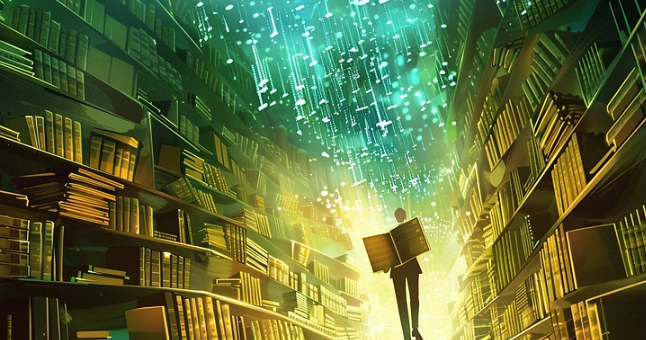
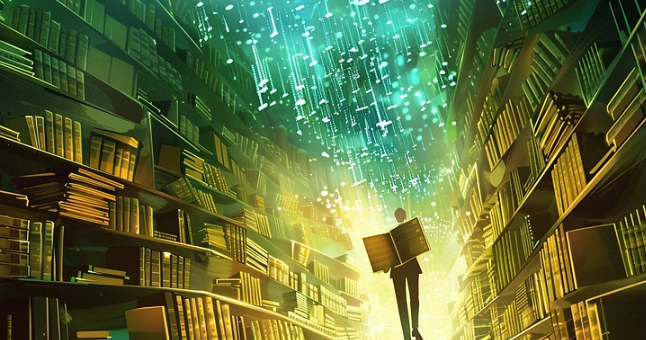
Data integration startup Vectorize AI Inc. says its software is ready to play a critical role in the world of artificial intelligence after closing on a $3.6 million seed funding round today.
The round was led by True Ventures, and was announced alongside the debut of its novel platform that’s meant to aid in retrieval-augmented generation or RAG.
The startup is aiming to tackle a problem it has identified among AI practitioners, namely the challenge of taking various bits and bytes of unstructured data like written documents, video, audio files and so on, and transforming these so they can be fitted neatly into a vector database and optimized for RAG.
RAG, or retrieval-augmented generation, is a technique that’s used to provide generative AI models with real-time access to the most relevant and up-to-date information, which is required to make better decisions. One of the problems with AI chatbots such as OpenAI’s ChatGPT is that they’re trained on much older information. For instance, the GPT-3.5 model that powered ChatGPT when it launched a couple of years ago was trained on basically the entire internet as it was in 2022. So it doesn’t have access to any recent news beyond that date.
By using RAG techniques, it’s possible to connect AI models to proprietary datasets and enhance their knowledge with the most recent information. To do this, teams generally rely on a vector database such as Pinecone, DataStax, Couchbase or Elastic, which stores unstructured data as vector embeddings that can be accessed and understood by AI models.
What Vectorize does is connect these vector databases to live, unstructured data sources such as an internal knowledge base, collaboration tool or customer relationship management platform. It’s an important capability because managing and vectorizing unstructured information is a major headache for data scientists.
At the heart of Vectorize’s platform is a “production-ready RAG pipeline” that makes it possible to transform unstructured data into optimized vector search indexes. Using this, companies can feed their most relevant new information into the large language models they are using to power their AI applications.
To simplify this task, Vectorize has devised an intuitive three-step process for transforming data. The first step involves importing data into its platform, which involves feeding it with scanned paper-based documents or connecting it to some kind of computer system. Once it’s connected, Vectorize extracts any natural language content within.
The next step is to evaluate that new data. The platform evaluates multiple chunking and embedding strategies in real time, quantifying the results to find the most optimal configuration. Customers can go with Vectorize’s recommendations or implement their own strategy on how best to vector their new data.
The final step is deployment, which involves creating a real-time vector pipeline to automatically update the AI models and ensure continuous accuracy. By doing this, AI models will always have access to the most current information as the organization’s data evolves.
Vectorize reckons that these three steps can accelerate the data preparation process, reducing the time it takes from weeks or months to just a few hours.
A few things set Vectorize apart from its competitors, such as its self-service model and its pay-as-you-go pricing. Users have the flexibility to import data from almost any source they can think of, and they can test and optimize different approaches to doing this before settling on the most efficient pipeline architecture.
Because the platform is pay-as-you-go, it’s also ready to use almost immediately, with no long enterprise commitments or onboarding processes.
In addition, the flexibility of Vectorize means users can define how frequently they want to update their vector search databases, so they can set it up to constantly update in real time, or just add new information on a weekly or monthly basis.
Another novelty of Vectorize’s platform is its “agentic AI” approach, which combines RAG with AI agents capable of autonomously solving problems for users. For instance, the AI cloud infrastructure company Groq Inc. uses Vectorize to power its AI support agents, which can automatically fix customer’s problems using real-time data and context.
The company offers free access to its platform with enough bandwidth to support smaller projects, while larger enterprises with more data to prepare only need to pay as they go for the information they feed into their vector databases. As such, Vectorize says it’s one of the most cost-effective data preparation tools for RAG on the market.
Nicholas Ward, president of the advertising technology company Koddi Inc. and an angel investor in Vectorize, believes the company’s platform will become a foundational technology for many enterprise AI projects.
“Having worked with Vectorize’s founders in the past, I’ve seen firsthand their ability to tackle complex data challenges,” Ward said. “The RAG platform is set to become a cornerstone technology for companies leveraging AI, from adtech to fintech and beyond.”
THANK YOU