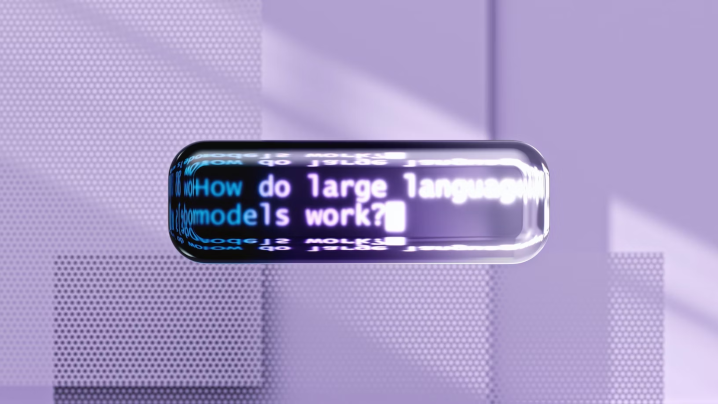
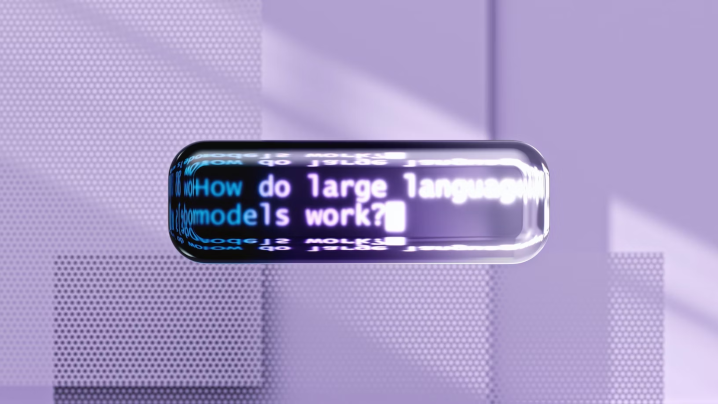
H2O.ai Inc. on Thursday introduced two small language models, Mississippi 2B and Mississippi 0.8B, that are optimized for multimodal tasks such as extracting text from scanned documents.
The models are available on Hugging Face under an open-source license.
Mountain View, California-based H2O.ai provides a suite of tools for building artificial intelligence applications. Enterprises can use the company’s software to identify the open-source language model most suitable for an application project, customize that model and check the accuracy of its output. H2O.ai also provides features for related tasks such as implementing RAG features.
The first multimodal model that the company released this week, Mississippi 2B, features 2.1 billion parameters. It’s designed to analyze images based on natural language instructions provided by the user. Mississippi 2B can generate a high-level description of an image, elaborate on a specific detail highlighted by the user and explain data visualizations.
The model also lends itself to text extraction tasks. A company could, for example, use Mississippi 2B to extract purchase details from a scanned receipt and upload the information to a sales database. The AI can optionally package the extracted text into the JSON file format, which makes it easier to load information into applications.
Mississippi 0.8B, H2O.ai’s other new model, is a scaled-down version of Mississippi 2B with 800,000 parameters. It’s designed for many of the same tasks with a particular emphasis on text extraction. According to H20.ai, the algorithm outperforms all comparable small language models at optical character recognition tasks.
The company compared Mississippi 0.8B against the competition using a benchmark assessment that comprised 300 tasks. The evaluated models had to process logos, handwritten text, digits and other types of content. H20.ai says that its model outperformed not only comparably-sized algorithms but also open-source large language models with more than 20 times as many parameters.
Mississippi 2B and Mississippi 0.8B are based on the same architecture. When the algorithms are given an image to process, they divide it into tiles that measure 448 pixels by 448 pixels. From there, a component known as an encoder turns the tiles into embeddings, mathematical structures that AI models use to hold information. Those embeddings are then analyzed to answer user questions.
H2O.ai trained Mississippi 2B and Mississippi 0.8B in different ways. The former model’s training dataset included 17.2 million sample tasks that each comprised an image, a question about that image and an answer. Mississippi 0.8B, in turn, was developed using 19 million examples.
“We’ve designed H2OVL Mississippi models to be a high-performance yet cost-effective solution, bringing AI-powered OCR, visual understanding and Document AI to businesses,” said H2O.ai founder and Chief Executive Officer Sri Ambati.
H20.ai envisions developers deploying its new AI model series on devices with limited processing power. According to the company, the algorithms are also useful for latency-sensitive use cases. Thanks to their considerably lower parameter counts, small language models can respond to user queries significantly faster than frontier LLMs such as GPT-4o.
THANK YOU