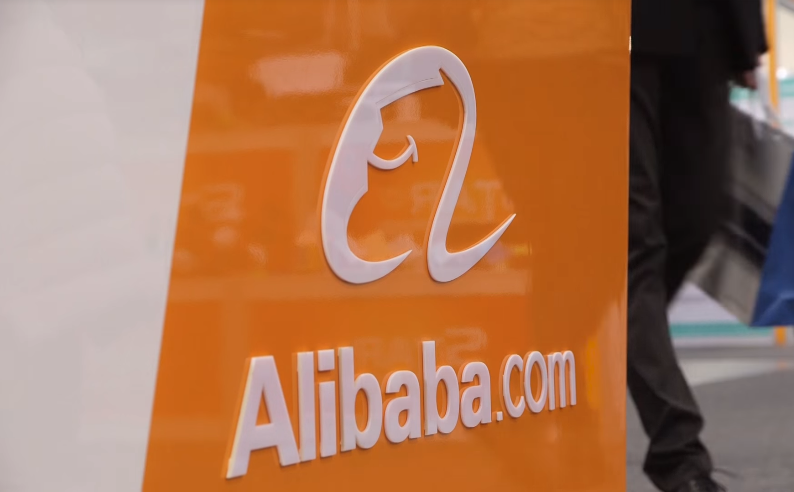
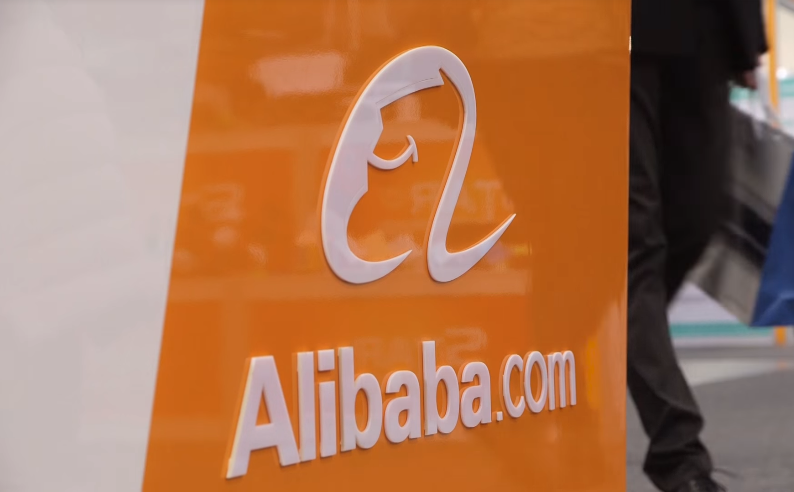
Shares of Alibaba Group Holding Ltd. rose more than 8% today following the company’s release of a reasoning model with performance comparable to DeepSeek-R1.
The new model, QwQ-32B, was open-sourced on Wednesday.
R1 comprises multiple neural networks that together have 671 billion parameters. When the model receives a query, it uses just one of the neural networks to generate an answer. In practice, that means R-1 activates only 37 billion of its 671 billion parameters at any given time. Alibaba’s new QwQ-32B model is considerably smaller: the company says the algorithm has 32.5 billion parameters in total.
QwQ-32B is based on the Transformer architecture that underpins most large language models. Transformer-based LLMs use a machine learning technique called attention to infer the meaning of sentences. Using attention, a neural network can not only consider multiple data points while making a decision also prioritize the most important ones.
For this model, Alibaba made several modifications to the original Transformer architecture. One of the main additions is a feature called rotary positional encoding. The capability allows the LLM to more thoroughly understand relationships between the text snippets it ingest, which helps boost output quality.
It can process prompts with up to tokens with up to 131,072 tokens. One token corresponds to a few characters. Alibaba says that the model is particularly adept at reasoning tasks such as writing code, solving math problems and performing tasks in external applications.
The company developed QwQ-32B using a method called reinforcement learning. In a reinforcement learning project, researchers give an AI model a set of training tasks and entrust a second AI model with checking the answers. When the LLM being trained completes a task correctly, it receives points that help guide the learning process.
Alibaba developed QwQ-32B through two training sessions. The first session focused on teaching the model math and coding skills. To support the learning process, Alibaba set up a server that ran the code QwQ-32B produced during training and checked it for errors.
During the second training session, the company honed QwQ-32B’s general problem-solving capabilities. The second session followed a relatively simple workflow. Nevertheless, it not only improved the model’s problem-solving skills but also enhanced its ability to align output with user instructions.
According to Alibaba, QwQ-32B outperformed R1 across three of the five benchmarks it used to compare the LLMs. The former model achieved the biggest point difference, a 6% edge, in a benchmark that measures LLMs’ ability to interact with external systems. The two other tests that Alibaba used evaluate LLMs’ question-answering skills and ability to align output with user introductions.
The release of QwQ-32B comes days after Alibaba committed to spending 380 billion yuan, or about $53 billion, on AI infrastructure in the next three years. That’s more than what the company invested in AI and its public cloud platform over the past decade.
Other Chinese tech giants are also prioritizing LLM development. Last week, Tencent Holdings Ltd. introduced a “fast-thinking” reasoning model dubbed Hunyuan Turbo S. It answers prompts in under a second and has comparable output quality to DeepSeek-V3, a predecessor of R1.
THANK YOU