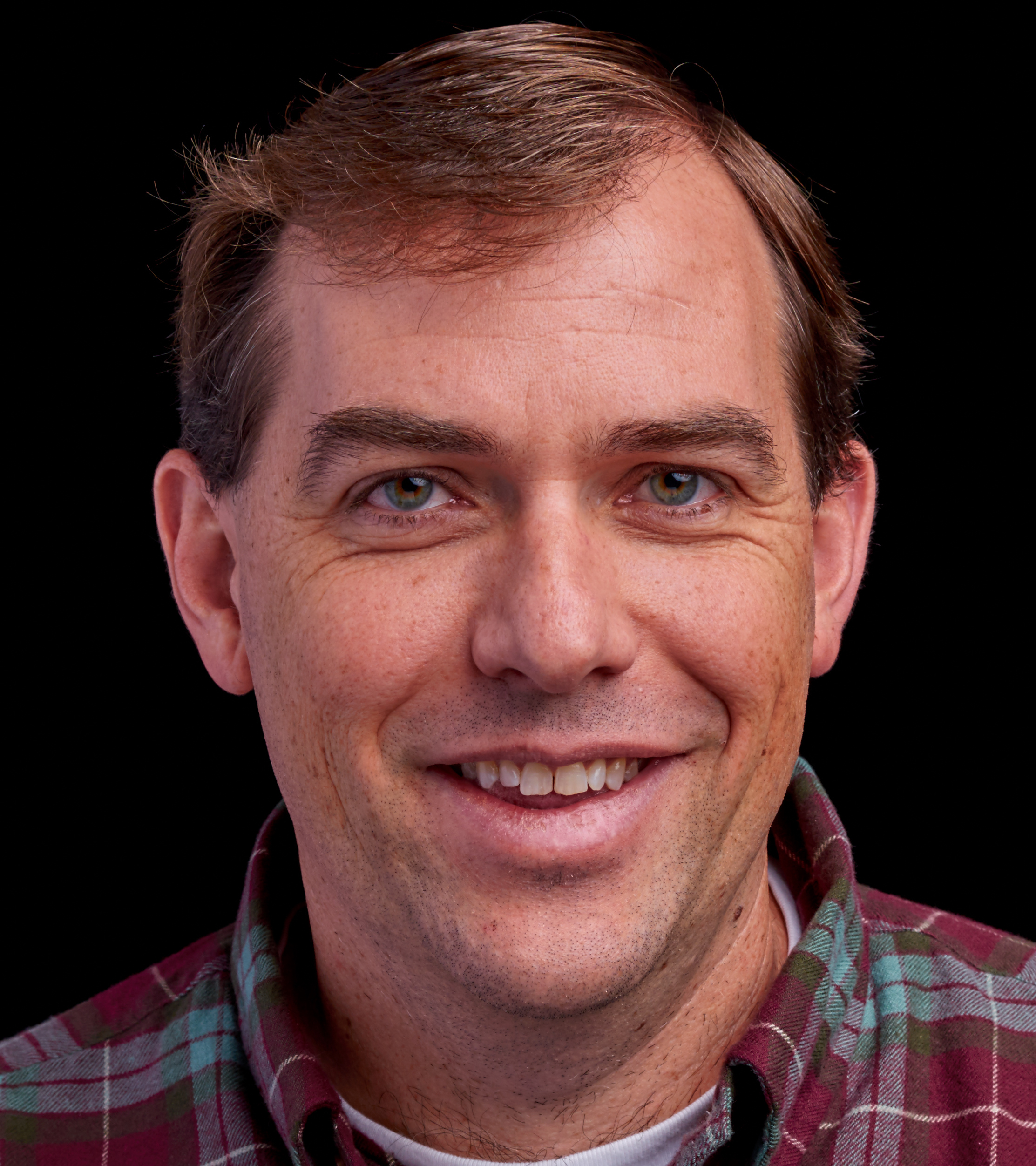
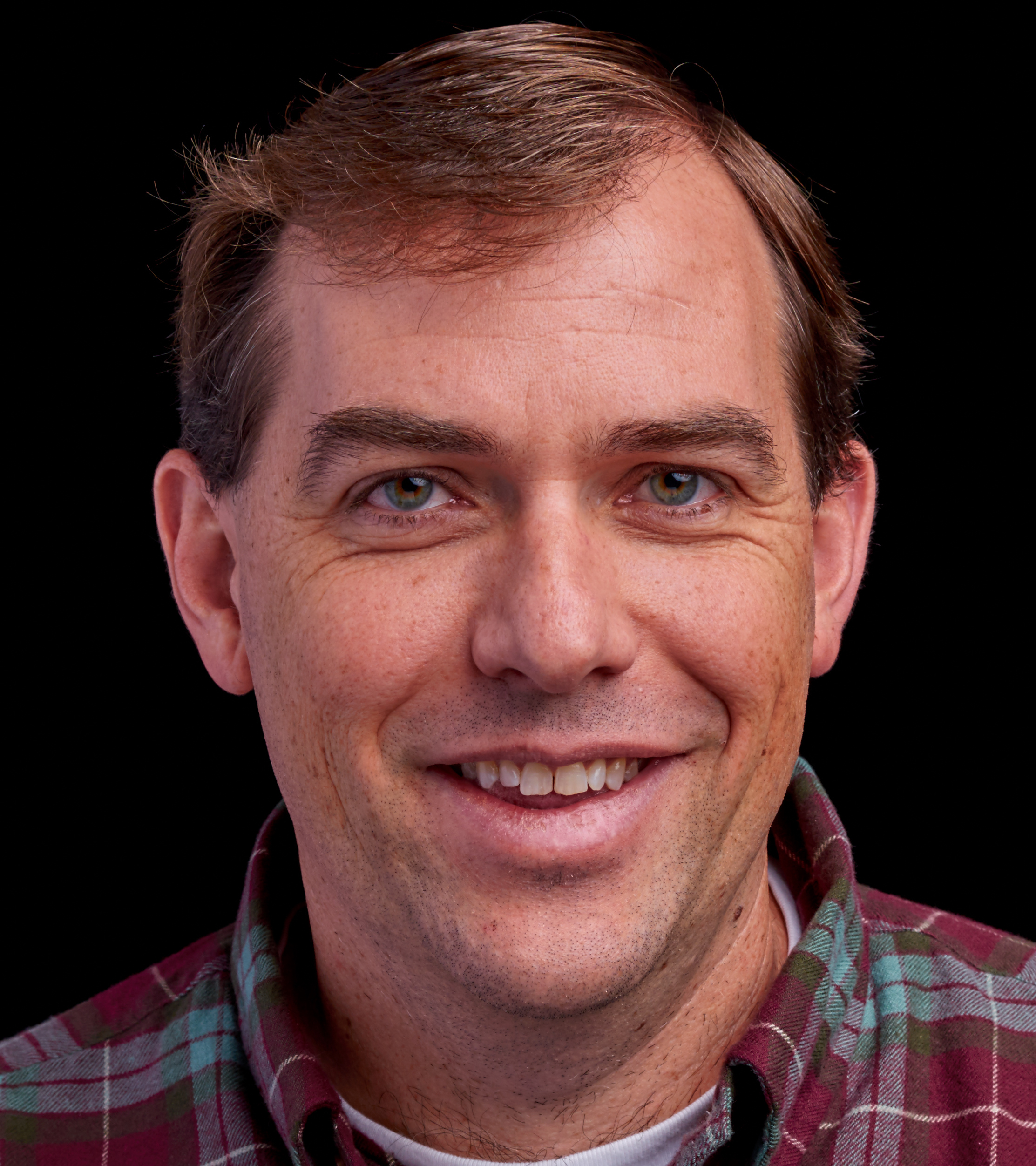
For more than two decades, Amazon Web Services Inc. has been the undisputed leader of cloud computing, but with the recent eight-month surge towards artificial intelligence applications, rivals such as Microsoft Corp. are swiftly gaining ground.
The sudden generative AI shift has caused a rush among developers and businesses to embrace AI, and Microsoft’s monumental $10 billion investment in OpenAI LP has positioned it as a potential industry frontrunner. With these changes, questions have been swirling about AWS’ leadership and position in the new cloud battleground: the generative AI market.
Can AWS maintain its leadership or will it forfeit the lead to Microsoft and other emerging contenders? I wanted to find out directly from AWS, and to do so I had an exclusive interview with Matt Garman, senior vice president of AWS sales and marketing at Amazon.
Garman, who previously headed the EC2 cloud computing initiative, possesses a unique combination of technical expertise and leadership. Now he’s in charge of steering AWS’ original cloud computing business toward the new, fast-growing generative AI market.
In the expansive Q&A (full transcript below), he identifies key opportunities and challenges in generative AI. Here’s a summary of our wide-ranging conversation:
Generative AI is earning increasing recognition among developers and enterprises. Yet organizations have publicly aired concerns about misuse and intellectual property rights. Garman clarifies that these companies are not banning generative AI but rather establishing measures to protect their IP. As AI technology proliferates, businesses will need to adjust to manage the growing flood of AI applications.
In fostering AI adoption, Garman acknowledges the necessity of a multifaceted strategy to serve a diverse customer base. AWS’ inclusive product strategy aims to cater to this diversity with solutions extending from the infrastructure layer to the application layer. He highlights how startups such as Hugging Face Inc., Stability Ltd., Runway AI Inc. and Anthropic have leveraged AWS’ cloud capabilities to scale quickly, democratizing access to technologies typically limited to larger corporations.
The primary concern in generative AI is the security of intellectual property and trust in the data’s results. Garman explains AWS’ approach to ensuring data security, saying, “For our first-party models, we are very careful about which data has been used to build that model.” AWS also provides open-source models within Jumpstart, enabling customers to operate models within its proprietary Virtual Private Cloud.
Industry observers predict that AI will significantly automate tasks and augment human capabilities, revolutionizing business models and society. In this context, Garman views generative AI as an extremely powerful tool for enhancing efficiency and effectiveness. He clarifies that AI is not on track to replace humans any time soon. Instead, it will amplify human abilities, allowing them to concentrate more on the innovative aspects of their work.
The escalating demand for graphics processing units to power AI and train models is a burning issue today. AWS, having invested in machine learning and AI infrastructure for years, is committed to meeting these challenges. It says it operates the largest and most high-performing GPU clusters in the cloud. Conscious of the potential environmental issues linked to power consumption, AWS plans to power all of its global data centers with renewable energy by 2025.
“Generative AI is an incredibly powerful capability that has a chance to make us much more efficient, much more effective,” he says. “It’s not going to replace people any, any time soon.” AWS is poised to navigate the future of generative AI with data security, model versatility and a strategy for managing the challenges and opportunities related to GPUs and custom silicon.
Here’s the full interview with Garman, edited lightly for clarity:
Amazon has been doing AI for a long time. Let’s start out by clearing the air on AWS’ position in AI. Briefly explain the history, the trajectory and the experience AWS has with machine learning and AI.
At Amazon and in AWS, we’ve been super-focused on AI and ML and have long felt for frankly 20 years we’ve been working on this space and have known that generative AI is, has been and will continue to transform how companies do business. And we’ve had an expertise in this for a really long time. AWS is incredibly excited about the potential that generative AI has to fully transform lots and lots of industries and businesses. We wanted to make sure that customers can leverage it in a safe, effective way that makes sense for their business.
AI is an area that we’ve been deeply investing in and an area that we feel passionate about will help our AWS customers and our customers all over the world really transform their business. And we think the approach that we’re taking in AWS is ultimately how most customers are going to want to consume and build generative AI into the applications that they run.
Some enterprises are banning employees from using ChatGPT. Even regulation is rearing its ugly head. Why are people freaking out about AI? What is your position on this? How do you see this playing out?
When ChatGPT came out, it really inspired and caused a broad swath of people to really understand what the power of AI was. It did a great job of bringing into the public consciousness of what’s possible. And so I think you saw a lot of people get really excited and want to jump in quickly. When you look at what some of the big banks are doing or what some of the other companies are doing, they’re not so much banning the idea of generative AI. They’re putting the brakes on their own teams to be careful about putting their own IP into those systems. Part of how those systems learn, like ChatGPT and others, is that when you enter questions in, when you put data into that system, it takes that system, integrates it into what it knows, and then it builds a broader corpus of knowledge that it can answer questions from.
A lot of companies are putting the brakes on in order to have the right controls and security in place so that their own IP doesn’t leak into those (public) models. And I think that’s appropriate. When we talk to our customers and enterprises, one of the things that they’re most worried about is that they understand that in the future, their own IP and their data is actually what’s going to be one of the most valuable and differentiating things that they have going forward. And so what they’re putting in place is controls to ensure that they have that right set of controls over their IP so that their employees don’t inadvertently share it into one of these models and it gets kind of uploaded, and then available for everybody, and they kind of lose that IP.
They don’t realize that they’re actually contributing to the revised corpus with their IP, which then comes into all kinds of issues around IP rights and releases it essentially. Imagine if you’re a bank, you want to make sure that your data doesn’t get loaded up into the model, so that a competing bank can learn from what you’re doing.
What are some of the key differences on how enterprises want to consume generative AI versus say how an independent software vendor wants to consume generative AI?
Everyone is going to want to use generative AI and appropriately. Generative AI is a powerful technology that has a potential to help us be more efficient, more effective, and really change customer experiences. I think when you think about those differences and how a startup thinks about things or how a large enterprise thinks about things or a SaaS [software-as-a-service] provider thinks about things, you know, a lot of them are not totally different as you might think, their stages of adoption may be different.
If you’re a startup, you’re trying to figure out how can you get out there fast, how can you iterate quickly? How can you get access to some of these technologies that may only normally be accessible to really large companies? And that’s one of the things that cloud and AWS enable. And so you see startups like Hugging Face, like Stability and others. Anthropic is building on top of AWS because they can get large-scale capacity quickly, they can iterate quickly, they can learn and they can grow.
A lot of startups love to use the cloud. And that was, as you know, that’s where AWS kind of grew up from the very beginning as the value proposition, and generative AI is no different there.
So when you go look at, at larger scaled ISVs, it’s really not that different of a story. I think one of the things that they love is the ability to scale, the ability to test new capabilities — really cool stuff that these larger established ISVs are doing and rolling out really innovative new technologies and capabilities all based on generative AI.
Enterprises have a little bit different needs than, say, a developer or startup that’s growing rapidly. Enterprises might want SaaS-like experiences like CodeWhisperer, for example, or developers wanting, say, Bedrock for the building blocks for generative AI. How do you mix that together?
Our take is there is no such thing as a homogeneous customer. Customers all have different ways that they want to consume this technology. Some are going to want to consume it at a package layer, some are going to want to consume it all the way at the infrastructure layer. And I think that’s where AWS really shines.
For people that want to build their own models, we build our own silicon and increasingly that is going to be a competitive advantage for us to have a choice. We have, and for a long time have been, the best place to run GPU infrastructure. Our customers love running large scale GPU clusters in AWS and we also build our own infrastructure that we think has cost and performance advantages … Trainium for large training clusters and Inferentia for running large inference clusters.
If you think about SageMaker, it’s the development platform of choice of almost every single ML developer out there to make sure that they’re doing safe AI, make sure that you’re testing various different models to see what actually works well with your application.
And Bedrock is providing an easy-to-use API for the variety of models, whether you’re using a large number of these foundational models that folks are going to want to be able to use for different sets of use cases and they may even want to combine different ones.
Consistent across almost every single customer that wants to use generative AI is that they want to make sure that they do it in a secure, safe environment where they know that their IP is safe, where they can have explainability, where they have as much information as possible on how the model was created. This is where our focus is: How can we give enterprises that assurance that they have the highest performing infrastructure, but also the best and most secure platform in order to go build that generative AI so that they know that their data and their IP doesn’t leak out to places where they don’t control it?
How do you ensure security? What’s the key value proposition there?
There’s a range of things for our first-party models. We have our own models, which we refer to as our Titan models. With those, we are very careful from a copyright perspective of which data has been used to build that model. We’re very clear about that. Customers know that they can be assured that the data that went to build that model is something that we have the rights to use to go build it.
We provide things like open source models inside of Jumpstart, part of SageMaker that provides pretrained open-source models to train and tune before deploying. And when you’re running on some of those open-source models, many are becoming really powerful and in many cases are actually outperforming some of the proprietary models today. Customers are able to run those entirely inside of their own proprietary VPC [virtual private cloud] or networking. And so they can run that model. They can isolate that from any sort of external connectivity and know that anything that they use in that model stays inside of that model, stays inside of their VPC.
The same with Bedrock, where anyone who uses any sort of tuning to tune Bedrock models, which is one of the key features that we’ll have inside of our Titan models, we ensure that that data doesn’t leak back into the core foundational model and stays inside of the customer’s VPC. So many of the controls that they use for the rest of their enterprise data work just the same for their generative AI capabilities.
AWS has a broad selection of initial capabilities, you mentioned you have first-party models, OpenAI has theirs, it’s not on AWS, and then there are third-party models via Bedrock. And then the recent wave of open-source innovation, just in the past like month and a half, you saw a huge surge. When will customers want to use the prominent models that you guys have and when will they want to use some of these long-tail Bedrock-like products and open source? How will you balance those?
Our goal is to give customers both the choice to be able to run what’s best for their application. For example, a model that’s optimized for a financial services customer may not be the one that’s optimized for genomics data, may not be the one that performs best for e-commerce or images or any of those other things. Another example is Stability AI, Stability is a great model for images right now, but not for text. And by the way, they’ll change over time and they’ll add some of those. We want customers to be able to pick and choose what is the best model that they want to use for the best use case.
Enter SageMaker. We make it really easy for customers to A/B-test things. And in a cloud you can do that. You don’t have to spend billions of dollars to go build your own model. You can leverage some of these others and test if model A performs better than model B or if some combination of models is actually the optimal one for you.
Over time people will tune and kind of build on top of some of these foundational models and they’ll have their own model that they tune and then condense from those. Then that’s the thing that they’ll actually use in production. And we want to make it super-easy for them to do that process, but in a cost-effective and secure way in order to actually use that and scale that out. Cost is one of the things people are looking at in the future, and they’re worrying about the cost of generative AI. Whether it’s first-party Amazon models or open-source or other proprietary ones, our goal is over time to support every single model out there.
Generative AI reminds me of the early days of AWS when you had the same. Do I build a data center and provision all this stuff or do I put it in the cloud and get instant value, variable elasticity? I mean, the same kind of thing is happening here with the generative AI and other foundational models. You can stand up your own if you want, good luck with that, or mix and match and code your own.
That’s right, and look, and over time you’ll see us leveraging generative AI more and more in some of the applications that we make available to customers as well. CodeWhisperer is a great example. It’s a coding companion, but still with that enterprise in mind, right? We have automated reasoning built in to make sure that you’re building secure code. We have the ability to highlight, if we’re showing you code samples that come from open source, what is the licenses? And to ensure that you want to use the code sample that comes from open source.
Our focus is a little different from the others. We are laser-focused here at AWS on how we can have generative AI make our customers successful, and a little bit less distracted by productivity suites or search or any of those other things. We are laser-focused on how we can make sure that our AWS customers can take best advantage of these technologies. And we start with those use cases and then work from there.
We are seeing AI take on more tasks, shifting the humans role, augmenting the human capability. How canAI can actually automate and differentiate for companies?
I think generative AI is an incredibly powerful capability that has a chance to make us much more efficient, much more effective. You know, it’s not going to replace people anytime soon. CodeWhisperer is not going to make it so that you don’t need developers anymore. It’s going to make it so developers don’t have to write bespoke code. It’s going to make it so that developers can write more secure code, but they can focus on some of the innovative customer experience that I can deliver for my business and for customers and not have to worry about the blocking and tackling of necessarily writing code. I think the future coding language is probably going to be English and that’s OK.
Or voice?
Yeah, exactly. But it’s going to be saying it in English, and then the tools will translate that into code. The expertise may not be understanding the nuances of Java or C++ or anything like that, but that’s OK. It just changes some of that skill pieces, now you got to think about the parts of your application that you want to go build as opposed to how you build it.
Will the model be “Human plus AI is better than AI by itself”?
100%, yep. And that’s going to be like that for a really, really long time and probably forever.
Graphics processing unit supply seems to be a bottleneck. There’s demand for AI training and inference. What do you see as the core constraint in the industry and what does the industry have to do to have a line of sight to relieve the pressure?
There’s a number of constraints here. I think one of the things that’s key is that it takes a lot of compute power to go build some of these foundational models. It takes billions of dollars of GPUs, but not just GPUs — servers, networking, data center, power, electricity, all of those pieces, right? And we’ve been building a lot of those things for a long time.
We have the largest GPU clusters anywhere in the cloud. We have the best-performing GPU clusters in the cloud and long-term, I think that power is actually one of those things that you have to really think about because these clusters have the potential to use hundreds of megawatts to gigawatts of power. Now, you know, by 2025 we’ll be running all of our global data centers on renewable energy, which helps a lot because there’s a risk that some of that power causes environmental issues.
We’re going to want to think about how we scale those (GPUs) in a bunch of different ways. And I think that’s part of where our custom silicon comes in. Yeah, GPUs are awesome. Nvidia does a fantastic job of building a really good product and they’re going to be super-important in this space for a really long time. But we also think there’s space for custom-designed silicon, and we think products like Trainium have the real potential to help customers lower cost over time, reduce the power footprint and improve performance. It’s a competitive advantage for our customers, and for our business, by having that low-cost option for customers that actually, in some cases, can out outperform what GPUs can do.
AWS has done lots of work at the silicon and the physical layer, you guys have been squeezing every ounce of physics out of it at AWS for years. The real technical and business value and action of AI is up and down the stack. Can you just share your thoughts on the kind of generative AI that’s happening up and down the stack?
I think that there’s just going to be innovation across the board. Every single industry, there’s going to be innovation at networking, there’s going to be innovation at the compute layer, there’s going to be innovation at the tool layers, there’s going to be innovation in supporting services, like vector databases and other things like that. There’s new startups that are popping up every day focusing on different parts of that tool chain.
All of those things are really interesting, and as we’ve talked about, all the way up to the application stack where there’s all sorts of new technologies. So I think it’s a technology that can be applied almost anywhere. Whatever we talk about this month may be totally different six months from now. There’s a lot of folks out there innovating, and that’s part of why AWS is great. We give people a platform to go innovate.
What I’m seeing in my reporting is that there are two types of customers right now on the AI side. There’s ones that have been into the cloud and ones that aren’t fully in the cloud. When big shifts happen like the pandemic showed us and now with the generative AI rapid transition, it was obvious that when in the cloud “the trend is your friend.” How does having a presence in the cloud influence a company’s ability to capitalize on the rapid transition to generative AI, considering the advantageous trend observed during major society consumption changes like the pandemic?
Getting all of your data and your workloads in the cloud enables you to adjust to changing trends and technologies. Generative AI is one of those, that every single customer and company has to really think about how they’re going to integrate into everything that they do. And it’s harder if your data’s not in the cloud. Step zero is to make sure your data is in AWS, that it’s available in a data lake, that you can look at, that your compute and workloads are there, that you have your structure around it.
Many of the customers who have already jumped in that cloud journey are in a good place to move fast and others are hustling because they realize that this is capability that they’re just not going to be able to do in their own data centers. There’s just no way of doing it. The scale is just not possible. The speed, the technology’s moving, it’s just not possible to do in your own data centers. This is further evidence and impetus for people to move to the cloud quickly, but to understand generative AI is going to change their business over the next many years.
We have OpenAI, which is not available on AWS, Anthropic, which is available on AWS. I’ve been talking to insiders and VC firms in some of the top enterprises, and they all want open, they want choice. Many complain privately they would like to see OpenAI run with Bedrock. Would you ever offer Sam Altman lots of customers for OpenAI via Bedrock?
Sure. I think all customers want to have a choice. I would love to have every Generative AI model that customers are interested and excited about running in Bedrock and AWS.
Open source is growing fast in the generative AI space. Customers and developers also want open source. There’s been a big surge just in the months. It’s the early cloud AI days and you guys have the big models, and the long tail of open source models are emerging. What’s your view on the mix of the models, the big prominence to the open source and the long tail? How do you see the mix playing out?
It’s hard to say. We’ve seen awesome results from some of the distilled open-source models recently. Facebook’s LLaMA model was awesome. I think there’s a new model that just came out this week that’s LightOn, I think, which is a even smaller model that’s outperforming LLaMA now on the open-source world. Totally trained on AWS.
There’s a lot of this interesting innovation that’s going out there. I think there’s also always going to be a need for these really large core models too, that help distill some of these open-source models and specialized models. But it’s such a fast-moving space, it’s hard to say. That’s why I think that the choice is so important. We at AWS prefer to make it really easy for customers to switch horses if they find a one that they like better later.
For startups out there, what’s your advice? The entrepreneurial track is not the same as it was during gen one of cloud. You got to get customers, but scale is a huge thing. How do you see this next-gen wave hitting, what’s your advice to startups and for companies?
The way we think about it is that AWS is a great place for startups and all sorts of customers, actually as a channel to get to customers. The vast majority of enterprises and companies out there are running their businesses in AWS. But we’re not going to go build a broad swath of innovative new technologies. We’ll deliver a lot of stuff, but there’s a lot that we won’t build and partners are key to everything that we do in AWS. We have a lot of programs from Marketplace all the way through to some of our channel programs and certifications to ensure that our partners are available for our customers to use in a really easy-to-use, really easy-to-integrate way.
So I’d encourage all of them to look at some of those programs that we have in the partner ecosystem and in the Marketplace as we’re seeing that that’s one of the ways that a lot of enterprises want to bring these tools together to be able to use a broad swath of things.
THANK YOU