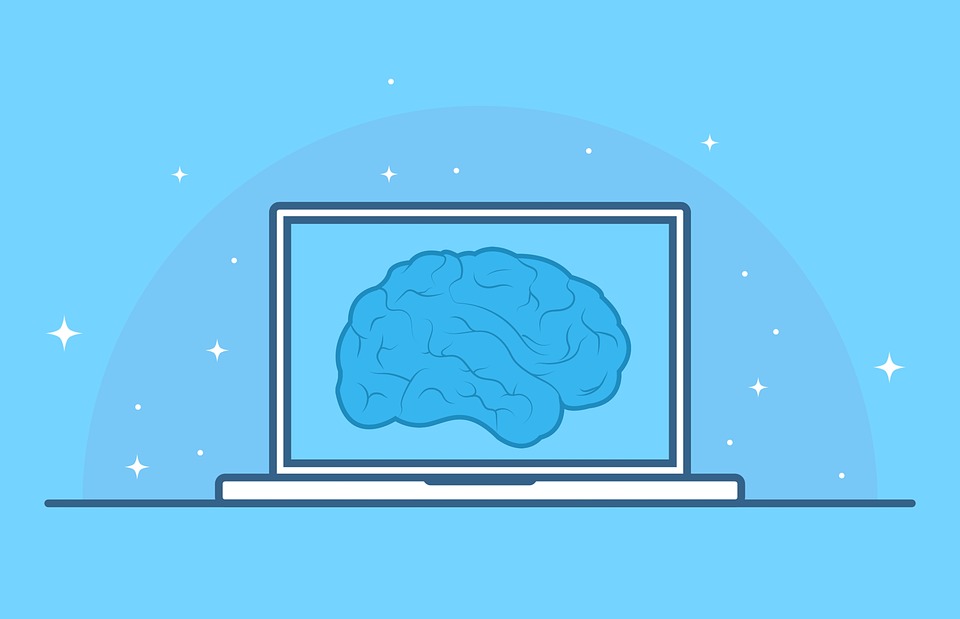
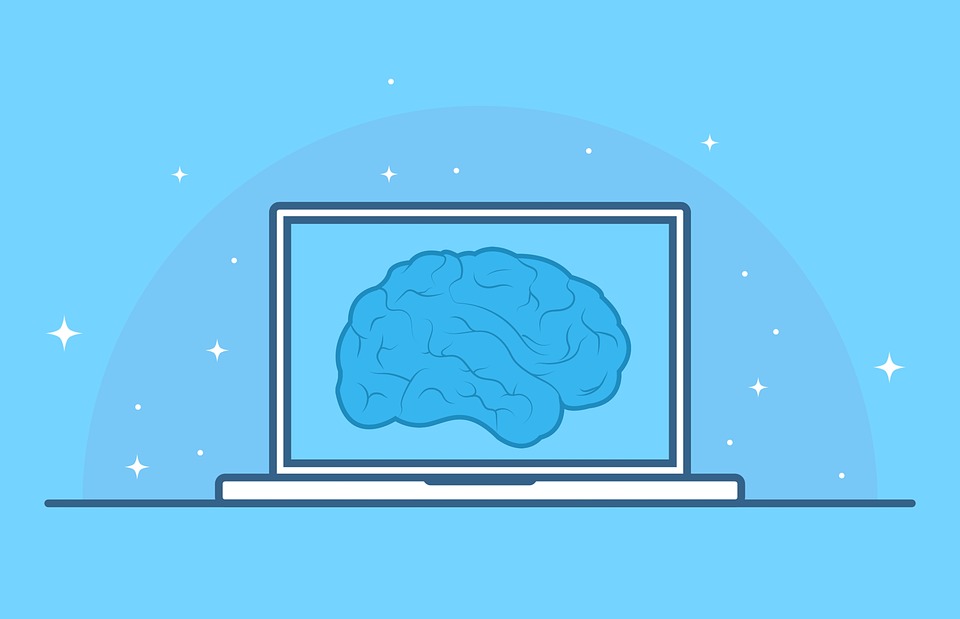
Israeli artificial intelligence startup Run:AI wants to speed up the way we train deep learning models after exiting stealth mode with $13 million in its war chest.
The money comes from a $10 million Series A round of financing announced today. It was led by Haim Sadger’s S Capital and TLV Partners, following an earlier $3 million dose of seed funding.
Run:AI said it has designed a high-performance compute virtualization layer for deep learning that it believes can significantly reduce the time it takes to train neural network models.
That should be useful because neural networks are the basis of deep learning, which is a subset of AI that mimics the way the human brain works and enables technologies such as image recognition, autonomous driving, smart assistants and more.
The issue is that deep learning models need to be thoroughly trained before they can be put to use. But training takes a long time as it requires huge numbers of graphical processing units or specialized AI chips to process the enormous data sets that are used to “teach” the models. The process is therefore very costly. As a result, the training process can often take weeks or months to complete, delaying the introduction of new models.
“Traditional computing uses virtualization to help many users or processes share one physical resource efficiently; virtualization tries to be generous,” explained Omri Geller, Run:AI’s co-founder and chief executive officer. “But a deep learning workload is essentially selfish since it requires the opposite: it needs the full computing power of multiple physical resources for a single workload, without holding anything back. Traditional computing software just can’t satisfy the resource requirements for deep learning workloads.”
To remedy the problem, Run:AI said, it has built an entirely new software stack for deep learning that works by virtualizing different compute resources into a single computer with nodes that are able to work in parallel with one another.
Key to Run:AI’s software stack is its “compute abstraction layer,” which uses graph-based parallel computing algorithms to analyze deep learning models and split them up into smaller parts. Training of different parts of the model can then be run in parallel, thereby accelerating the time it takes to complete the process.
In addition, Run:AI said, its abstraction layer technique helps sidestep the limitations of the underlying hardware’s random-access memory. That makes it possible to run bigger and more accurate models, such as being able to spot finer details in images, without the need to upgrade hardware.
Run:AI is a promising startup because there’s no question that deep learning requires a totally different stack to traditional computing in order to run more efficiently, said Holger Mueller, principal analyst and vice president of Constellation Research Inc.
“There are tremendous opportunities for rethinking the whole technology stack for new use cases,” Mueller said. “It’s now up to Run:AI to prove these conceptual benefits in real-world scenarios.”
THANK YOU