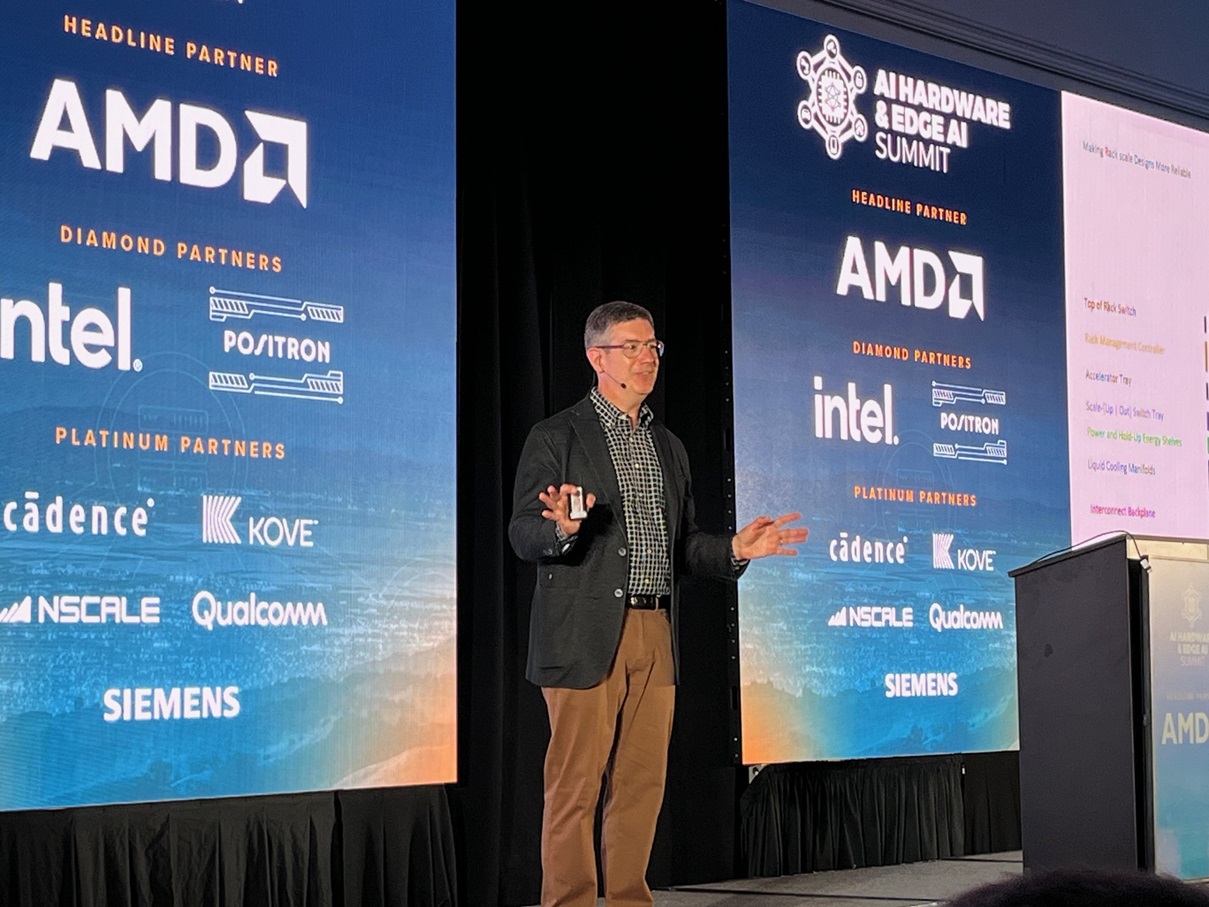
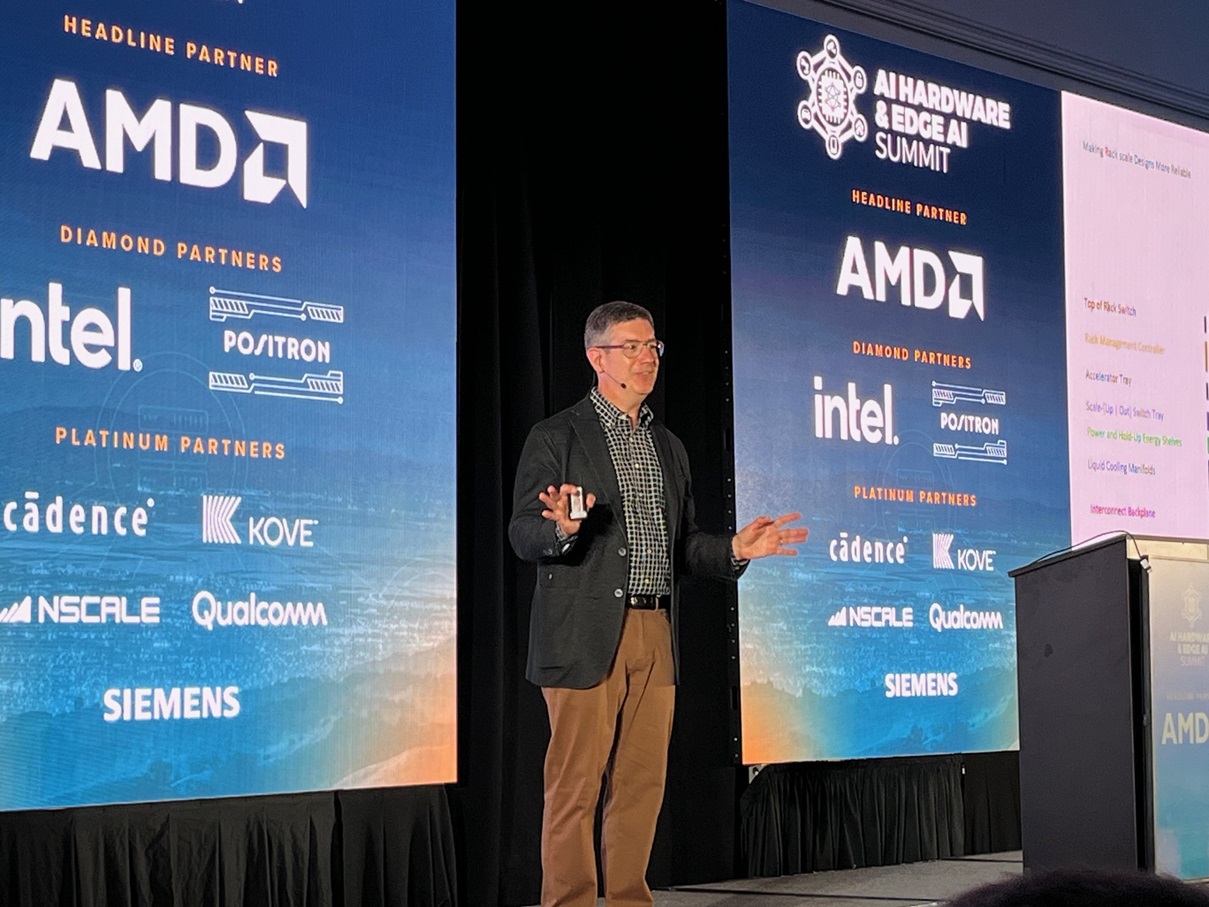
While much of the attention surrounding the growth of artificial intelligence has centered on software development and building models, the engine driving AI is still hardware in the form of compute, storage and networking.
Increasingly, the hardware engine has not always run smoothly as technologists grapple with the need to process massive amounts of data at scale as rapidly as possible. “We are seeing technology plateaus at every stage,” said Partha Ranganathan, vice president and engineering fellow at Google LLC. “We need new innovation to think outside the hardware box.”
Raganathan delivered his remarks during a keynote presentation on Tuesday at the AI Hardware and Edge AI Summit in San Jose, California, an annual gathering where key players in the hardware community assessed the progress, or lack thereof, in tools and systems required for performance-optimized AI. The challenge confronting many of the companies tasked with building out infrastructure to support generative AI is that a combination of speed and complexity is leading to roadblocks along the way.
“This journey is accelerating pretty rapidly,” Dan Rabinovitsj (pictured), vice president of infrastructure at Meta Platforms Inc., said during a presentation at the conference on Wednesday. “The overall infrastructure that’s required to build all of this is becoming more and more complex. And as things get more complex, they break.”
One area of concern involves graphics processing units or GPUs, the specialized processors that have become central to the growth of generative AI applications and workloads. Enterprises are loading up on GPUs to power AI adoption, with chipmaker Nvidia Corp. becoming the prime supplier. Nvidia announced GPU sales growth of 409% earlier this year and reported 122% growth in quarterly revenue and earnings last month.
The problem is that businesses are encountering failure issues when GPUs are clustered together to process AI workloads. One report issued by Meta in July documented hundreds of GPU-related interruptions during a 54-day Llama 3 model training run.
“Things don’t scale linearly,” Rabinovitsj told the conference audience. “As we’ve added GPUs, any interruption starts to slow things down. How much work can I get out of cluster given all the interruptions that are happening? This has been so challenging for AI.”
One solution has been to increase the level of server or cluster testing before running a training job. Meta has implemented this testing in a quest to eliminate bugs that could propagate during the training of AI models.
The goal, according to Rabinovitsj, has been to reduce silent data corruptions or SDCs. These are usually data errors that go undetected and can become a widespread problem that impact computational integrity for large-scale infrastructure systems and applications.
“You might propagate a corrupt piece of data all the way through your training run,” explained Rabinovitsj, who indicated that Meta planned to release news on SDCs in October. “We’re super-careful about this stuff, as everyone should be. It has a significantly high impact on inference.”
Chipmakers are also working to pack even greater performance into processors used for AI. An area of focus for Advanced Micro Devices Inc. has been endpoint AI where personal computers are undergoing a transformation, moving away from traditional architectures and toward intelligence-optimized systems.
AMD debuted its Ryzen 9 9950X laptop processor in June, optimized to run edge-based AI workloads. “The PC is getting completely reimagined,” Vamsi Boppana, senior vice president of AI at AMD, said during a conference keynote on Wednesday. “The needs we have seen in this space are for significantly more dedicated AI compute.”
Chipmakers are also seeking to build technology that will facilitate robust communications between GPUs and networks. Executives at Broadcom Inc. have emphasized the importance of distributed computing solutions to drive AI, and Ethernet is the company’s solution of choice to address unique requirements for high bandwidth, intermittent data surges and massive traffic demands for bulk data transfers.
“What is going to connect all of these GPUs together?” asked Hasan Siraj, head of software and AI infrastructure products at Broadcom. “That is the network. We are dealing with one of the biggest distributed computing problems in the industry.”
Along with technical challenges that the hardware industry has been forced to confront, practitioners must also address the environmental impact of power-hungry GPU clusters and AI-optimized servers that span the globe. One presenting company during the AI Hardware event was the GPU cloud provider Nscale, whose business model was built on providing a sustainable solution that leverages renewable energy.
Nscale Chief Operating Officer Karl Havard predicted that transparency on the part of AI cloud providers around security, resilience, sustainability and compliance will become more significant.
“The performance conversation will change; transparency will be key,” Harvard said. “When you move up the stack to power AI, 100% renewable energy is now key.”
Surrounding the conversation at the AI Hardware Summit this week was a sense of optimism that the promise of AI is real and not another “bubble” of tech popularity that some have recently predicted. The difference this time, according to Thomas Sohmers, founder and CEO of Positron AI Inc., is that the integration of AI into the world’s economic structure has opened innovation beyond the largest technology players. AI has ushered in a new era of limitless labor, according to Sohmers, and the use cases are real.
“Nvidia is selling every GPU they make because people have actual applications they want to run,” Sohmers told attendees at the AI Hardware Summit. “This is not a bubble; it’s the leading indicator of the exponential potential of ‘free labor.’ Business will very soon have the ability to spin up 100,000 expert employees on demand. It can’t be just the biggest and wealthiest companies who can do this.”
THANK YOU