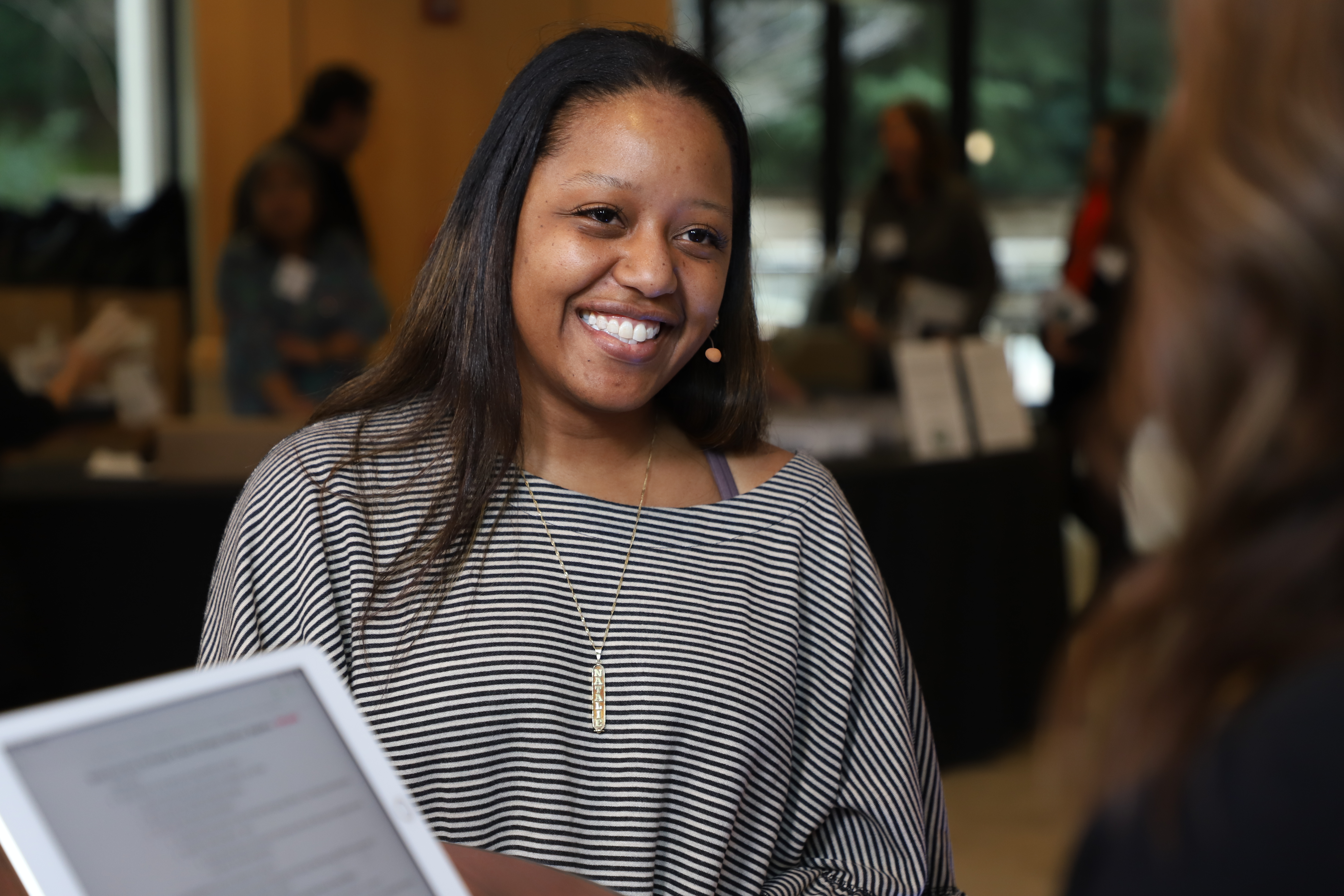
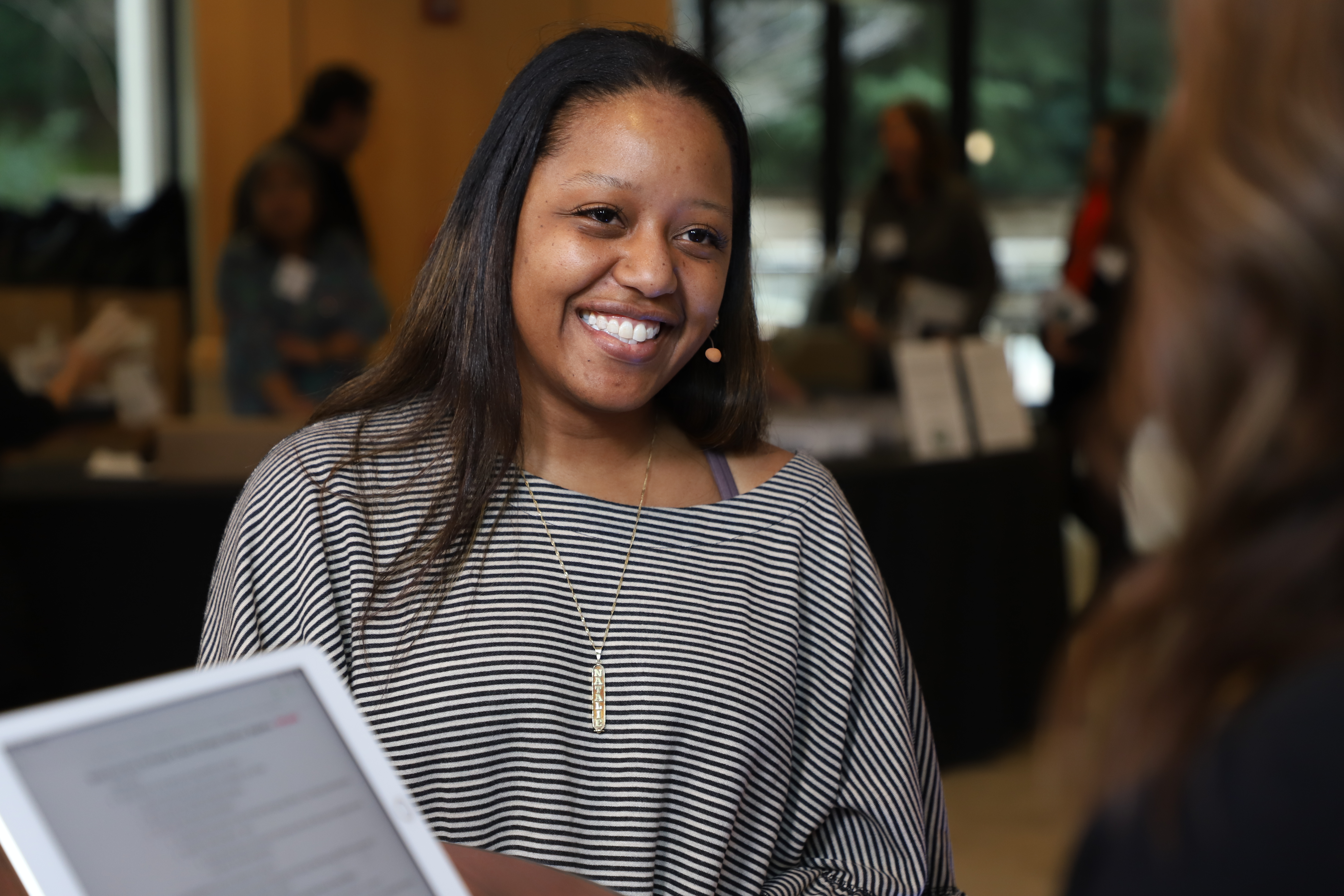
Two developments have ushered in this golden age of data analytics. One is the gargantuan, always-expanding volume of data available. The other is advanced new machine-learning and artificial-intelligence technology.
One might think these two dogs could race to the finish by themselves. There is, however, another element needed in data science today. Without it, skewed inputs and outputs and general bias could mar the best analytics efforts.
“People is what the greatest challenge to data science is going to be in the future,” said Natalie Evans Harris (pictured), co-founder and head of strategic initiatives at BrightHive Inc. Consumers of analytics software might easily forget that the algorithms didn’t make themselves; people made them. What’s inside those people’s heads will likely find its way into the algorithms. Anyone who might trust them to make decisions ought to be concerned about that.
Advanced new AI and ML software has lowered the barrier to entry for businesses, government agencies and others. It’s never been easier for them to apply data science to their particular set of problems. “But we also need to recognize that no matter how good AI gets, there’s still humans that need to be a part of that context, because the algorithms are only as strong as the people that have developed them,” Evans Harris said.
How can we make sure we develop strong, relatively unbiased algorithms? By putting a variety of people with a diverse range of experience and education to the task, according to Evans Harris.
Evans Harris spoke with Lisa Martin, host of theCUBE, SiliconANGLE Media’s mobile livestreaming studio, during the Stanford Women in Data Science event at Stanford University. They discussed data science’s people problems and the behaviors that can help limit bias in algorithms.
This week, theCUBE spotlights Natalie Evans Harris and BrightHive in its Startup of the Week feature.
Accurate data analysis — the kind that answers questions or predicts outcomes — is no mean feat. The data that an algorithm trains on will ultimately inform the algorithm. Selecting the proper data to analyze from the enormous amount available can be a challenge.
“If you have really large data sets, you might not even realize that the data are slightly biased on gender or whatever you’re analyzing,” Jeff Welser, a vice president and lab director at IBM Corp.’s Research Almaden Research Center in San Jose, told SiliconANGLE in December. “It might be that you’ve overtrained on those characteristics.”
Say an algorithm is meant to predict driving behaviors of the general population. What if alcoholics and individuals with an artificial arm are overrepresented in the data set? The algorithm trained on that data probably won’t have the greatest predictive power. Humans are needed to ask questions of data to avoid situations like this. This is a skill that anyone working with data must learn, according to Evans Harris.
The entrepreneur previously worked for the National Security Agency to help build its data science program. There, she discovered how vital skillful question-asking is to data science. It actually became an official class for the data scientists there.
“We made every single one of them take a class on asking questions — the same class that we had our intelligence analysts take,” Evans Harris said. “So the same ways that the history and the foreign language experts needed to learn how to ask questions of data, we needed our data scientists to learn that as well.”
Different types of people will ask different questions of data. Asking more questions from many different angles can result in interesting insights, inspire new analysis projects, and vet data for bias.
Absolutely bias-free AI is probably a fantasy, according to Alexander Linden, research vice president at Gartner Inc. In fact, Gartner predicts that through 2022, 85 percent of AI projects will deliver erroneous outcomes due to bias in data, algorithms, or the teams responsible for managing them.
“Today, there is no way to completely banish bias,” Linden said. “However, we have to try to reduce it to a minimum. In addition to technological solutions, such as diverse datasets, it is also crucial to ensure diversity in the teams working with the AI and have team members review each other’s work. This simple process can significantly reduce selection and confirmation bias.”
Other experts echo Linden’s sentiment.
“Most people think algorithms are objective — but in large part they’re opinions embedded in code that also includes biases,” AI startup adviser Steve Ardire told IBM Big Data and Analytics Hub. “We must develop effective mechanisms in algorithms to filter out biases and build ethics into AI with ability to read between the lines to get closer to common sense reasoning.”
Good data practices transcend accurate algorithms. More and more questions about privacy and ethics in data analytics and AI are popping up. Legislation such as the European Union’s General Data Protection Regulation is pressing companies to be more responsible with customers’ data.
BrightHive helps organizations build something it calls data trusts. A BrightHive data trust allows networks of organizations to securely, responsibly and ethically share data, collaborate and generate new insights.
BrightHive offers both data trust development and management. It enables a network of individuals in a trust to communicate and collaborate about proper uses of data. All members of a data trust participate in the governance and control of data. A learning community helps develop “data capacity” among member organizations. And data trusts have embedded community-developed ethical principles for data use.
“We need to recognize that privacy is more than just virus protection, that there is a trust that needs to be built between the individuals, the communities, and the companies that are using this data,” Evans Harris said. “What the answers are is what we’re still figuring out. I argue that a large part of it is just human capital.”
BrightHive, Bloomberg L.P. and Data for Democracy collaborated on an initiative called “Community Principles on Ethical Data Sharing.” It aims to develop a “Hippocratic Oath” for data scientists.
Here’s the complete video interview, part of SiliconANGLE’s and theCUBE’s coverage of the Stanford Women in Data Science event:
THANK YOU