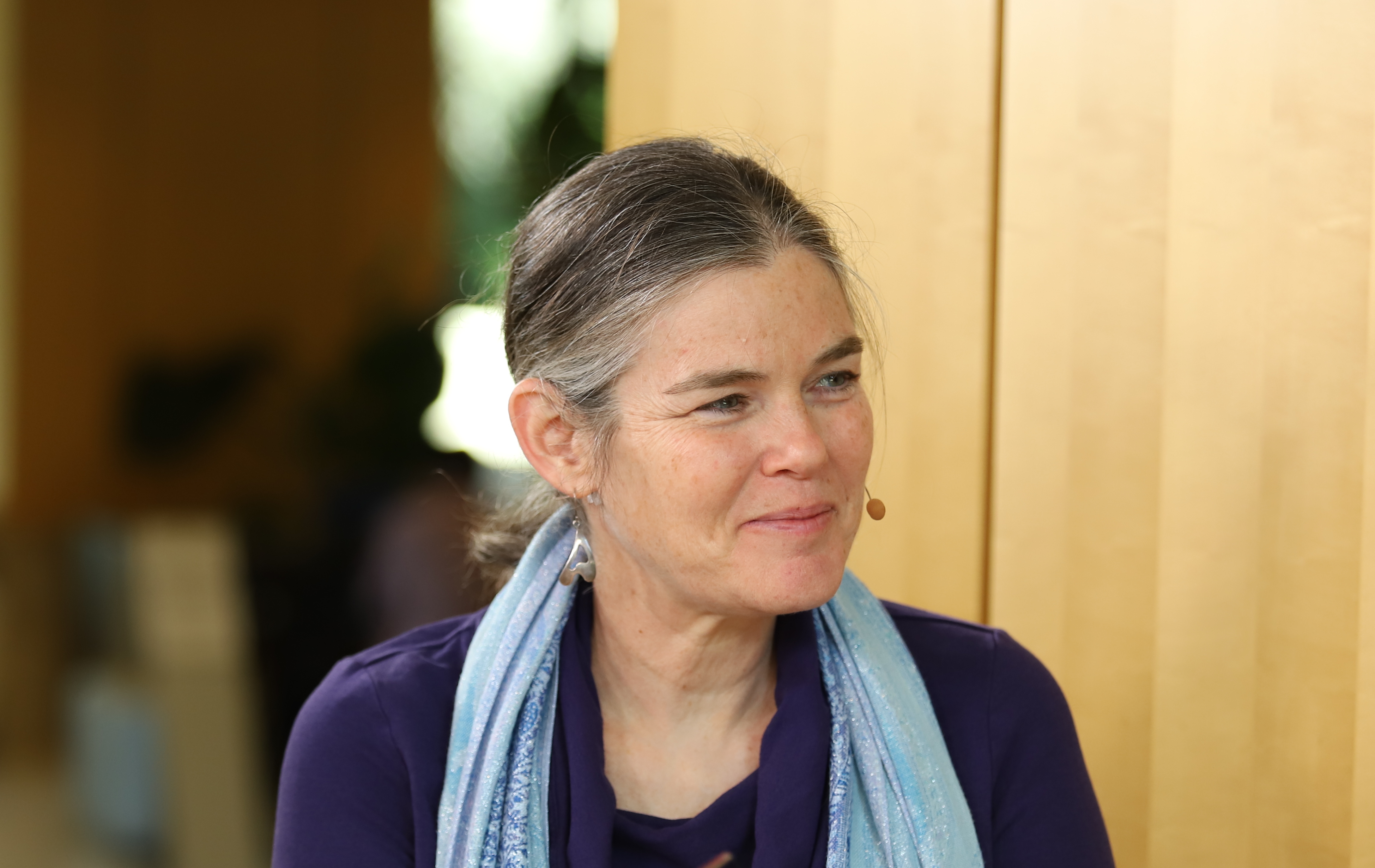
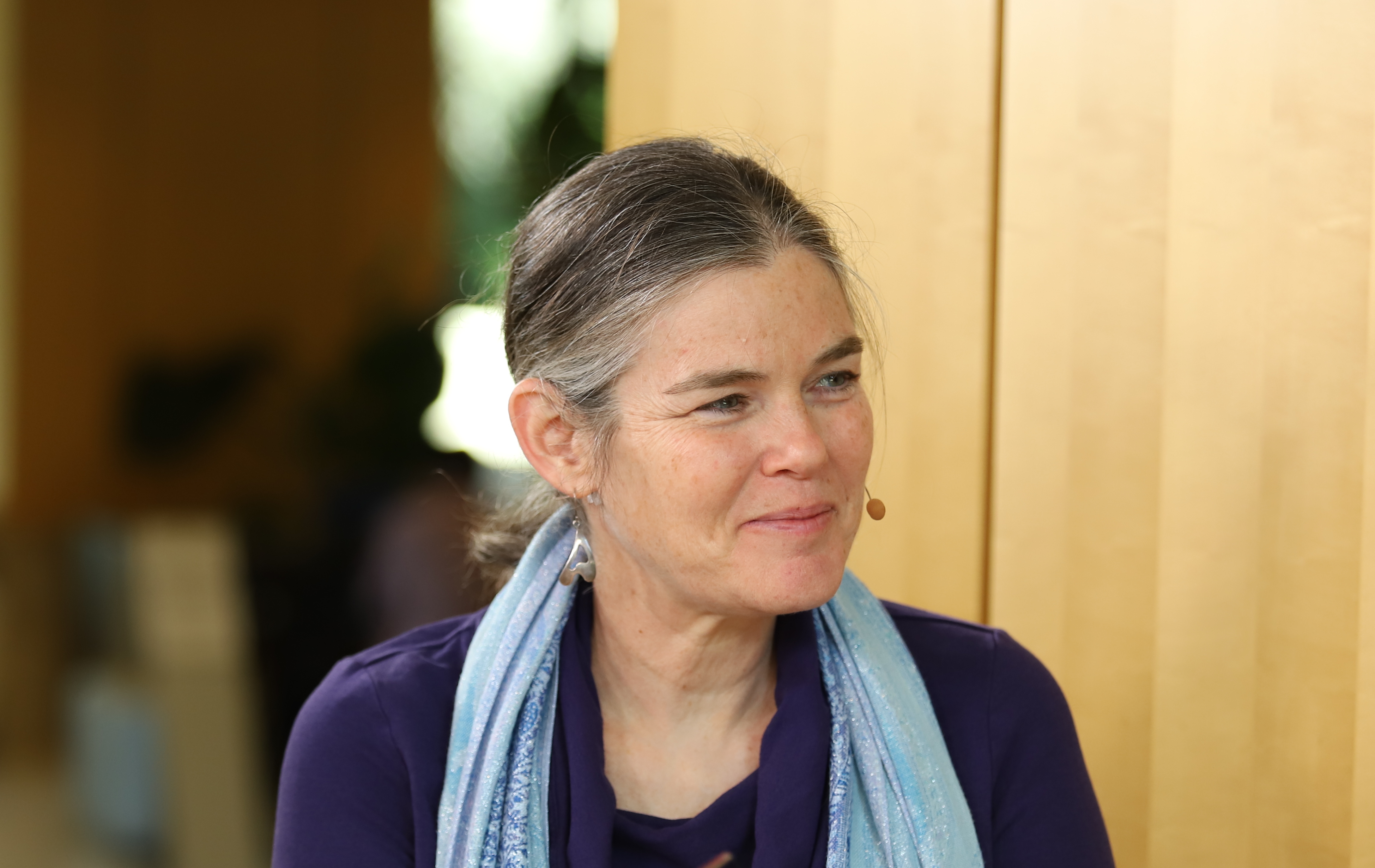
The convergence of previously discrete fields is a hallmark of the digital era. Remember the divide between development and operations teams? That gap vanished into the cloud, as DevOps became the new way of working.
Now technology is becoming incorporated into other disciplines. In the 1990s, quantitative biology took a leap from a descriptive science to gene sequencing, thanks to technology such as microarrays. At the same time, big data was revolutionizing information technology.
“What I think is coming now, 30 years later, is the convergence of those two fields into one field that I like to think of as digital biology,” said Daphne Koller (pictured), founder and chief executive officer of Insitro Inc.
Koller spoke with Sonia Tagare, host of theCUBE, SiliconANGLE Media’s mobile livestreaming studio, during the Women in Data Science conference in Stanford, California. They discussed how applying machine-learning techniques to traditionally biological research fields — such as drug research — could bring down the costs of medicine.
Measuring biology has taken on new levels of detail, fidelity and scale thanks to new technology, according to Koller. Artificial intelligence and machine learning allow scientists to interpret what they are seeing and engineer new solutions that “will have implications in biomaterials, in energy, in the environment, in agriculture, and I think also in human health,” Koller said.
One of the biggest problems in the health field is the negative trend in number of drugs approved versus dollars spent on research. This is known as Eroom’s Law, because it is the opposite of Moore’s Law.
“Despite many important advancements, the costs just keep going up and up and up,” Koller said.
Machine learning could hold the key to breaking this trend, but it requires a cross-discipline approach, according to Koller. “One needs to really build a culture of people who work together from different disciplines, each bringing their own insights and their own ideas into the mix,” she said.
The team she has created at Insitro is half life scientists and half machine learning and data science experts.
“They start from the very beginning to understand what are the problems that one could solve together: How do you design the experiment? How do you build the model? And how do you drive insights that can help us make better medicines for people?” she said.
Using a data-driven approach, collecting and analyzing huge amounts of data will reveal new hypotheses, according to Koller.
“Hopefully, we’ll be able to create enough data and apply machine learning to address key bottlenecks in the drug discovery and development process,” she said. “[Then] we can bring better drugs to people, and we can do it faster and, hopefully, at much lower cost.”
Watch the complete video interview below, and be sure to check out more of SiliconANGLE’s and theCUBE’s coverage of the Women in Data Science conference.
THANK YOU