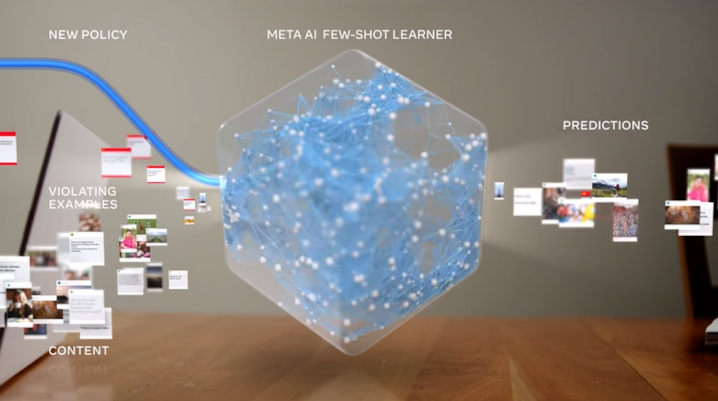
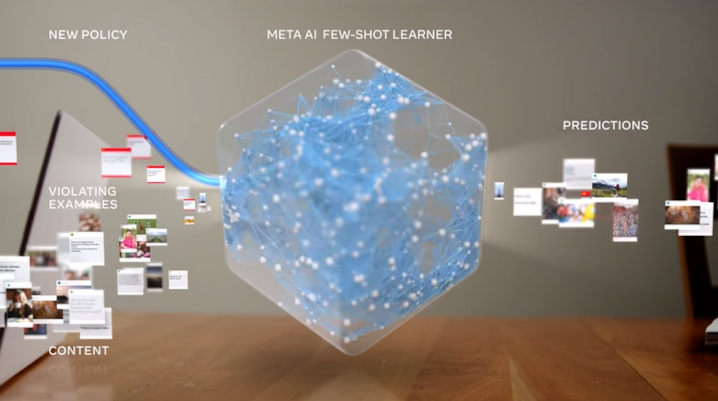
The artificial intelligence unit of Meta Platforms Inc., previously Facebook Inc., today detailed a new machine learning system that will help the company combat harmful content across its social media platforms.
The system is known as Few-Shot Learner, or FSL for short. The main advantage of FSL over earlier technologies, Meta says, is its ability to adapt to new types of harmful content that appear on the company’s social media platforms in weeks rather than months.
To learn a new skill, an AI model must be given a training dataset from which it can glean what task it needs to carry out and how. The training dataset consists of records relevant to the skill the AI is being taught. Each record includes a label, which is a snippet of metadata that helps the neural network learn.
Typically, Facebook trains the AI models it uses to detect harmful content with training datasets consisting of anywhere from thousands to millions of harmful content examples. The company not only has to assemble the examples but also add in the labels, or metadata snippets, that the AI models require to learn effectively. The process takes months in some cases, Meta’s AI researchers wrote today in a blog post detailing the FSL system.
FSL can learn to detect new types of harmful content in weeks instead of months. Meta’s researchers achieved this speedup by reducing the amount of training data that the system requires. In some cases, Meta says, FSL can learn to detect new types of harmful content without a single labeled example.
FSL’s versatility stems from its use of an emerging AI approach. “This new AI system uses a relatively new method called ‘few-shot learning,’ in which models start with a large, general understanding of many different topics and then use much fewer, and in some cases zero, labeled examples to learn new tasks,” Meta’s researchers explained.
The training workflow that Meta used to develop FSL consists of three main phases.
In the first phase, the system is trained on billions of “generic and open-source language examples.” Then, FSL is given examples of content that breaches Meta’s community standards, as well as borderline content. FSL’s ability to quickly adapt to new tasks emerges during the third training phase. In this phase, FSL receives a condensed snippet of text explaining a new content policy, which the system can consult to find harmful posts.
“Unlike previous systems that relied on pattern-matching with labeled data, FSL is pretrained on general language, as well as policy-violating and borderline content language, so it can learn the policy text implicitly,” the researchers wrote.
Meta has deployed FSL in production to reduce the prevalence of harmful content across Facebook and Instagram. The company’s researchers measured the effectiveness of the system through a series of tests. By comparing the prevalence of harmful content before and after the system was rolled out, Meta determined that FSL is capable of successfully detecting posts missed by traditional technologies.
According to Meta, FSL outperforms state of the art few-shot learning methods by up to 55% in some cases. On average, the social network logged a 12% improvement.
“We believe that FSL can, over time, enhance the performance of all of our integrity AI systems by letting them leverage a single, shared knowledge base and backbone to deal with many different types of violations.” Meta’s researchers stated. “But it could also help policy, labeling, and investigation workflows to bridge the gap between human insight and classifier advancement.”
THANK YOU