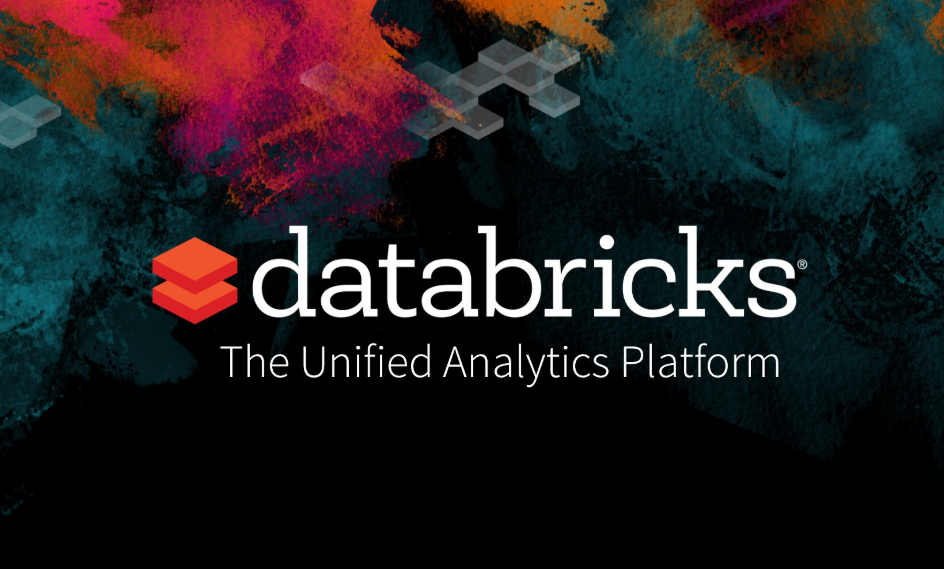
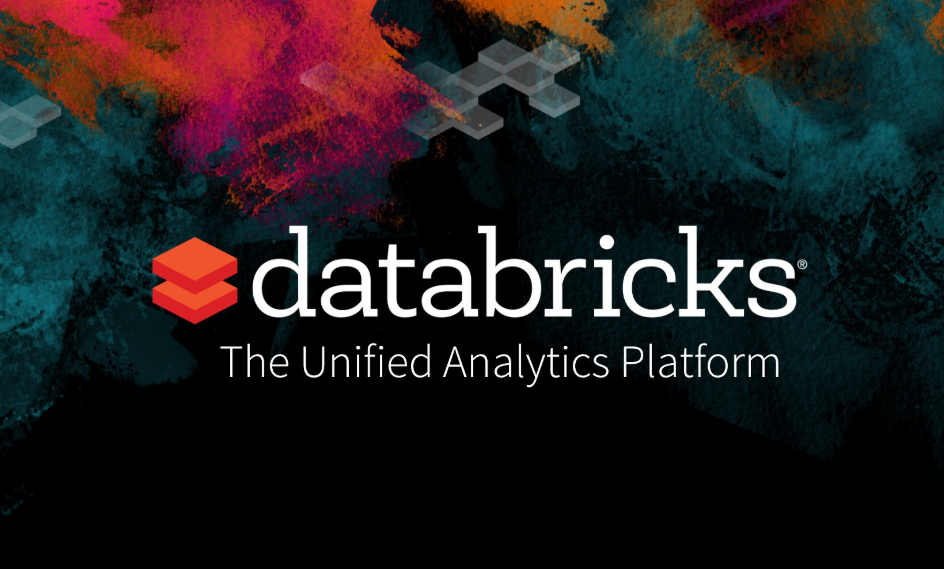
Big-data company Databricks Inc. is hoping to empower so-called citizen data scientists to create their own machine learning models with new “Automated Machine Learning” capabilities in its Unified Analytics platform.
The AutoML capabilities announced today rely on machine learning too, and are designed to help untrained workers muddle their way through the key steps involved in creating and training machine learning models. Machine learning models are mathematical representations of real-world processes that are used to make predictions, and are created by providing training data for an algorithm to learn from.
Creating machine learning models is no easy task, however. It’s normally done by highly trained data scientists and requires extensive preparation of the training data that’s going to be used. Other requirements include feature engineering, hyperparameter tuning, automatic model tracking, reproducibility and deployment. These are the processes that Databricks said it now can automate with its new capabilities.
“By introducing the concept of ‘low-code’ and ‘no-code,’ AutoML represents a fundamental shift in the way organizations approach machine learning and data science,” said Adam Conway, Databricks’ vice president of product management. “With the right automation, AutoML can dramatically shorten time-to-value for data science teams.”
Wikibon analyst James Kobielus told SiliconANGLE he welcomed Databrick’s new AutoML tools because automation is fast becoming the standard approach for enterprises looking to implement machine learning in DevOps.
“There simply aren’t enough expert, experienced and trained data scientists in the world to do all this work manually at the speed and scale required for modern machine learning operations,” Kobielus said. “These latest AutoML announcements address a sweet spot in the marketplace for augmented programming tools to help the next generation of citizen data scientists automate more of the development, training and tuning of ML models.”
Kobielus added that he was particularly impressed with Databricks’ sophisticated tools for model hyperparameter tuning, which he said can make all the difference between a continually well-performing ML model and one that suffers from rapid decay in real-world deployments.
“We hope Databricks will follow these announcements with a strong push to educate the business analysts and subject matter experts of the world in the new arts of AutoML,” he said.
The new capabilities are being integrated with Databricks’ MLflow offering, which is an open-source framework it announced last year that’s used to package machine learning code, execute it and test it, and then deploy it into production across multiple cloud platforms.
MLflow itself draws on the power of the open-source big data processing framework Apache Spark, the key component of Databricks’ Unified Analytics Platform, which is used to analyze data, build data pipelines across siloed storage systems and prepare labeled datasets for model building.
THANK YOU