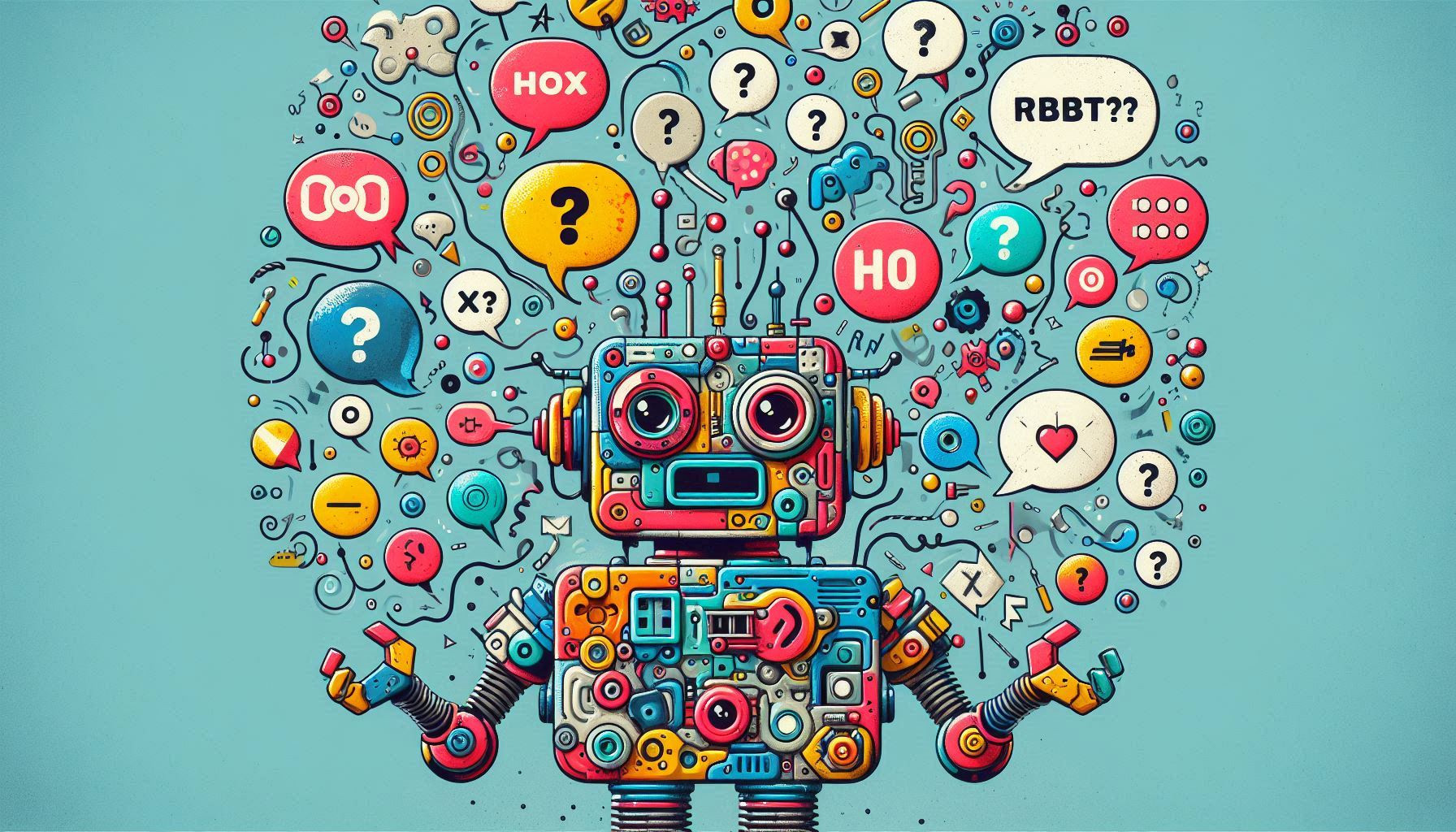
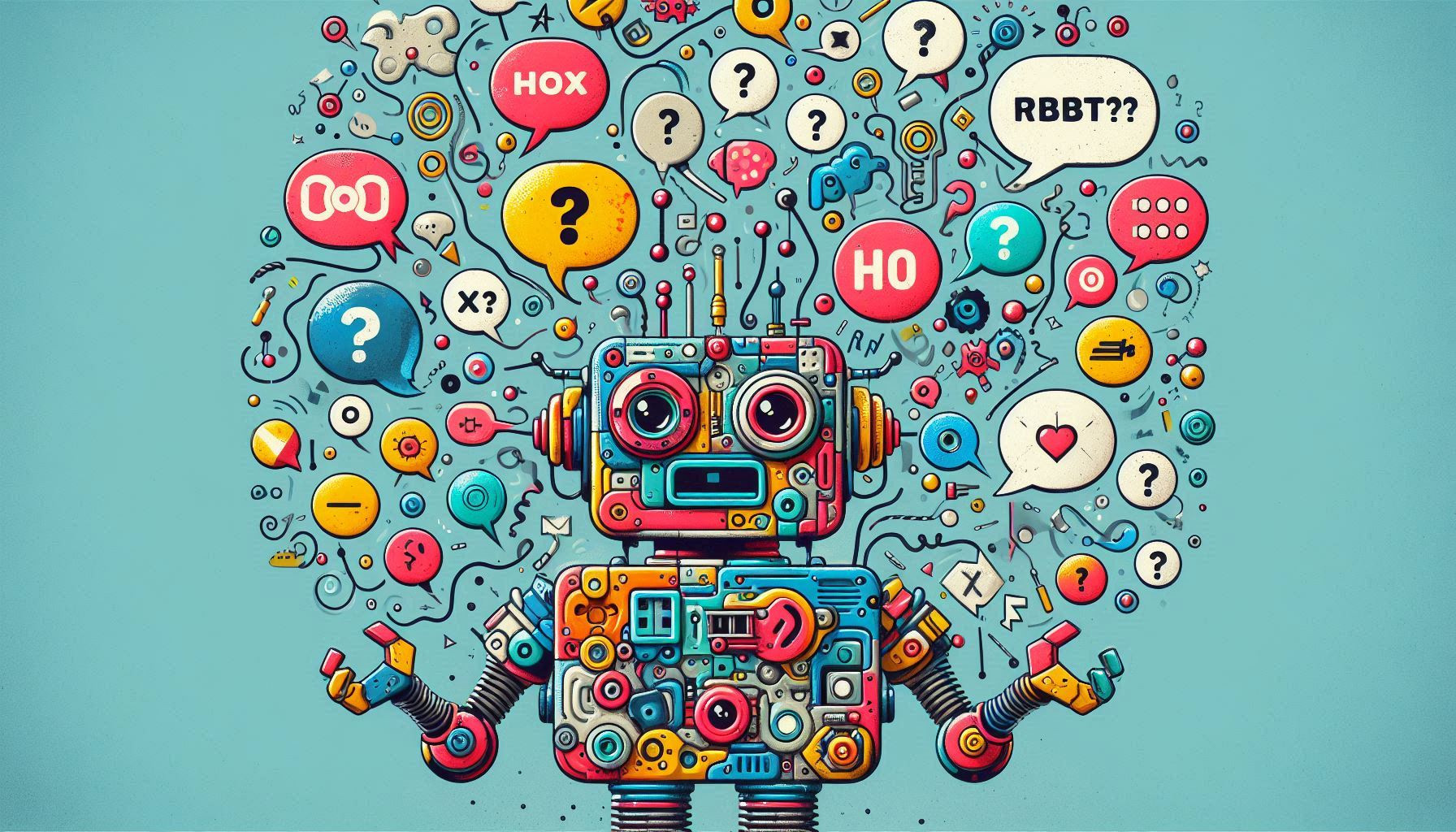
Generative artificial intelligence startup Sierra Technologies Inc. is taking it upon itself to “advance the frontiers of conversational AI agents” with a new benchmark test that evaluates the performance of AI agents in real-world settings.
Compared with previous benchmarks, Sierra’s 𝜏-bench goes further than simply assessing the conversational capabilities of AI chatbots, measuring their ability to complete various complex tasks on behalf of human customer service agents.
Sierra AI was co-founded by former Salesforce Inc. Chief Executive Bret Taylor and ex-Google LLC exec Clay Bavor, and has built what it claims are much more advanced AI chatbots with contextual awareness that enhances their ability to respond to customer’s queries.
Unlike ChatGPT and other chatbots, Sierra’s AI agents can perform actions such as opening a ticket for a customer that wants to return an item and get a refund. It enables customers to complete certain tasks through company chatbots in a self-service way, meaning they never need to talk to a human.
The startup says that a better benchmark is needed to measure the capabilities of these more advanced chatbots, especially as it’s not the only AI company trying to make inroads in this area. Earlier this week for example, a rival company called Decagon AI Inc. announced it had raised $35 million to advance its own AI agents, which can also engage in more contextualized, conversational interactions with customers and take actions where necessary.
“A robust measurement of agent performance and reliability is critical to their successful deployment,” Sierra’s head of research Karthik Narasimhan wrote in a blog post. “Before companies deploy an AI agent, they need to measure how well it is working in as realistic a scenario as possible.”
According to Narasimhan, existing benchmarks fall short of doing this, as they only evaluate a single round of human-agent interaction, in which all of the necessary information to perform a task is exchanged in one go. Of course, this doesn’t happen in real-life scenarios, as agent’s interactions are more conversational, and the information they need is acquired through multiple exchanges.
In addition, existing benchmarks are mostly focused on evaluation only, and do not measure reliability or adaptability, Narasimhan said.
Sierra’s 𝜏-bench, outlined in a research paper, is designed to go much deeper, and it does this by distilling the requirements for a realistic agent benchmark into three key points.
Narasimhan explained that real-world settings require agents to interact with both humans and application programming interfaces for long durations, so as to gather all of the information needed to solve complex problems. Second, AI agents must be able to follow complex policies and rules specific to the task or domain, and third, they must maintain consistency at scale across millions of interactions.
Each of the tasks in the 𝜏-bench benchmark is designed to test an AI agent’s ability to follow rules, reason and remember information over long and complicated contexts, as well as its ability to communicate effectively in these conversations.
“We used a stateful evaluation scheme that compares the database state after each task completion with the expected outcome, allowing us to objectively measure the agent’s decision-making,” Narasimhan explained.
Sierra put a number of popular large language models through its benchmark, and the results suggest that most AI companies still have a long way to go in terms of creating useful chatbots that can actually assist customer service agents beyond simply summarizing a conversation. It found that every one of the 12 LLMs it tested struggled at solving the various tasks in 𝜏-bench. The best performer, OpenAI’s GPT-4o, achieved a success rate of less than 50% across two domains, retail and airline.
The reliability of the 12 LLMs was also extremely questionable, according to the 𝜏-bench test results. Sierra found that none of the LLMs could consistently solve the same task when the interaction was simulated multiple times. The simulations involved slight variations in utterances while keeping the underlying semantics the same. For instance, the reliability of the AI agent powered by GPT-4o was rated at less than 25%, meaning it has just a 25% chance of being able to resolve a customer’s problem without handing off to a human agent.
The results also showed that the LLMs are not particularly great when it comes to following the complex policies and rules set out in its policy documents.
On the other hand, Sierra said its own agents performed much better, because they have a much broader set of capabilities. For instance, the Sierra Agent’s software development kit allows developers to declaratively specify the agent’s behavior, so they can be orchestrated to fulfill complex tasks more accurately. In addition, its agents are also governed by supervisory LLMs that ensure consistency and predictability when dealing with different dialogues that outline the same problems. Finally, Sierra provides agent development lifecycle tools that enable developers to iterate on their agents on the fly, so as to improve their performance based on real-world observations.
Going forward, Sierra said, it will make 𝜏-bench available to the AI community, so anyone can use it to aid in the development of their own conversational LLMs. Its own developers will use 𝜏-bench as a guide when they compile and fine-tune future AI models to ensure they can perform an ever-increasing range of complex tasks with high consistency.
The startup also wants to improve 𝜏-bench by enhancing the fidelity of its simulated humans, leveraging more advanced LLMs with improved reasoning and planning. It will also make efforts to reduce the difficult of annotation through automation, and develop more fine-grained metrics that can test other aspects of AI agents’ conversational performance.
THANK YOU