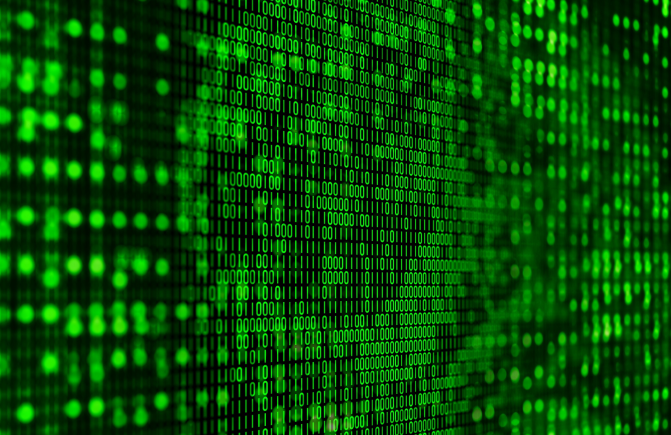
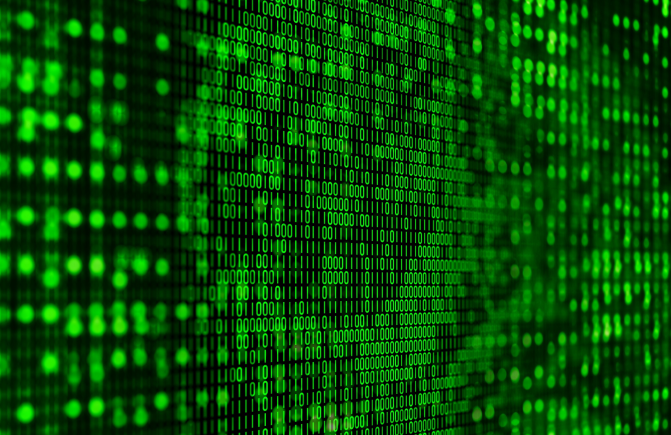
Datology AI, a data curation startup that aims to make it easier to build the enormous training datasets required by generative artificial intelligence models, said today it has closed on an $11.65 million seed funding round.
The round was led by Amplify Partners and saw participation from Radical Ventures, Conviction Capital, Outset Capital and Quiet Capital. Also joining were angel investors such as Google LLC Chief Scientist Jeff Dean, Meta Platforms Inc. Chief AI Scientist Yann LeCun, Quora Inc. founder and OpenAI board member Adam D’Angelo, Cohere Inc. co-founders Aidan Gomez and Ivan Zhang, and ex-Intel Corp. AI Vice President Naveen Rao.
It’s an impressive list of backers, and DatologyAI is aiming to solve one of the biggest challenges in generative AI development today. In a blog post today, Datology AI founder and Chief Executive Ari Morcos explained that the startup provides the tooling needed to automate the curation of datasets that are used to train large language models such as ChatGPT and Google’s Gemini.
It works by identifying which information within a dataset is most important for the model, depending on its application. It can also suggest ways to augment datasets with additional information and work out how it can be batched, or split into more manageable chunks to streamline the model training process.
Curating datasets is a big problem because generative AI models are known to show certain biases that result from prejudicial patterns within their training datasets, but these patterns are difficult for humans to spot. Training datasets are extremely big too, made up of numerous data formats that may contain lots of noise and unnecessary information. In a recent survey by Deloitte Touche Tohmatsu Ltd., 40% of companies said data-related challenges such as preparing and cleaning data remain one of the major headaches when it comes to developing AI models.
Morcos is perhaps the best person to tackle this challenge, as he spent more than five years working at Meta’s AI lab. There he developed neurology-inspired techniques to improve the capabilities of that company’s AI models that mostly involved tinkering with their underlying training data.
According to Morcos, today’s generative AI models are a reflection of the data on which they’re trained, meaning that essentially, “models are what they eat.”
He pointed out that training AI on the right data and doing it in the right way can have a dramatic impact on the overall quality of the model. That’s because training datasets impact almost every aspect of the resulting model, including its performance, its overall size and the depth of its domain knowledge. By having a more efficient training dataset, it’s possible to cut down on training times significantly and create a much smaller model, saving on time and computing costs.
The last point is relevant because some companies are spending millions of dollars on computing resources in order to train and run their AI models. Some of those companies have accumulated petabytes of data – so much that it’s impossible to know where to begin. As a result, it has become standard practice simply to select a random subset of data, Morcos said.
But selecting data at random is problematic because it means the models are being trained on lots of redundant data, which slows down training and increases costs. Moreover, some types of data may actually be misleading and harm the model’s performance, while other training datasets may be unbalanced with “long tails,” which can lead to biases in the resulting AI model.
“The bottom line is: training on the wrong data leads to worse models which are more expensive to train,” Morcos said. “And yet it remains standard practice.”
DatologyAI aims to fix this by helping companies identify the right information to compile their datasets, and present this data in the right way. It’s especially useful for companies faced with petabytes of unlabelled data, which has to be labeled manually.
“Our vision at DatologyAI is to make the data side of AI easy, efficient and automatic, reducing the barriers to model training and enabling everyone to make use of this transformative technology on their own data,” Morcos explained.
The startup claims it can curate petabytes of data in almost any kind of format, be it text, video, images, audio, tabular, genomic or geospatial, and deploy the datasets it compiles on their AI training infrastructure. That’s different from existing data preparation tools, which are often more limited in their scope and the kinds of data they support.
In addition, DatologyAI says, it can also identify the most complex concepts within each dataset and ensure that higher-quality samples are used. It can also spot types of data that might be harmful and cause models to behave differently to what the designer expects.
It isn’t the first startup to tackle the problem of training data, and previous efforts at automation haven’t always worked out as intended. As an example, a German nonprofit AI research group called Large-scale Artificial Intelligence Open Network recently had to take down one of its algorithmically curated datasets after images of child abuse were found within it.
It’s for this reason that DatologyAI doesn’t intend to automate every aspect of dataset curation completely, but rather assist data scientists by suggesting ways in which they can trim their existing datasets. “[Our approach] leads to models that train dramatically faster while simultaneously increasing performance on downstream tasks.”
DatologyAI said it’s currently working with a limited number of customers in order to refine its data curation tools, ahead of a broader release of its platform planned for later this year.
THANK YOU