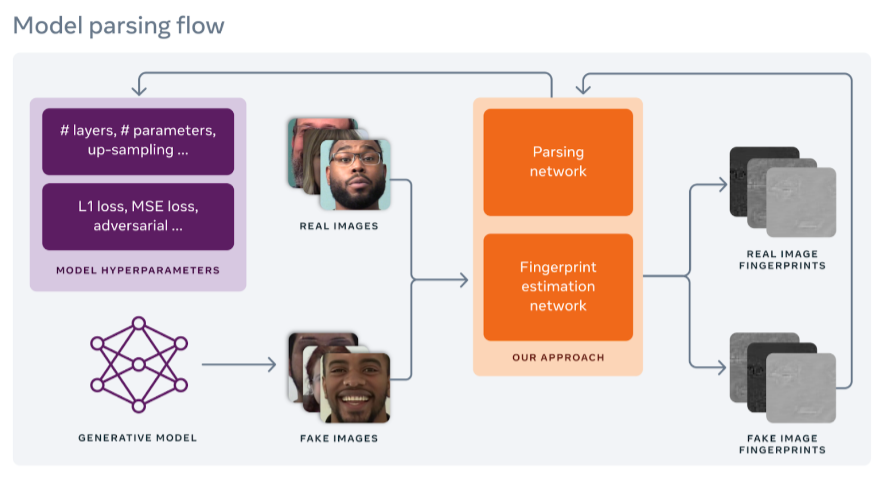
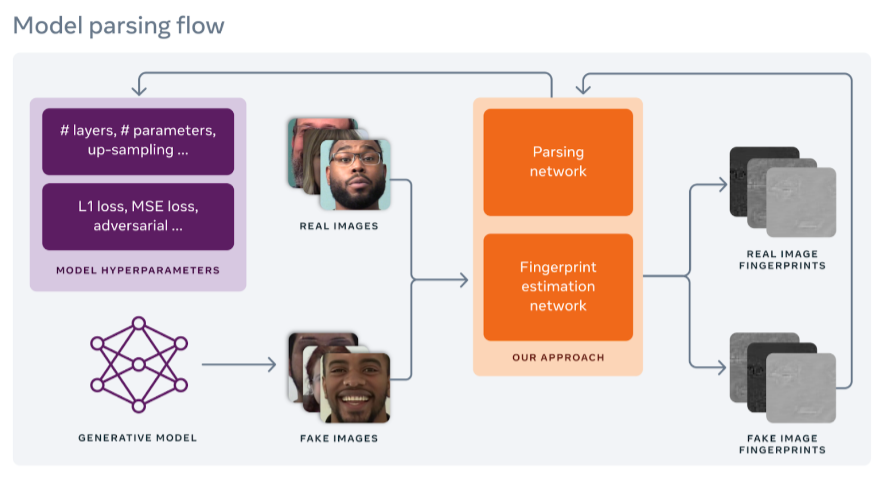
Facebook Inc.’s artificial intelligence research unit has teamed up with Michigan State University on what the company says is a new and extremely accurate tool that can not only detect deepfakes but also understand how they were were built.
Deepfakes is the name for fake, computer-manipulated images or videos that have been altered in hard-to-detect ways to make people appear to have said something they didn’t or look like they were in places that they weren’t. They’re often used to defame notable people, and their widespread use on the web has become a growing concern.
The problem with deepfakes is that the technology used to create them has increasingly become more accessible and easier to use. Most deepfakes look extremely realistic too, so they can be very embarrassing for the victims they portray. The fact that they’re also difficult to identify also means they’re often used to meddle in politics and elections.
As one of the world’s most widely used social media outlets, Facebook is one of the prime targets for people looking to distribute deepfakes. So naturally Facebook feels it has an obligation to stamp them out, or at least critics do, and the company is using some advanced AI techniques to try to do exactly that.
Partnering with MSU, Facebook AI has created what it says is an entirely new research method that relies on reverse engineering deepfakes to identify them and also the generative AI model that was used to create it.
In a blog post, Facebook’s researchers said they opted to use a method based on reverse engineering primarily because it enables them to discover the source of a particular deepfake. By source, it means the specific AI model used to create it. That’s important, the researchers said, because it can help them to identify instances of “coordinated disinformation” based on deepfakes.
The reverse engineering method scans each deepfake image or video using a “Fingerprint Estimation Network” that tries to uncover details of the fingerprint left by the generative model used to create it. The generative fingerprints can be likened to the device fingerprints that can be used to identify a specific camera used to produce a photograph, the researchers explained.
“Image fingerprints are unique patterns left on images generated by a generative model that can equally be used to identify the generative model that the image came from,” the researchers wrote in a blog post.
Facebook calls this a “model parsing approach” that makes it possible to gain a critical understanding of the generative model used to create a particular deepfake. Although there are numerous generative models in existence, most of them are well-known. And even in the case of an unknown generative model, Facebook said, the method it has developed can be used to identify different deepfakes as being produced from that same model.
Facebook and the MSU research team tested its approach by putting together a fake image dataset of 100,000 deepfakes that were created by 100 publicly available generative models. Facebook didn’t provide specific results, but it said the approach performs “substantially better” than previous methods used to try to identify deepfakes and the generative models used to create them.
Analyst Holger Mueller of Constellation Research Inc. characterized the war being waged on deepfakes as a battle between the good guys and the bad guys. He said that it’s a battle that is now being waged with AI, and that it’s currently being won by the bad guys, as deepfakes have progressed and become more difficult to detect in recent months.
“That’s why it’s good to see Facebook working with Michigan State University to help the good guys catch up by attacking the deepfakes on the algorithmic AI side,” Mueller said. “The good thing about this new method of detecting them is that once a certain kind of deepfake has been detected, researchers can then use that knowledge to flag and label others that were created using the same model. It’s good to see AI coming to the rescue.”
Facebook and the MSU team said they’re planning to open-source the dataset they used, the code and the trained models to the wider research community to facilitate more research in deepfake detection, image attribution and reverse engineering of generative models.
“Our research pushes the boundaries of understanding in deepfake detection, introducing the concept of model parsing that is more suited to real-world deployment,” Facebook’s AI team wrote. “This work will give researchers and practitioners tools to better investigate incidents of coordinated disinformation using deepfakes as well as open up new directions for future research.”
Facebook isn’t alone in trying to combat the problem of deepfakes. Microsoft Corp. last year announced the Microsoft Video Authenticator tool that analyzes photos and videos to provide a “confidence score” about whether or not it has been manipulated. The tool is part of Microsoft’s Defending Democracy initiative that aims to fight disinformation, protect voting and secure election campaigns.
THANK YOU