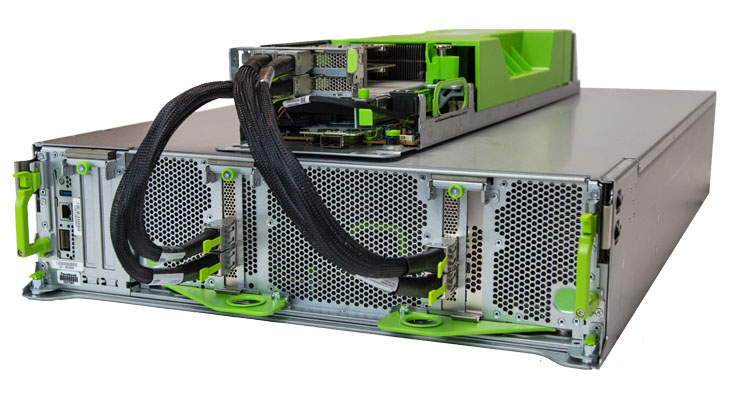
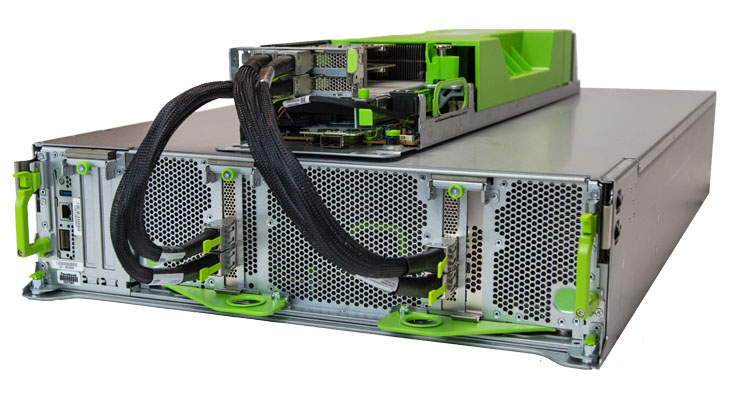
Facebook Inc. has released an updated version of its open-source graphics processing unit-based server design “Big Basin” to the Open Compute Project.
Facebook launched the OCP to help other companies build more efficient server and storage systems for their data centers based on so-called “white-box” hardware, which is cheaper and usually unbranded gear. The initiative has proved to be a big success, with companies including Apple Inc., Google LLC, Microsoft Corp., Intel Corp. and Lenovo Group Ltd. all signing on to the effort.
Big Basin v2 is Facebook’s latest donation to the OCP. It follows the original Big Basin server, upgraded to include eight of Nvidia Corp.’s flagship Tesla V100 graphics cards. To get a little more technical, the server also includes a Tioga Pass central processing unit chip as the head-node, while the PCIe bandwidth that’s used to transfer data between CPUs and GPUs has been doubled.
These improvements give Big Basin v2 a massive 66 percent single-GPU performance increase, Facebook engineers Kevin Lee and Xiaodong Wang wrote in a blog post Tuesday. This improvement should be especially interesting for anyone involved in machine learning, the engineers said, because it means they can build larger models, and train and deploy them more efficiently.
Facebook is already using the Big Basin v2 servers itself for a variety of purposes, including monitoring user interactions to be able to predict the kinds of things they might want to see on their news feed. Besides its news feed, Facebook also use machine learning for things such as personalized advertisements, language translation, search, speech recognition, suggesting tags in images and more.
Lee and Wang provided some detail on how Facebook implements machine learning models, saying it runs them through an artificial intelligence software platform called FBLearner, which is made of three components: Feature Store, Flow and Predictor.
The engineers explained that Feature Store is used to create “features” from data, which are then fed into Flow, which in turn is used to build, train and evaluate machine learning models based on those features.
“The final trained models are then deployed to production via FBLearner Predictor,” the engineers wrote. “Predictor makes inferences or predictions on live traffic. For example, it predicts which stories, posts, or photos someone might care about most.”
Facebook is providing great detail about the hardware and software systems it uses to power its machine learning projects. But Facebook doesn’t offer cloud AI services to enterprise customers, unlike other leaders in the sphere such as Amazon Web Services Inc. and Google LLC, which keep their hardware systems under closer wraps.
“We believe that collaborating in the open helps foster innovation for future designs and will allow us to build more complex AI systems that will ultimately power more immersive Facebook experiences,” the company said.
THANK YOU