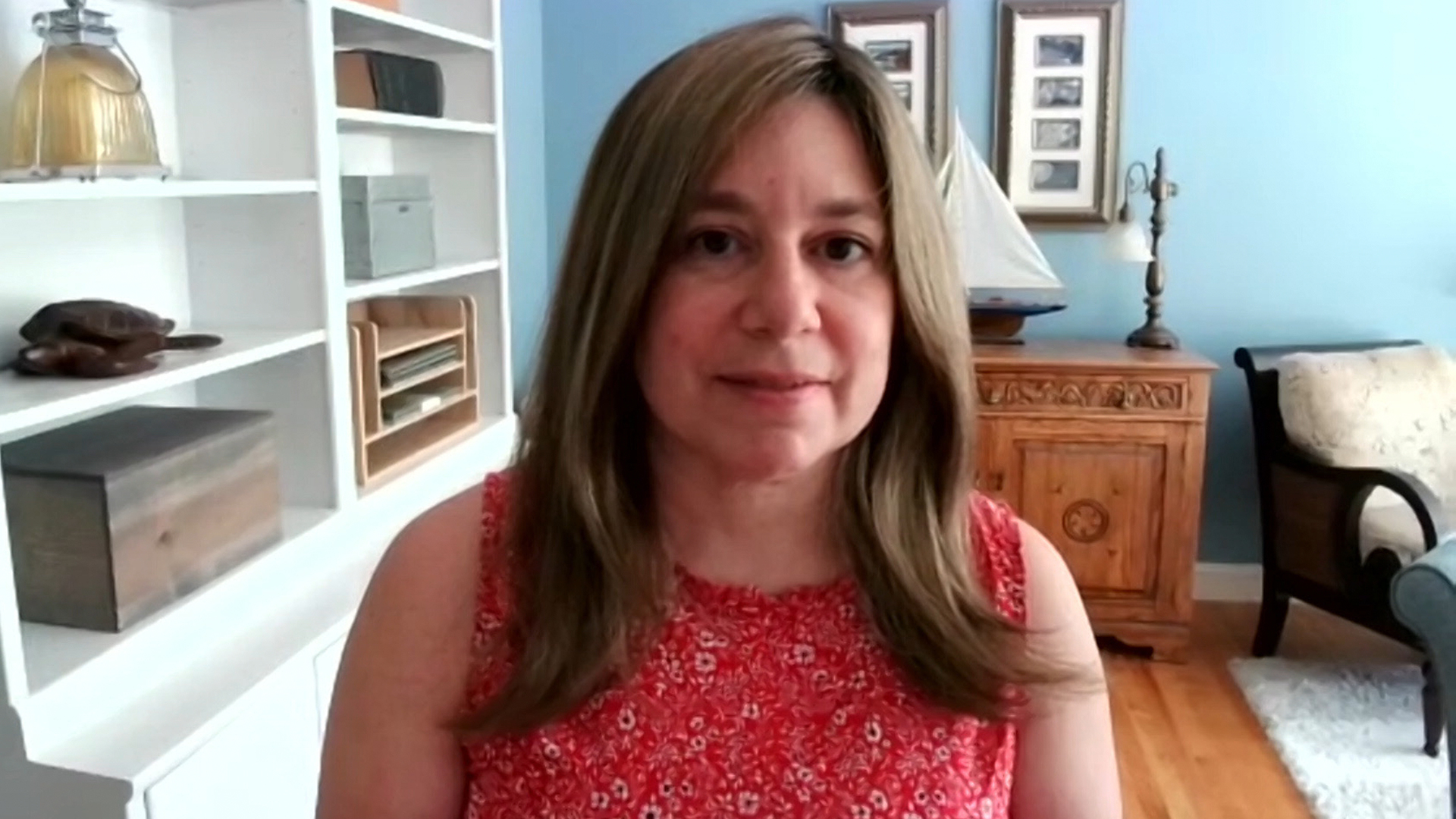
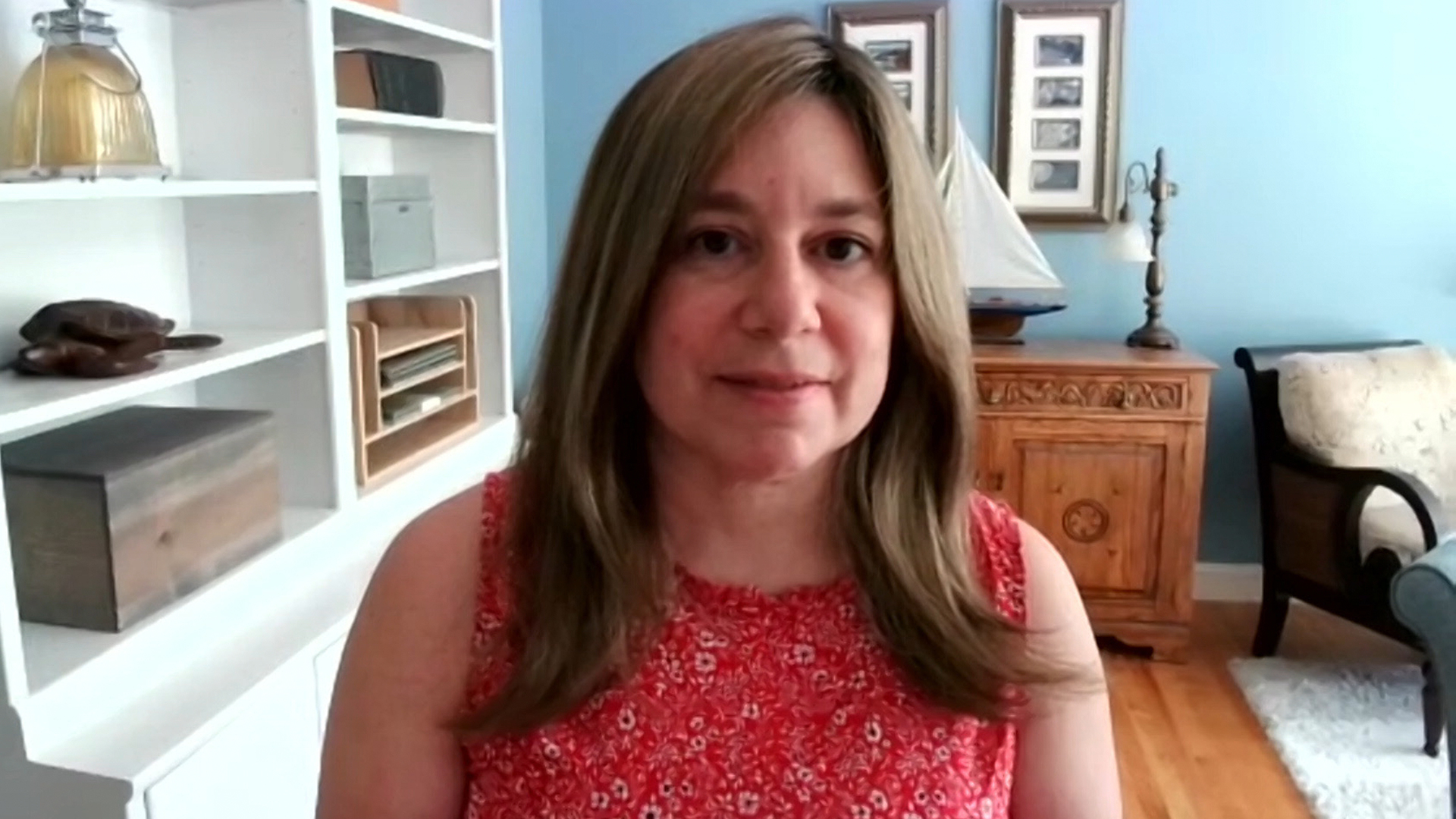
For the past decade, enterprises have been investing heavily in people, processes and technology specifically designed to gain insights from data, better serve customers and drive new revenue streams. But as data becomes increasingly distributed, the world of data is changing — making governance more challenging, especially where operational use cases are a priority.
“You create this massive complexity to managing the data, governing the data, orchestrating the data, because it’s not just a centralized data warehouse environment anymore,” said Michele Goetz (pictured), vice president and principal analyst of Forrester Research Inc. “You have a highly diverse and distributed landscape. What the struggle then becomes is: How do you trust the data? How do you govern it and secure and protect that data?”
So what are companies thinking through when it comes to data governance? Goetz spoke with Dave Vellante, host of theCUBE, SiliconANGLE Media’s livestreaming studio, during the Data Citizens ’21 event. They discussed how businesses are governing and managing data in an increasingly complex digital landscape. (* Disclosure below.)
Forrester Research spends a lot of time researching technology trends through services such as Forrester Market Insights, and Goetz was able to speak to a lot of the ways that enterprises are facing the challenges of managing data. She sees multiple trends. For one, a lot of companies are creating a federal model, where a digital core team is supported by other departments that aren’t part of the digital team’s core business group. However, edge computing can mean other ways to manage data teams are better.
“We’re … seeing data and analytics and governance teams come together under chief data officers or chief data and analytics officers,” Goetz said. “[But] when you push data into the edge, the goal is that you’re actually driving an experience and an application. In that case, we are seeing data engineering teams starting to be incorporated into the solutions teams that are aligned to lines of business or divisions themselves.”
Oftentimes for edge computing, there needs to be a solution consultant who is also overseeing value-based portfolio management for new data use cases to keep up with the pace of the business, according to Goetz. It’s usually a data engineering team that is part of the DevOps to execute on that.
“So really the balance is: We need the core, we need to get to the insights and build our models for AI,” Goetz said. “And then the next piece is: How do you activate all that? — and there’s a team over there to help. So it’s really spreading the wealth and expertise where it needs to go.”
The reality is that as technology moves into more of an artificial intelligence and machine learning type of model, more context is necessary. This brings a balancing act between data globally, which is what data engineers can support, and the unique context of the data that is actually related a company’s value and outcome — as well as the feature engineering that is being done on the machine learning models, according to Goetz.
“There has to be a really tight link and collaboration between the data engineers, the data scientists and analysts, and the business stakeholders themselves,” she said. “So data teams aren’t just sitting in the basement or in another part of the organization and digitally … disconnected anymore. You’re finding that they’re having to work much more closely and side by side with their colleagues and stakeholders.”
At the end of the day, it’s really about what’s being shared and how it’s shared, according to Goetz. As data teams build platforms, everybody is usually contributing into some sort of library where their components and products are being ascribed to — which is able to help different teams grab those components and build out what those solutions are going to be. This enables people who aren’t data scientists to collaborate.
“This is where a lot of the auto [machine learning] begins, because those who are less data science-oriented but can build an insight pipeline can grab all the different components — from the pipelines, to the transformations, to capture mechanisms, to bolting into the model itself and allowing that to be delivered to the application,” she said, adding that it’s about “balancing out between process and platforms that enable and encourage and almost force you to collaborate and manage through sharing.”
Here’s the complete video interview, part of SiliconANGLE’s and theCUBE’s coverage of the Data Citizens ’21 event. (* Disclosure: TheCUBE is a paid media partner for Data Citizens ’21. Neither Collibra NV, the sponsor for theCUBE’s event coverage, nor other sponsors have editorial control over content on theCUBE or SiliconANGLE.)
THANK YOU