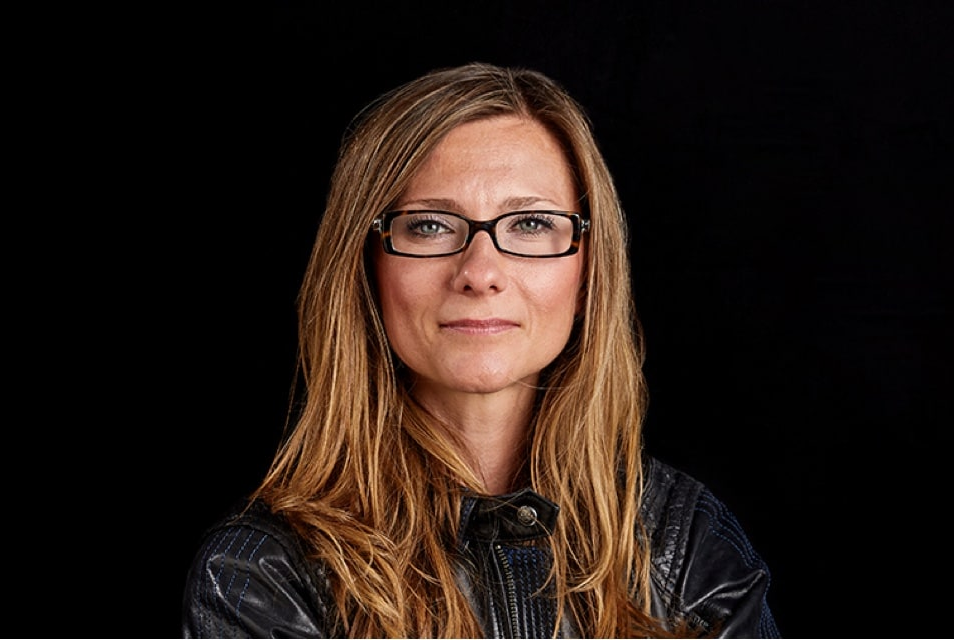
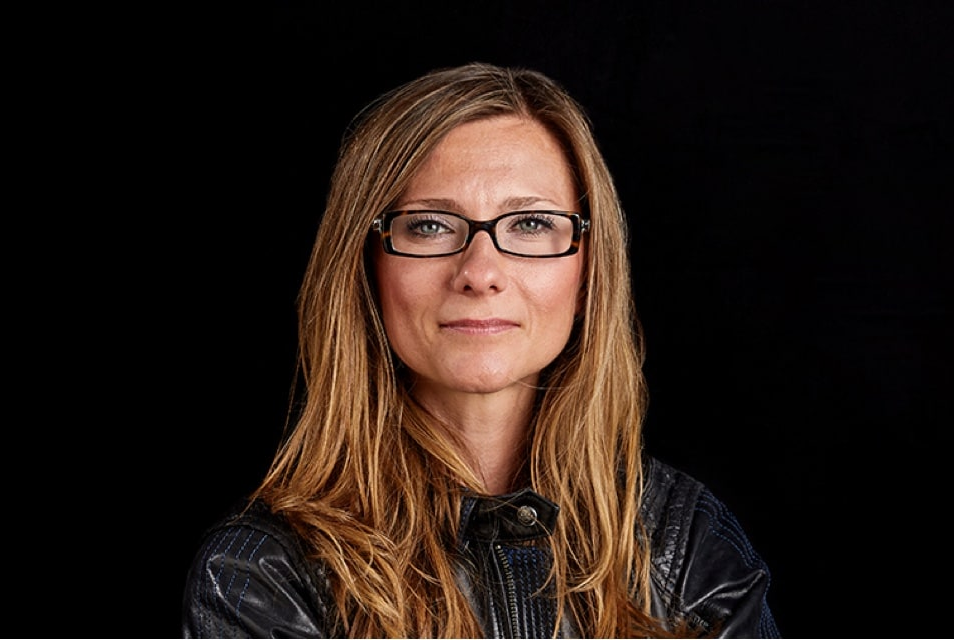
As enterprise data grows, governance is a conversation at the forefront of many organizations seeking to monetize internal data sets. By being able to ensure the quality, integrity and security of data, companies are able to make real-time, critical decisions that impact budgets and help businesses meet other core objectives.
Yet Harley-Davidson Motor Co., like many companies working toward digital transformation, isn’t fully taking advantage of its data. With the goal of strategically using data to inform them on application use, the company is looking to apply a variety of proven technologies and increase operational efficiency. There’s even an opportunity for organizations like Harley-Davidson to use their own data to make a substantial profit by sharing across and beyond their own industry.
“We are on the brink of big data, and it’s only going to get bigger. There are organizations right now that have understood how much of an asset their data really is. They’re starting to sell their data off to other similar people or similar industries. They can make money off of it because data truly is an asset now,” said Victoria Stasiewicz (pictured), global information systems manager at Harley-Davidson. “The key to it was obviously making sure that it’s curated, that it’s cleansed, that it’s trusted, so that when you are selling that back, you can truly make money off of it.”
Stasiewicz spoke with Dave Vellante (@dvellante), host of theCUBE, SiliconANGLE Media’s livestreaming studio, during IBM’s DataOps in Action event. They discussed data governance, virtualization, and the benefits of operationalizing artificial intelligence and machine learning into the data pipeline. (* Disclosure below.)
[Editor’s note: The following answers have been condensed for clarity.]
You’re steeped in a data role at Harley-Davidson. Can you describe a little bit about what you’re doing and what that role is like?
Stasiewicz: What my team is charged with is building out data governance at an enterprise level, as well as supporting the AI and machine-learning technologies. I have a portfolio — that portfolio really includes data in AI and governance, our master data, reference data, and data quality function.
What are the changes that you’ve discerned in data and in your organization over the last, let’s say, half a decade?
Stasiewicz: We started looking at access management and the ability to allow some of our users to do some rapid prototyping that they could never do before. So, what more and more we’re seeing as far as data citizens or data scientists, or even analysts throughout most enterprises, is that they want access to the information [and] they want it now. They want speed to insight at this moment, using pretty much minimal viable product. They may not need the entire data set, and they don’t want to have to go through leaps and bounds to just get access to that information or to bring that information into a centralized location.
What we’re seeing change throughout the industry is that a lot of teams and a lot of enterprises are looking at, ‘how can we enable our scientists and our analysts the ability to access data virtually?’ So, instead of recreating redundant data sources, we’re actually enabling data virtualization at Harley-Davidson. We’ve been doing that — first working with our DB2 warehouse on cloud and connecting to some of our other trusted versions of data warehouses that we have throughout the enterprise to enable analysts to do some quick reporting without having to bring all that data together.
How does self-service fit in? Were you able to achieve some level of self service? How far can you go there?
Stasiewicz: We were. We did use some other tools. I’ll be quite honest, aside from just the IBM tools today that’s enabled some of that self-service analytics, SAP SAC was one of them. Alteryx is another big one that our analyst team likes to use today to wrangle and bring that data together. That really allowed for our analysts spread in our reporting teams to start to build their own derivations, their transformations for reporting themselves, because they’re more user interface-based versus going in the back-end systems and having to write data, scrape or write SQL queries. That usually takes time and requires a deeper level of knowledge than what we’d like to allow for our analysts to have today.
Watch the complete video interview below, and be sure to check out more of SiliconANGLE’s and theCUBE’s coverage of the IBM DataOps in Action event. (* Disclosure: TheCUBE is a paid media partner for the IBM DataOps in Action event. Neither IBM, the sponsor for theCUBE’s event coverage, nor other sponsors have editorial control over content on theCUBE or SiliconANGLE.)
THANK YOU