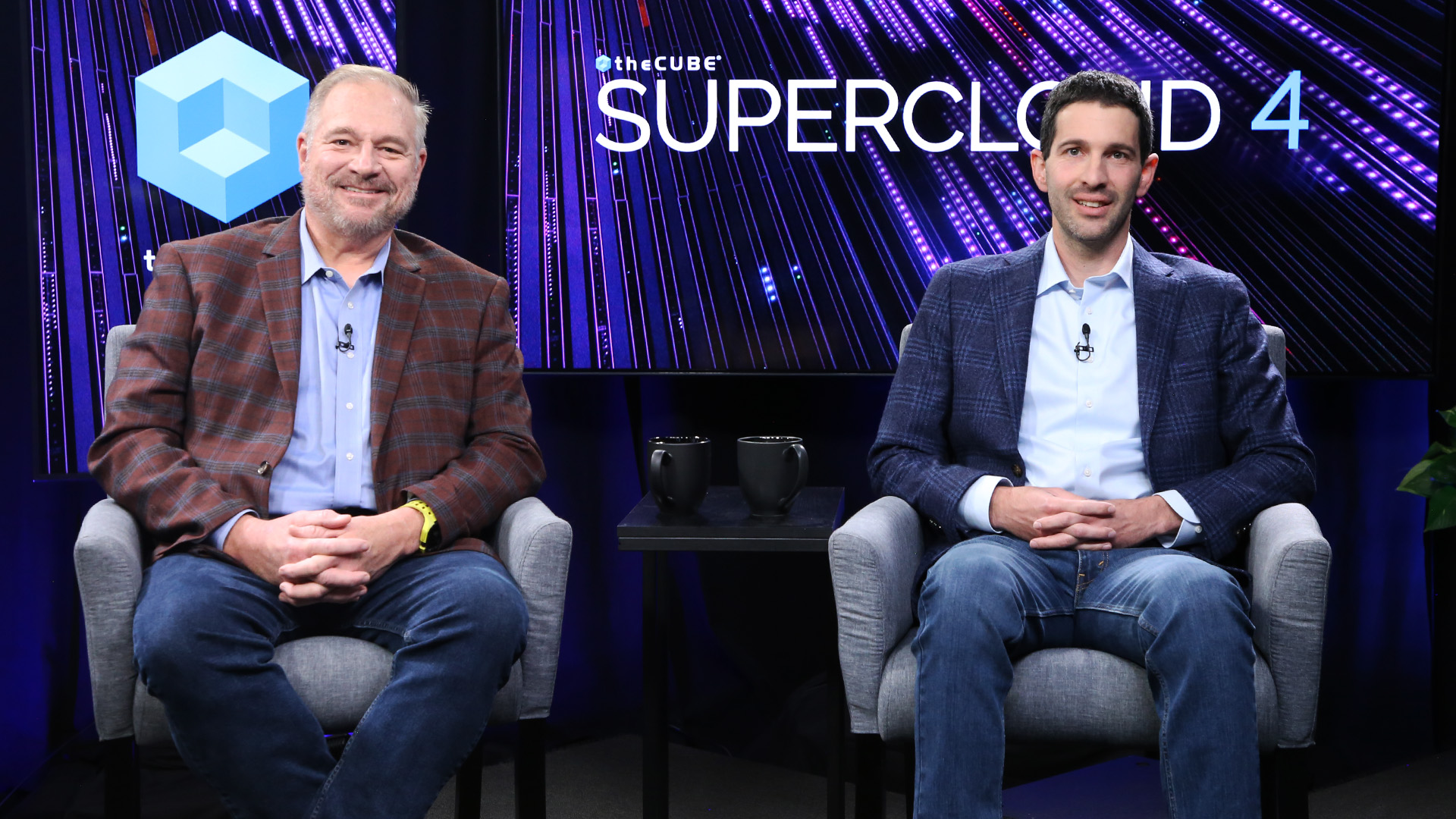
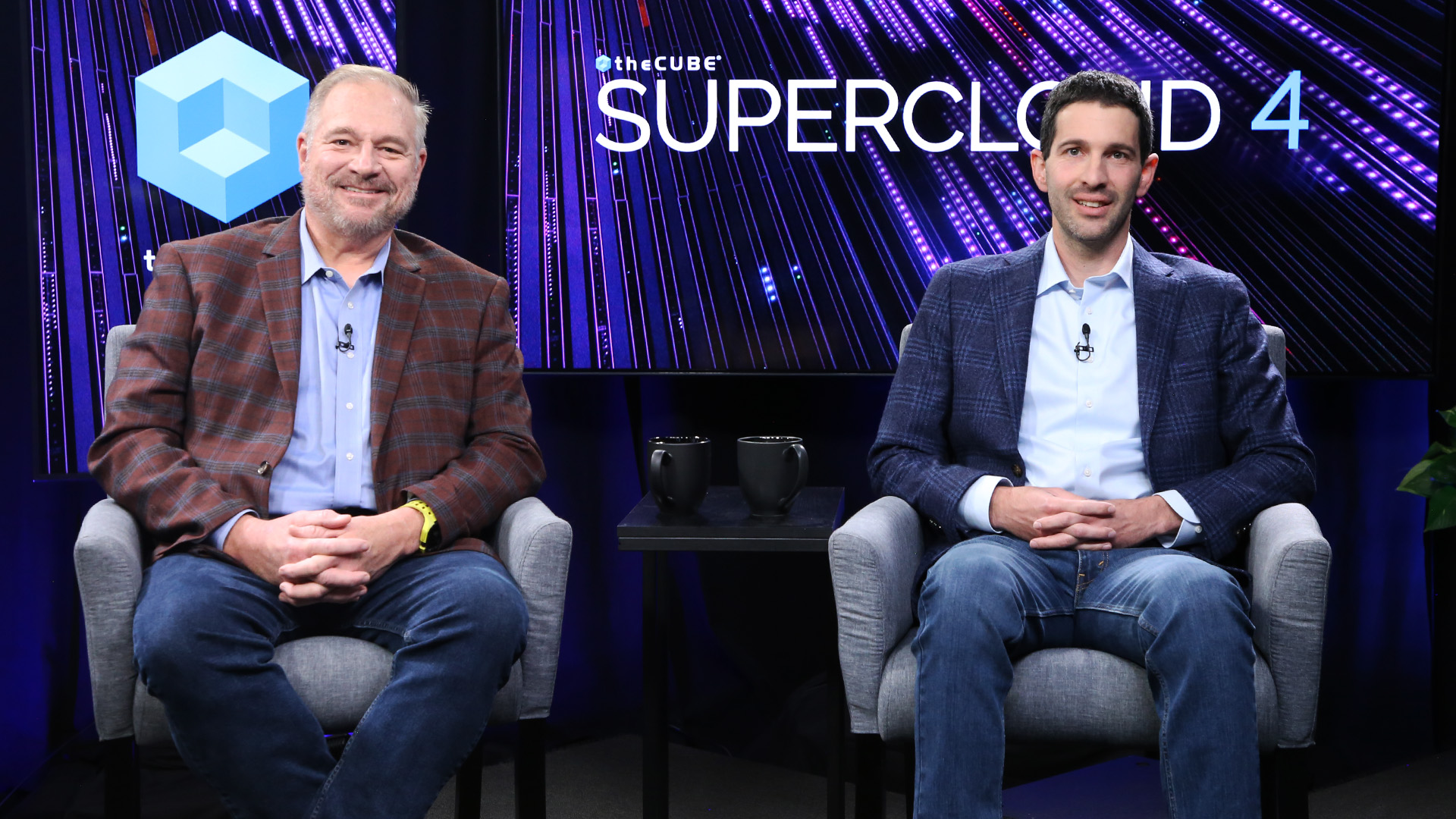
The future of computing is moving toward a ubiquitous model, where workloads and data can be run and leveraged everywhere, including in the cloud, on-premises and at the edge.
Most companies have adopted the cloud because of factors such as cost, agility, flexibility and scalability. Still, only about 20% of customers are fully employing it, as noted by theCUBE industry analyst Dave Vellante. In the hybrid world, however, people are shifting from extreme-cloud or no-cloud approaches to a more balanced approach, according to David Linthicum (pictured, left), chief cloud strategy officer at Deloitte Consulting.
Linthicum wrote the book “An Insider’s Guide to Cloud Computing” to reveal the secrets and details behind cloud computing, including what works and what doesn’t, how to make investments, leverage technology and build a supercloud to save money and mitigate complexity.
“A lot of my clients were telling me that they really need to understand what the story is behind the story. In other words, what are the secrets behind cloud computing,” Linthicum said. “What works and what doesn’t? And they seem to be getting a lot of that detail from communicating with lots of industry insiders, and I just figured I’d write a book on an insider’s guide to cloud computing.”
Linthicum and Lior Gavish (right), co-founder and chief technology officer of Monte Carlo Data Inc., spoke with theCUBE industry analysts Dave Vellante and Rob Strechay at Supercloud 4, during an exclusive broadcast on theCUBE, SiliconANGLE Media’s livestreaming studio. They discussed how companies are utilizing a combination of different to optimize scalability and value.
Workloads and data sets should be distributed across the cloud, on-prem and at the edge, according to Linthicum. Meanwhile, the focus should be on leveraging the platform that offers the most optimization, scalability and value.
This is where artificial intelligence comes in as a game-changer since it accelerates the ability to understand the health and reliability of data systems, particularly when enterprise data is integrated with generative AI models, Gavish explained. Enterprises need to augment AI with its internal data through a retrieval augmentation generation architecture or fine-tune models to make them more knowledgeable about specific domains.
“These systems are pretty complex, and whether you fine-tune or use RAG [Retrieval Augmentation Generation], you’re building a lot of data pipelines that are feeding information from data that’s found across the enterprise and into the customer-facing application,” Gavish said. “And Monte Carlo is there for our customers to help them make those data pipelines work and work reliably and be trusted.”
Companies that rely on individual tools, such as robotic process automation, may have to explore alternative options as emerging technologies, such as generative AI, that can now carry out similar tasks. This raises intricate considerations regarding how organizations should manage its processes and upskill its workforce.
Gen AI is making coding and other tasks easier, but there is a lack of experienced individuals in this field, presenting both a challenge and an opportunity for learning and adaptation, according to Gavish. The next generation of AI models will unlock unstructured data for enterprises, allowing them to analyze and use textual data, images, videos and voice in new ways, requiring the acquisition of new skill sets. Enterprises typically form small teams to brainstorm and prototype various ideas for using generative AI, which are then handed off to other teams for implementation.
“We all have to learn and adapt it to our own lives,” Gavish said. “In some of the early use cases that we’ve seen extremely successful around coding or content creation, we’ve seen a bunch of success with that. There’s a lot more coming, and I think it’s the tip of the iceberg. We’ve seen mostly foundation models doing very basic manipulation of public information.”
Specialized AI may not need to be stored in the cloud unless there is a financial or optimization benefit, as the price of the hardware in data centers has significantly decreased in the last decade. AI can be effectively run on-premises, in the cloud or at the edge, and it’s important to leverage its capabilities wherever it exists, according to Linthicum.
“The ability to put something within a robot system because we were looking for the AI that’s closest to where the data’s going to get gathered, that’s an intelligent edge-based system,” he said. “That may be the benefit of the platform moving forward. Just like we said a while ago, we’re moving to this ubiquitous computing model where everything’s not going to be in one platform.”
Here’s the complete video interview, part of SiliconANGLE’s and theCUBE’s coverage of Supercloud 4:
THANK YOU