrates disparate data types. It features AI-enabled services for data governance, risk monitoring, real-time analytics and business intelligence.
Taken together, these technologies can take a massive bite out of trial duration, Santikary said. “When you use these kind of AI techniques and real-world evidence data and all this, the projection is that it will reduce the cycle by 60 to 70 percent — the whole study begin to end time.”
Be sure to check out more of SiliconANGLE’s and theCUBE’s coverage of the MIT CDOIQ Symposium.
THANK YOU