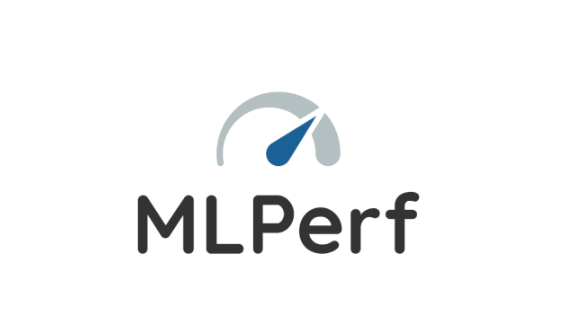
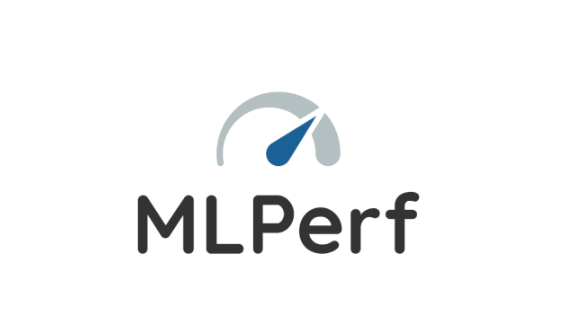
MLCommons, the open engineering consortium for benchmarking the performance of chipsets for artificial intelligence, today unveiled the results of a new test that’s geared to determine how quickly hardware can run the most advanced AI models.
Nvidia Corp.’s most advanced chips were the top performers in tests on a large language model, though Intel Corp.’s hardware came a surprisingly close second.
MLCommons is a vendor-neutral, multi-stakeholder organization that offers a level playing field for chipmakers to report on various aspects of their AI performance using the MLPerf benchmark tests. Today, it announced the results of its new MLPerf Inference 3.1 benchmarks, which come in the wake of its 3.0 results in April.
Notably, this is the first time that MLCommons has offered benchmarks for testing AI inference, though it’s not the first time it has attempted to validate performance on the large language models that power generative AI algorithms such as ChatGPT.
In June, MLCommons revealed the MLPerf 3.0 Training benchmarks that covered LLMs for the first time. However, training LLMs is quite a different thing from running inference, which refers to powering these models in production. With inference, LLMs are fundamentally performing a generative task, such as writing sentences or creating images. In training, these models are simply acquiring information.
MLCommons said its latest benchmark is based on an LLM with 6 billion parameters, known as GPT-J 6B. It’s designed to summarize texts from news articles published by Cable News Network and the Daily Mail. It simulates the inference part of AI data crunching, which powers generative AI tools.
Nvidia submitted the results of two of its most advanced chips, including the GH200 Grace Hopper Superchip, which links a Hopper graphic processing unit with a Grace central processing unit in a single chip. The combination is said to provide more memory, bandwidth and the ability to shift tasks between the GPU and an Arm-based CPU to optimize performance.
In addition, Nvidia submitted its HGX 100 system, which packs eight of its most advanced H100 GPUs and delivers exceptionally high throughput.
Nvidia’s hardware displayed the most impressive results across all of MLPerf’s data center tests, showing the best performance for tasks including computer vision, speech recognition and medical imaging. They also came out tops in terms of more demanding workloads such as LLM inference and recommendation systems.
The GH200 Grace Hopper Superchip proved superior to the HGX 100, with performance averaging around 17% better. That’s not surprising, as the Grace Hopper chipset has more processing power and supports faster networking speeds between Arm-based CPUs and the GPU.
“What you see is that we’re delivering leadership performance across the board, and again, delivering that leadership performance on all workloads,” Dave Salvator, Nvidia’s accelerated computing marketing director, said in a statement.
Karl Freund, founder and principal analyst of Cambrian-AI Research LLC, wrote in Forbes that Nvidia’s results show why it remains the one to beat and why it dominates the AI industry. “As always, the company ran and won every benchmark,” Freund said. “The most interesting thing was the first submission for Grace Hopper, the Arm CPU and the Hopper GPU Superchip, which has become the Nvidia fleet’s flagship for AI inferencing.”
Although Nvidia came out tops, Intel Corp.’s Habana Gaudi2 accelerators, which are produced by its subsidiary Habana Labs, came a surprisingly close second to Nvidia’s chips. The results showed that the Gaudi2 system was just 10% slower than Nvidia’s system.
“We’re very proud of the results of inferencing, (as) we show the price performance advantage of Gaudi2,” said Eitan Medina, Habana’s chief operating officer.
It’s also worth noting that Intel’s Habana Gaudi2 is based on a seven-nanometer manufacturing node, in contrast to the five-nanometer Hopper GPU. What’s more, Intel promises its system will become even faster for AI inference tasks when it’s updated with something called FP8 precision quantization later this month.
Intel promises that the update will deliver a two-times performance boost. In addition, Intel is also said to have a 5nm Gaudi3 chipset in the works that is likely to be announced later this year.
Intel added that its Habana Gaudi2 chip will be cheaper than Nvidia’s system, though the company has not yet revealed the exact cost of that chip.
Nvidia is not standing still, though, saying it will soon roll out a software update that will double the performance of its GH200 Grace Hopper Superchip in terms of its AI inference ability.
Other competitors who submitted results include Google LLC, which previewed the performance of its latest Tensor Processing Units, but fell short of Nvidia’s numbers. Qualcomm Inc. also did well, with its Qualcomm Cloud AI100 chipset showing strong performance resulting from recent software updates. Qualcomm’s results were all the more impressive as its silicon consumes far less power than that of its rivals.
THANK YOU