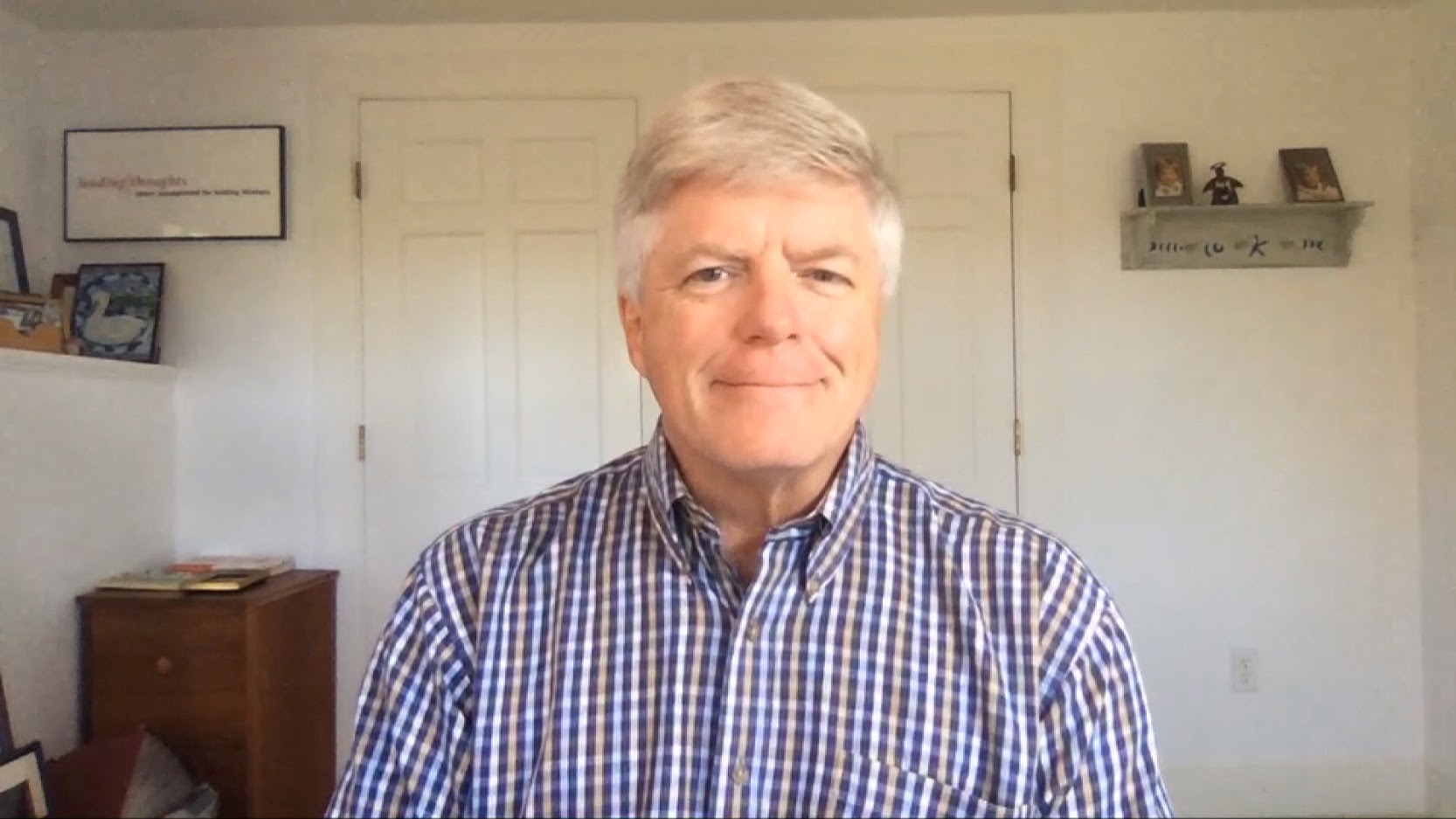
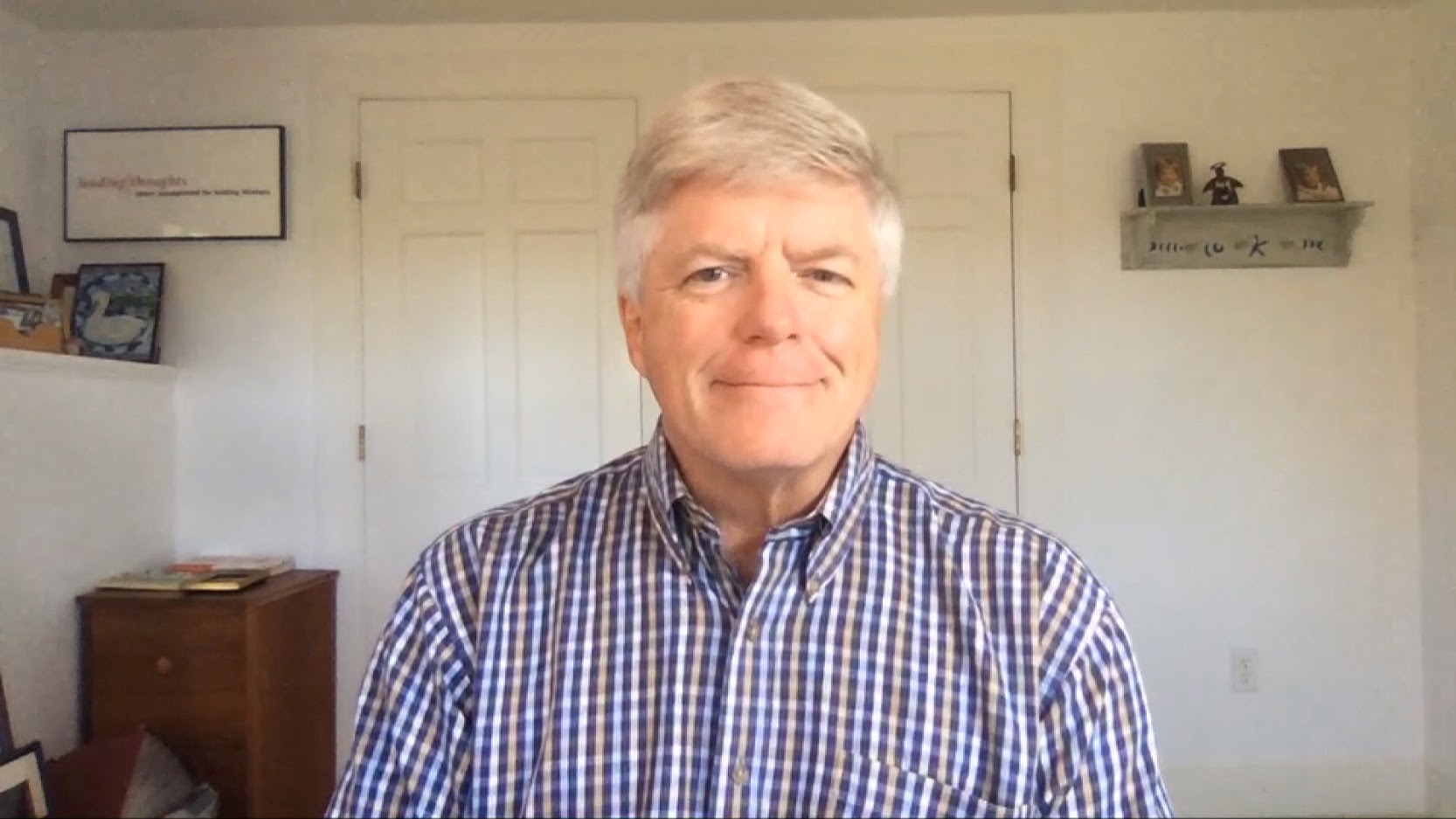
Advancements in artificial intelligence are making machines smarter, so the sharpest and most data-hungry machines can now take on more sophisticated jobs. But AI is still not fully suitable for servant robots, driving vehicles, finding new planets or dealing with pandemics.
The reason: Although AI is evolving quickly, it’s still no match for what’s underneath human capacity. Machines still don’t (and may never) have our gut decision-making abilities to make engaged, conscious decisions. Humans are the ones capable of foreseeing events and dealing with critical decisions regardless of past information.
And, by the way, humans are behind the curtains configuring the decision-making process of machines. For all that, machines are trained and better than us at particular tasks.
All that was explored by Tom Davenport (pictured), the president’s distinguished professor of information technology and management at Babson College, co-founder of the International Institute for Analytics and fellow of the MIT Initiative for the Digital Economy. He spoke with Jeff Frick, host of theCUBE, SiliconANGLE Media’s livestreaming studio, during the BizOps Manifesto Unveiled event. They discussed the BizOps Coalition, BizOps Manifesto and AI-decision making. The interview was condensed for clarity. (* Disclosure below.)
I came across your LinkedIn post and the first sentence grabbed my attention: “I’m always interested in new attempts to address long-term issues in how the technology works within businesses.” What did you see in BizOps that kind of addresses one of these really big, long-term problems?
Davenport: The long-term problem is that we’ve had a poor connection between business people and IT people; between business objectives and the IT solutions that address them. And so BizOps is a new attempt to deal with that issue, with a new framework.
Do you think doing it this way with the BizOps Coalition, getting a collection of like-minded individuals and companies together and even having a manifesto — is that what it takes to start to see some results?
Davenport: I think certainly no one vendor or organization can pull this off single-handedly. It does require a number of organizations collaborating and working together. So I think a coalition is a good idea, and a manifesto is just a good way to lay out what you see is the key principle of the idea, and that makes it much easier for everybody.
You’re the data guy. You’re the analytics guy. And a big piece of this is data analytics and moving to data-driven decisions. As you think of analytics and machine learning driving decisions — beyond supporting decisions but actually starting to make decisions in machine time — what does that mean to you?
Davenport: The nice thing about automating decisions with contemporary AI tools is that we can ensure that data and analytics get brought into the decision without any possible disconnection. Now, I think humans still have something to add here, and we often will need to examine how that decision is being made and maybe even have the ability to override it.
What are the attributes of the decision that enable us to more easily automate it? And how do you see that morphing over time, both as the data to support that as well as our comfort level enables us to turn more actual decisions over to the machine?
Davenport: We need data, and the data that we have to kind of train our models have to be high quality and current. We need to know the outcomes of that data. Most machine learning models, at least in business, are supervised, and that means we need to have labeled outcomes in the training data. But you know, the pandemic that we’re living through is a good illustration of the fact that the data also have to be reflective of the current reality.
And one of the things that we are finding out quite frequently these days is that the data that we have does not reflect what it’s like to do business in a pandemic. So I think that’s one of the key things with automated decision-making. We have to make sure that the data from the past is a good guide to what’s happening in the present and in the future, as far as we understand it.
How should people really be thinking about the AI applications where I can apply this technology to get direct business value?
Davenport: AI projects that are working well are what I call the low-hanging fruit ones. Some people even refer to it as boring AI, sucking data out of a contract in order to compare it with a bill of lading for what arrived at your supply chain. Companies can save or make a lot of money with that kind of comparison. It’s not the most exciting thing, but AI is really good at those narrow kinds of tasks. It’s not so good at the really big moonshots, like curing cancer, figuring out what’s the best stock or bond under all circumstances, or even autonomous vehicles.
Here’s the full video interview, part of SiliconANGLE’s and theCUBE’s coverage of the BizOps Manifesto Unveiled event. (* Disclosure: TheCUBE is a paid media partner for the BizOps Manifesto Unveiled event. Neither Broadcom Inc., the sponsor for theCUBE’s event coverage, nor other sponsors have editorial control over content on theCUBE or SiliconANGLE.)
THANK YOU