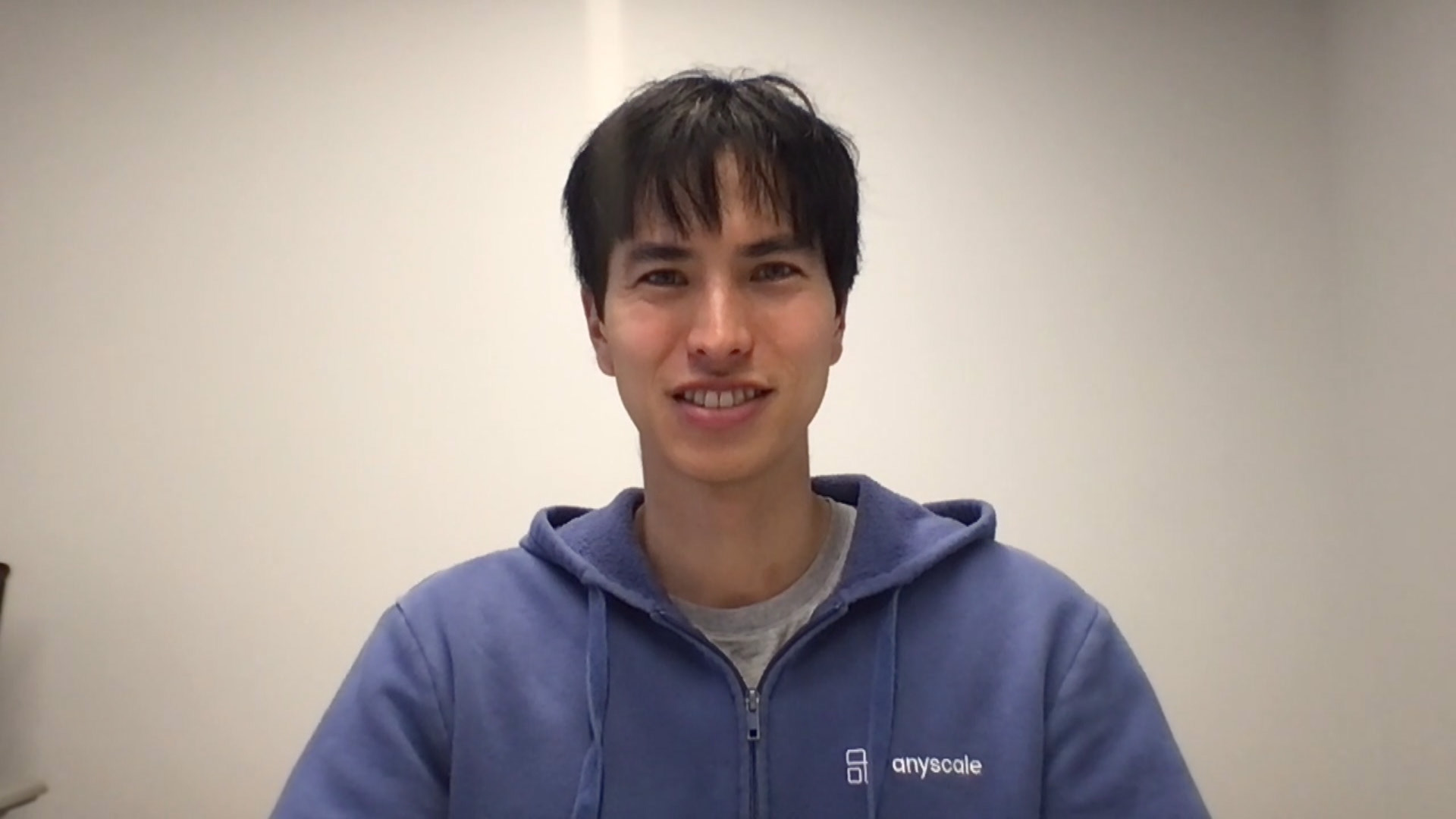
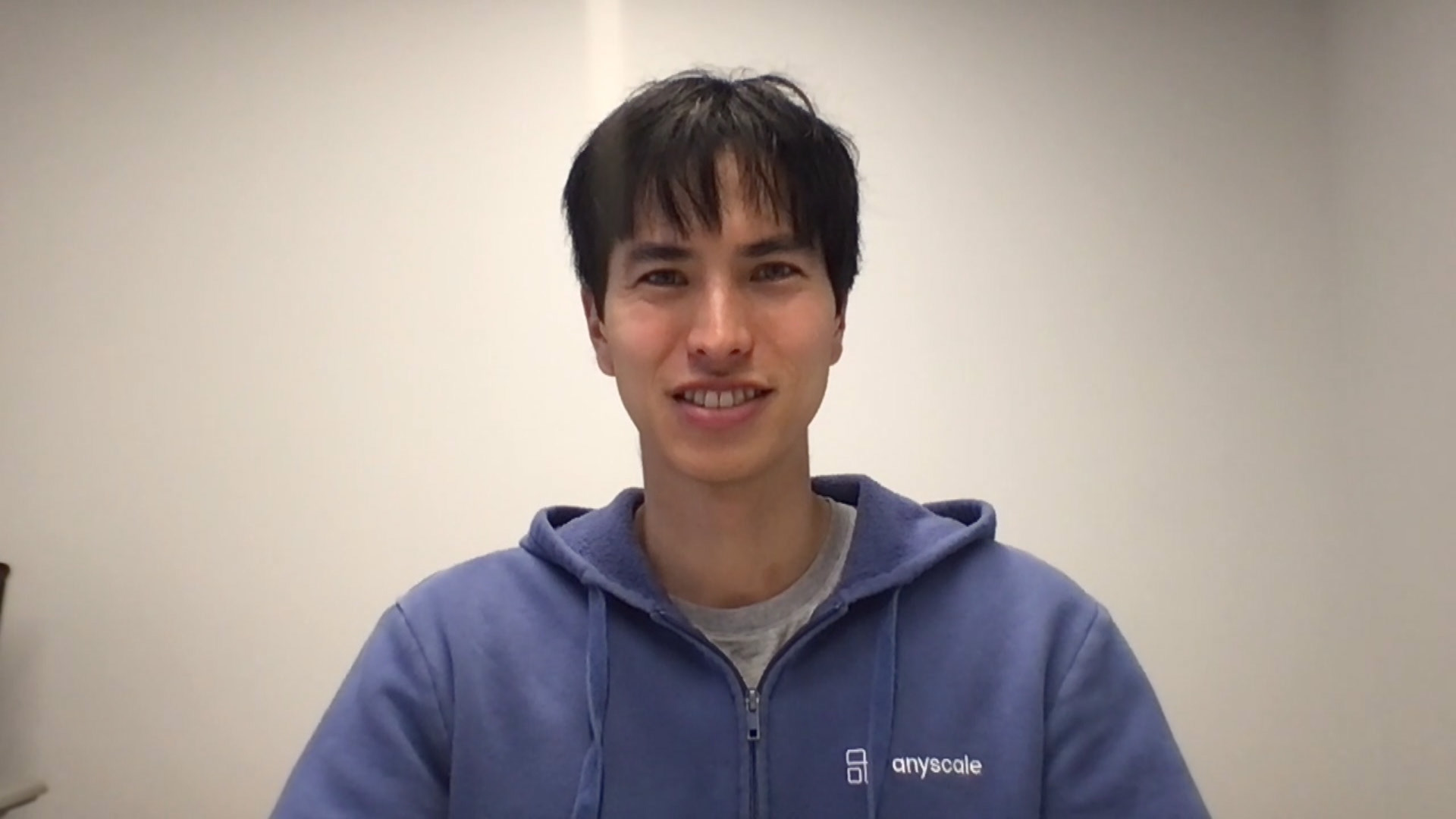
Foundational machine learning models are typically large — trained using unlabeled data at scale, to further be adapted to a wide spectrum of specific tasks.
But, given their depth, these models also require large amounts of compute resources to perform at a meaningful scale. And that computing at scale is the problem that Anyscale Inc. is working to solve.
“One of the reasons many AI projects and initiatives fail or don’t make it to production is the need for this scale, the infrastructure lift to actually make it happen,” said Robert Nishihara (pictured), co-founder and chief executive officer of Anyscale. “Our goal here with Anyscale and Ray is to make scalable computing easy. So that as a developer or as a business that wants to get value out of AI, all you need to know is how to program on your laptop.”
Nishihara spoke with theCUBE industry analyst John Furrier at the AWS Startup Showcase: “Top Startups Building Generative AI on AWS” event, during an exclusive broadcast on theCUBE, SiliconANGLE Media’s livestreaming studio. They discussed the critical importance of infrastructure scalability in the exploitation of machine learning for the enterprise. (* Disclosure below.)
The industry can’t seem to get enough of foundational models, even as the term dominates the popular discourse around AI. These preexisting models help companies get to the value and scale faster. And this is what makes them so highly sought after, according to Nishihara.
“They enable businesses and developers to get value out of machine learning, to use machine learning off the shelf with these large models that have been trained on tons of data and that are useful out of the box,” he explained. “And then, as a business or as a developer, you can take those foundational models and repurpose, fine-tune, or adapt them to your specific use case and what you want to achieve.”
The cost of training purpose-built ML models from scratch can be incredibly steep, and so foundational models derive their importance from circumventing that process for enterprises. But in harnessing the foundational models themselves, there are three primary processes: training, refining and adapting. Anyscale, and its Ray distributed ML platform, are capable of handling all three workloads, according to Nishihara.
“The reason that Ray and Anyscale are important here is that building and using foundation models requires a huge scale. It requires a lot of data. It also requires a lot of compute, GPUs, TPUs, and other resources,” he said. “To actually take advantage of that and build these scalable applications, there’s a lot of infrastructure that needs to happen under the hood.”
Enterprises can, alternatively, acquire for themselves the infrastructural resources needed for in-house operations. However, doing so can saddle the ops and dev teams with the added task of managing infrastructure, when they could be focusing squarely on rapid product development, according to Nishihara.
It can be said that distributed ML platforms like Ray are, with AIOps, what the cloud is for data centers. And a paradigm where companies don’t have to figure out their own infrastructures will foster a magnitude increase in creativity, Nishihara explained.
“With Ray and Anyscale, we’re going to remove the infrastructure from the critical path so that as a developer or a business, all you need to focus on is your application logic, what you want the the program to do, what you want your application to do, and how you want the AI to actually interface with the rest of your product,” he said.
Ray is an open-source project that was created by Nishihara and his colleagues while at the University of California, Berkeley as a simple-to-use way to build and run scalable apps. Anyscale is the consolidated platform that provides Ray as a managed service for end users.
“Basically, we will run Ray for you in the cloud and provide a lot of tools around the developer experience, managing the infrastructure and providing more performance and superior infrastructure.”
Compute needs of AI-reliant companies have been growing at a rate of around 35x every 18 months, according to Nishihara. This fast-paced demand has resulted in large-scale players, such as Uber, Shopify and Netflix, turning to distributed application frameworks like Ray for their ML infrastructure needs.
Here’s the complete video interview, part of SiliconANGLE’s and theCUBE’s coverage of the AWS Startup Showcase: “Top Startups Building Generative AI on AWS” event:
(* Disclosure: Anyscale Inc. sponsored this segment of theCUBE. Neither Anyscale nor other sponsors have editorial control over content on theCUBE or SiliconANGLE.)
THANK YOU