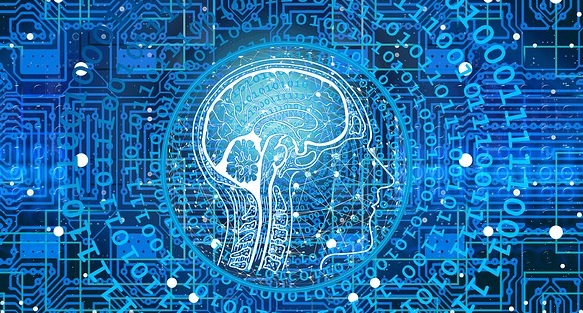
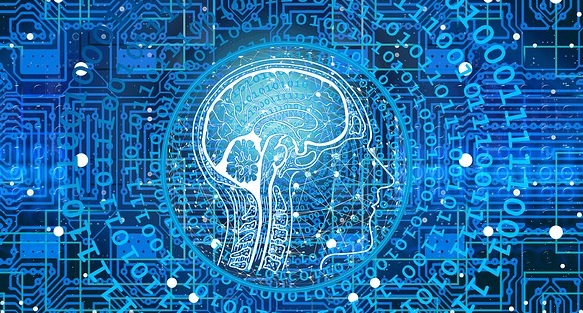
It would be difficult to overstate the current wave of hype surrounding generative AI.
The November release of ChatGPT, an artificial intelligence tool capable of generating human-like text, ignited a frenzy of publicity compared by some to the introduction of the first iPhone in 2007.
With recent news headlines like “Generative AI is a legal minefield,” “Generative AI should make haste slowly” and even “Generative AI is us playing God,” the field has exploded into public consciousness. ChatGPT has fueled a growing realization that AI may finally be on its way to singularity, a process for exceeding human intelligence.
“It’s awakened in everybody a sense that maybe the singularity is closer than we thought,” John Hennessy, chairman of Google parent Alphabet Inc., said during an appearance at a technology conference in mid-February.
While public attention has been largely focused on ChatGPT’s ability to write credible college essays, compose songs and generate to-do lists, the more significant story concerning the rise of generative AI involves the development of an ecosystem around it. There are a number of small, startup companies building an intriguing set of tools that could have significant impact in the years ahead. And, no, a chatbot did not write any part of this story. (* Disclosure below.)
The field of generative AI involves an ability to produce original and realistic content that resembles existing data. General AI could perceive and classify this story; generative AI could create this story on demand. However, the process for AI to make this kind of leap effectively is enormously difficult and complex. OpenAI, the company behind ChatGPT, used an estimated 45 terabytes of text data to build its GPT-3 model. That equates to over 292 million pages of documents.
This is why the world of generative AI has been largely confined to the most well-funded players. Microsoft reportedly made a $10 billion investment in OpenAI. DeepMind, developer of an AI system for processing complex mathematical problems, is an Alphabet subsidiary. The Make-A-Video generative AI tool that produces videos from text is owned by Meta Platforms Inc.
Yet even the largest technology players in the world can’t do it all when it comes to generative AI. Bringing large foundational models, pre-trained on massive volumes of data, to production requires innovation. A growing number of smaller companies are developing new tools for training highly dense models.
One company that has set out to address the need for easier-to-manage models is Neural Magic Inc. The company has developed logic to simplify optimization of machine learning models by making them smaller while still retaining key foundational elements.
“In many cases, we can make a model 90% to 95% smaller, even smaller than that in research,” Brian Stevens, chief executive officer of Neural Magic, said in a recent interview with theCUBE, SiliconANGLE Media’s livestreaming studio. “So now, all of a sudden, you get this much smaller model that is just as accurate.”
Another firm seeking to improve model accuracy is ArthurAI Inc., a New York-based startup that secured $42 million in new funding last fall. The company built a software platform to automatically detect data drift and fairness issues in AI applications, complete with an alerting tool for developers when accuracy goes awry. Arthur.ai’s platform can process up to 1 million data operations per second.
Developers represent a key constituency in the future of generative AI. Hugging Face Inc. has created a platform, similar to GitHub, where developers can host open-source AI models and training datasets.
Hugging Face is also reportedly working on a language generation tool that will compete with OpenAI’s ChatGPT. In mid February, Amazon Web Services Inc. announced that it will expand its partnership with Hugging Face, and the startup plans to build the next version of its language model on the cloud giant’s platform.
“There is a broad range of enterprise use cases that we don’t even talk about, and it’s because transformative generative AI capabilities and models are not available to millions of developers,” Swami Sivasubramanian, vice president of database, analytics and machine learning at AWS, said in an interview with SiliconANGLE. “With this partnership, Hugging Face and AWS will be able to democratize AI for a broad range of developers. We can accelerate the training, fine-tuning and deployment of large language models.”
The extension of AWS’ partnership supports cloud-native data orchestration, an area receiving more attention as generative AI applications expand. One startup at the center of this emerging field is Astronomer Inc., a platform for leveraging open-source Apache Airflow.
Originally incubated within the Airbnb GitHub, Airflow uses Python programming to schedule and monitor workflows. Astronomer’s Astro is an Airflow-powered, cloud-native data orchestration platform designed to help data teams and practitioners manage the myriad tools and procedures associated with AI applications.
The inherent complexity of generative AI is driving a desire for tools that can streamline the development and deployment process. Startups such as OctoML Inc. and Anyscale Inc. are focused on providing solutions in this area that are designed to make model execution easier.
OctoML was spun out of the University of Washington by the creators of the machine learning portability and performance stack Apache TVM. Many consumers rely on this tool, and they are probably unaware of it. TVM enables models on the hardware backend, and it has become a key component of the Amazon Alexa platform.
“The company’s mission is to enable customers to deploy models very efficiently in the cloud and enable them to do it quickly, run fast and run at a low cost, which is something that’s especially timely right now,” Luis Ceze, co-founder and chief executive officer of OctoML, said in an interview with SiliconANGLE. “Getting the right hardware to run these incredibly hungry models is hard. So, we also help customers deal with hardware availability problems, as well as the solutions part.”
Anyscale grew out of Ray, an open-source framework for distributed machine learning. Users can leverage native libraries, such as Ray Tune and Ray Serve, to scale the most compute intensive machine learning workloads. Anyscale’s goal is to help developers make the transition from AI creation on a single computer to implementation across thousands of machines.
“What we are building at Anyscale is really trying to get to the point where, as a developer, if you know how to program on your laptop in Python for example, then that’s enough,” Robert Nishihara, co-founder and chief executive officer of Anyscale, said in an interview last year with SiliconANGLE. “Then you can do AI, you can get value out of it, scale it and build the kinds of incredibly powerful AI applications that companies like Google, Facebook and others can build.”
One of the startup companies looking to democratize AI building is Stability AI Ltd. Founded in 2019, Stability has emerged as a central player in the generative AI world due to the popularity of Stable Diffusion, a neural network capable of generating images from text prompts.
Stability has built a massive cluster of over 4,000 Nvidia A100 GPUs running on AWS to train AI systems. Stable Diffusion alone is trained on a subset of 2.3 billion images from a dataset that contains 5.85 billion image-text pairs. In advance of February’s MWC in Barcelona, Qualcomm Inc. announced that its AI research team successfully installed Stable Diffusion on a smartphone.
Progress in the field of generative AI has not been without its share of controversy. Stable Diffusion’s use of large image datasets triggered a copyright lawsuit in January by artists claiming unauthorized use of their work. Microsoft, GitHub and OpenAI are the subject of a class action motion, accused of violating copyright law by leveraging Copilot, a code-generating AI system, to program using licensed software.
The courts will ultimately define the boundaries for AI development. Yet there is no mistaking the rapid advancement made in the field and the subsequent growth of a new ecosystem of companies around it. Is this generative AI’s moment?
“AI is at an inflection point, setting up for broad adoption reaching into every industry,” Nvidia Chief Executive Jensen Huang, said during a recent call with industry analysts. “From startups to major enterprises, we are seeing accelerated interest in the versatility and capabilities of generative AI. Generative AI’s versatility and capability has triggered a sense of urgency at enterprises around the world to develop and deploy AI strategies.”
(* Disclosure: This story provides further insight from an ongoing series of programs produced by theCUBE, SiliconANGLE Media’s livestreaming studio, to explore technology trends within the Amazon Web Services Inc. startup ecosystem. Neither AWS, nor other partners, have editorial control over content on theCUBE or SiliconANGLE.)
THANK YOU