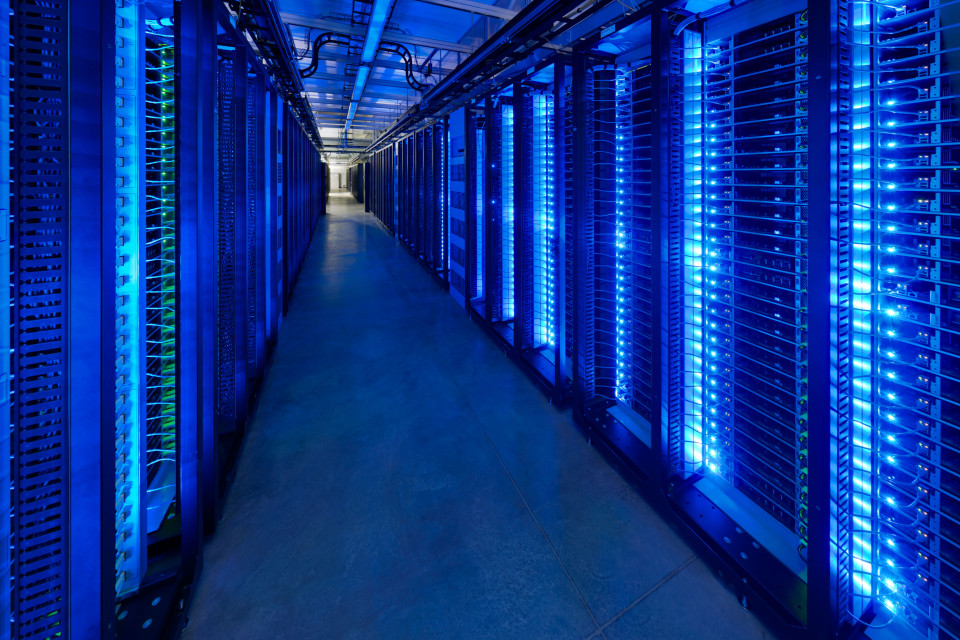
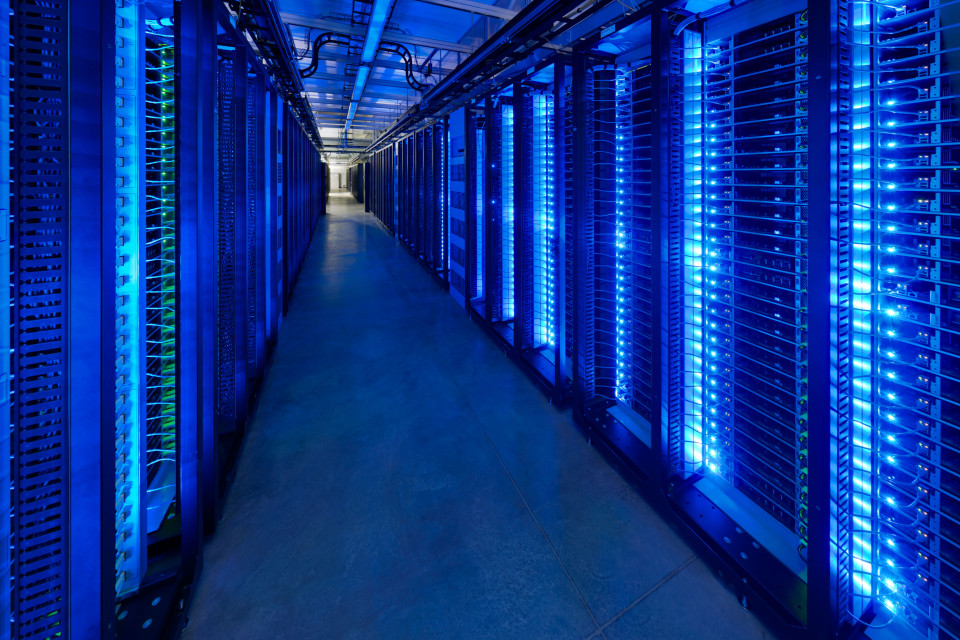
Few organizations use artificial intelligence on the scale that Facebook Inc. does. The social network’s deep learning models perform 200 trillion predictions each day, a level of output made possible by purpose-built hardware designed from the ground up to run neural networks.
At the Open Compute Summit today in San Jose, California, Facebook open-sourced three of the core building blocks that make up its infrastructure. They include a powerful server engineered for the sole purpose of training deep learning models and a pair of similarly specialized, internally designed chips.
The training phase of AI projects is often the most hardware-intensive aspect of the entire development workflow. Before an algorithm can process live data, engineers have to feed it immense quantities of training information to help it learn what patterns to look for and how. In the case of Facebook, the company’s programmers draw upon a repository of over 3.5 billion public images to hone their models.
The AI training server it open-sourced today helps speed up the process. Dubbed Zion, the machine is powered by eight central processing units that each sport a generous amount of so-called DDR memory and can share this memory with one another to coordinate processing.
Administrators may equip Zion with up to eight additional chips optimized for the specific type of AI they’re training in a given project. These accelerators are based on OAM, another technology that Facebook open-sourced today. It’s a hardware standard that semiconductor makers can implement to package different kinds of chips in a common, standardized module.
Zion’s use of OAM makes the server highly versatile. The server can be equipped with a wide range of accelerators, including graphics cards, field-programmable gate arrays and even fully custom processors so long as they all come in the same standardized module.
“Zion decouples memory, compute and network intensive components of the system, allowing each to scale independently,” Facebook engineers Kevin Lee, Vijay Rao and William Christie Arnold wrote. “As our AI training workloads continue to grow in size and complexity, the Zion platform can scale with it.”
This need for scalability is also reflected in the design of Kings Canyon and Mount Shasta, the two chips Facebook unveiled alongside Zion. They’re application-specific integrated circuits that the social network giant optimized at the hardware level for their respective target workloads.
Kings Canyon is built to perform inference, the term for the data processing performed by an AI that has already been trained. Facebook uses it in dedicated “accelerator racks” that each have room for multiple chips and connect to the servers in the company’s data centers over the network.
Mount Shasta, the other custom chip, is optimized for video transcoding. In Facebook’s data centers, the processor handles the task of generating low-resolution versions of the clips uploaded by users to the social network. This makes video content more accessible for members who have slow or unreliable internet connections.
“On average, we expect our video accelerators to be many times more efficient than our current servers,” Facebook’s engineers wrote.
Support our open free content by sharing and engaging with our content and community.
Where Technology Leaders Connect, Share Intelligence & Create Opportunities
SiliconANGLE Media is a recognized leader in digital media innovation serving innovative audiences and brands, bringing together cutting-edge technology, influential content, strategic insights and real-time audience engagement. As the parent company of SiliconANGLE, theCUBE Network, theCUBE Research, CUBE365, theCUBE AI and theCUBE SuperStudios — such as those established in Silicon Valley and the New York Stock Exchange (NYSE) — SiliconANGLE Media operates at the intersection of media, technology, and AI. .
Founded by tech visionaries John Furrier and Dave Vellante, SiliconANGLE Media has built a powerful ecosystem of industry-leading digital media brands, with a reach of 15+ million elite tech professionals. The company’s new, proprietary theCUBE AI Video cloud is breaking ground in audience interaction, leveraging theCUBEai.com neural network to help technology companies make data-driven decisions and stay at the forefront of industry conversations.