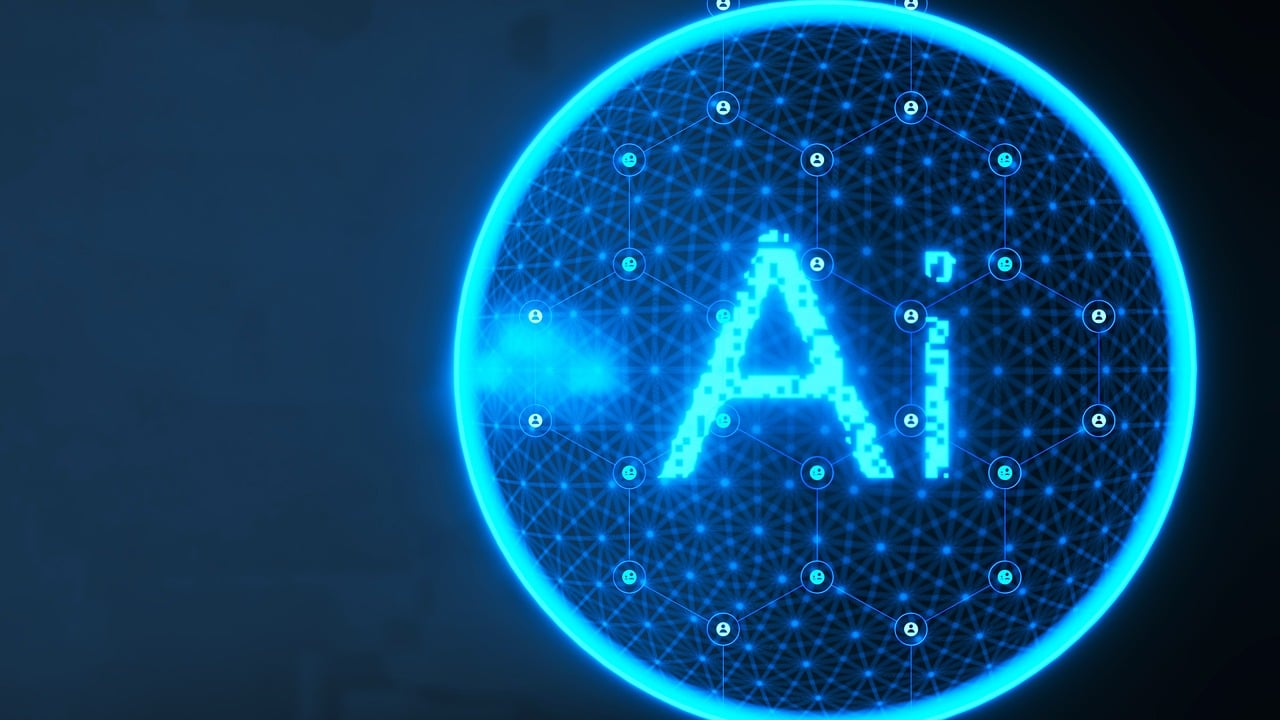
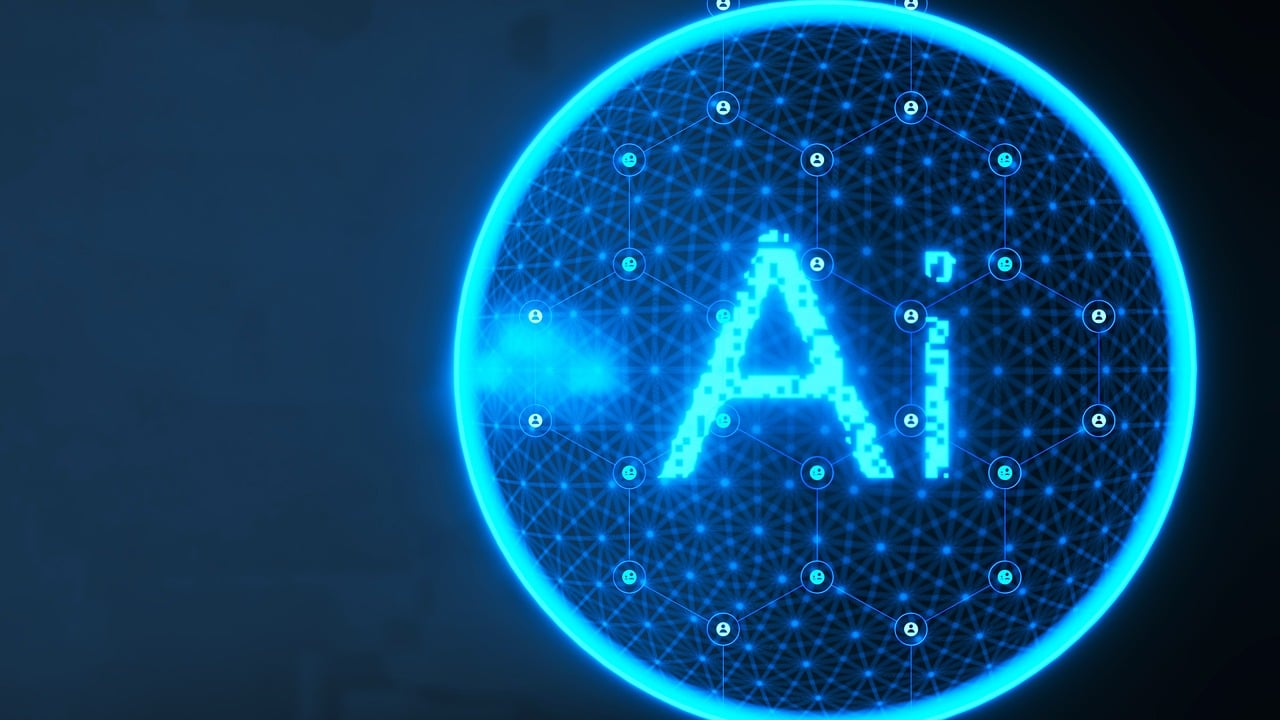
A recent study published by Nature says newer, bigger versions of the three major artificial intelligence chatbots may be more likely to generate wrong answers than claim that they don’t know.
Although more refined and bigger large language models that use more data and more complex reasoning and fine-tuning proved to be better at giving more accurate responses, they also had another problem: They answered more questions overall.
“They are answering almost everything these days,” José Hernández-Orallo at the Valencian Research Institute for Artificial Intelligence in Spain said about the phenomenon. “And that means more correct, but also more incorrect answers.”
The assessment also discovered that people who use chatbots aren’t very good at spotting bad answers, in part because of how well the chatbot creates an answer that looks like a truthful one. Hernández-Orallo added that the result is that users often overestimate the capabilities of chatbots and that’s a problem.
The action of an LLM producing an answer that looks truthful, but isn’t has an amusing term: “bullshit.” It was proposed by Mike Hicks, a philosopher of science and technology at the University and technology at the University of Glasgow U.K.
“That looks to me like what we would call bullshitting,” said Hicks. “It’s getting better at pretending to be knowledgeable.”
He suggested this term instead of the industry standard “hallucinations,” where an LLM produces a confident but completely incorrect answer. Although these errors can represent between 3% and 10% of responses to queries, there are ways to mitigate them by adding guardrails to expert LLMs to ground them with more accurate information.
However, it’s more difficult with generalized AI models that train with vast datasets. The problem can be even more prevalent when training data comes from the web, which can include AI-generated sources, leading to even more hallucinations.
The research team examined three LLM families, including OpenAI’s GPT, Meta Platform Inc.’s Llama and BigScience’s open-source model BLOOM. To test them, the researchers tested thousands of prompts using questions on arithmetic, anagrams, geography, science and the models’ ability to transform information.
Although accuracy increased as models became larger and decreased as questions became harder, researchers hoped that models would avoid answering questions that were too difficult. Instead, models such as GPT-4 answered almost everything.
Equally at issue, people asked to rank answers as correct, incorrect or avoidant tended to classify inaccurate answers as accurate a little too often. Between easy questions, 10% got it wrong and with difficult questions, 40% got it wrong.
To deal with the issue, Hernández-Orallo said, developers need to adjust models to handle hallucinations on easy questions to refine accuracy and simply decline to answer hard questions. This may be what’s needed to allow people to get a better understanding of where the AI model can be trusted to be consistent and accurate.
“We need humans to understand: ‘I can use it in this area, and I shouldn’t use it in that area,’” Hernández-Orallo said.
THANK YOU