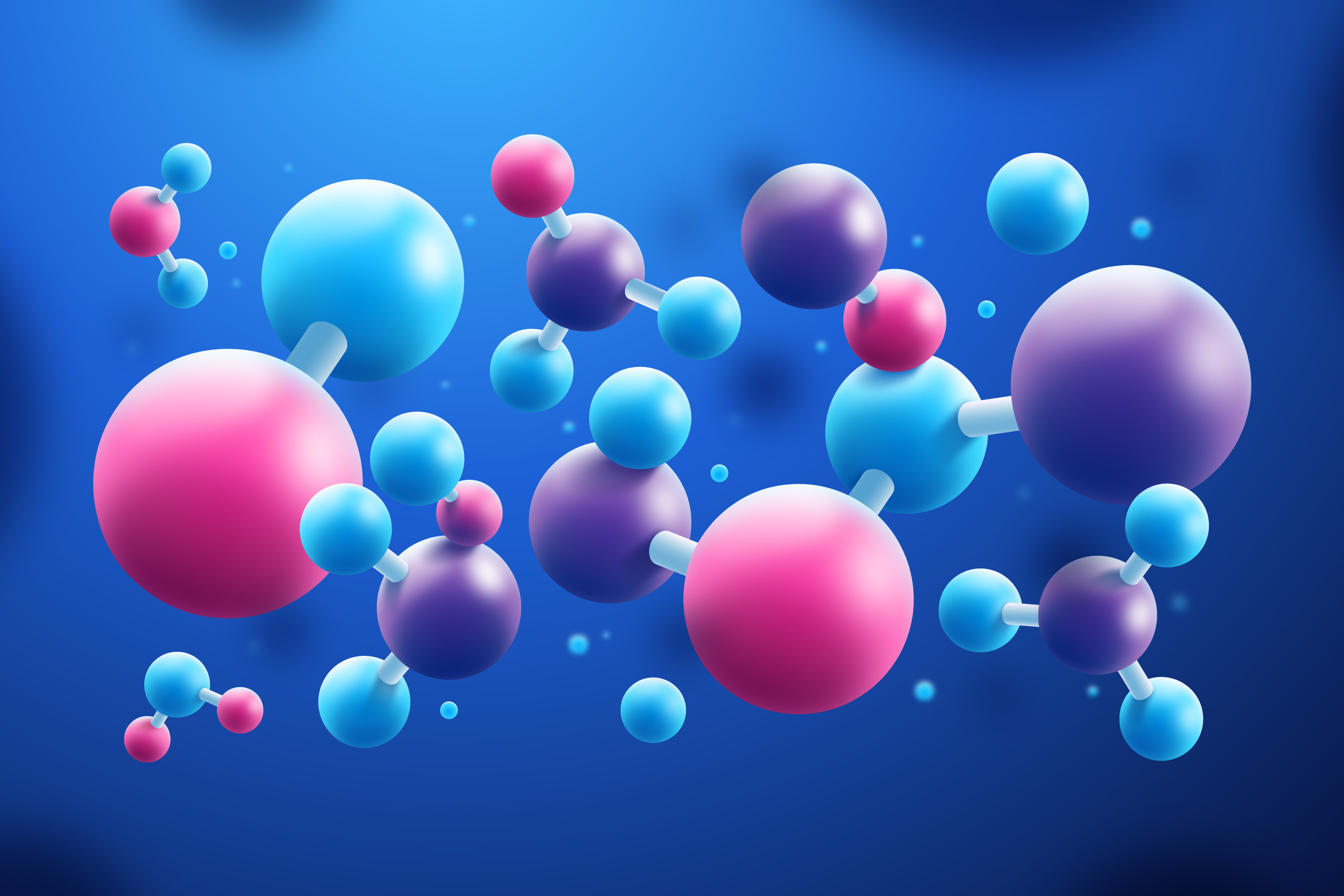
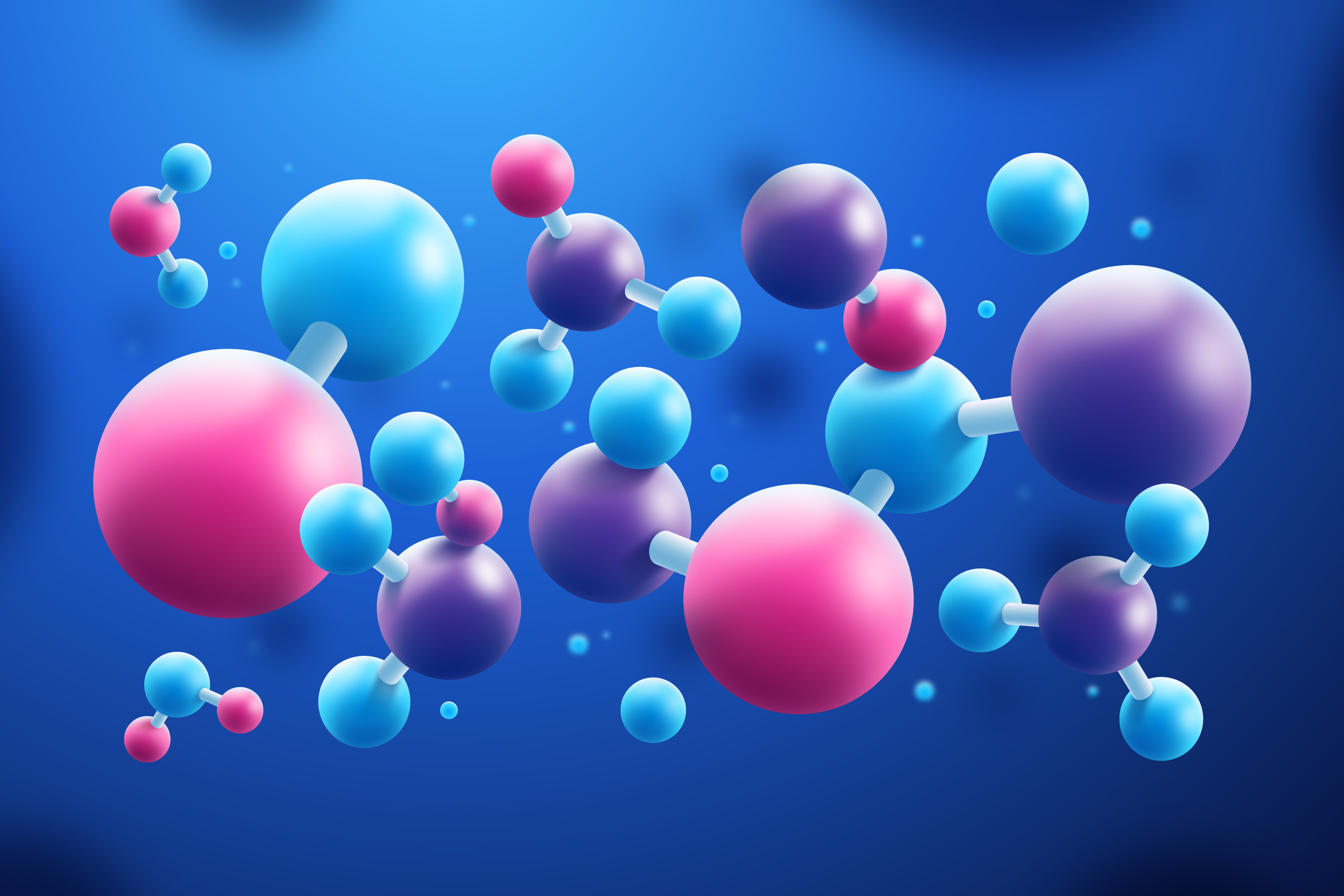
Researchers at the Massachusetts Institute of Technology’s Computer Science and Artificial Intelligence Laboratory have created a new, AI-powered system for designing more durable microstructured composite materials for use in vehicle manufacturing.
In a blog post today, MIT CSAIL Ph.D. student Beichen Li, who led research on the project, said the new system integrates physical experiments, physics-based simulations and neural networks to accelerate the design of new composite materials used in everything from cars to airplanes. Using the system, Li and his team designed entirely new microstructured composites that are much tougher and more durable than traditional materials, striking what they say is the perfect balance between stiffness and toughness.
“Composite design and fabrication is fundamental to engineering… [and] the implications of our work will hopefully extend far beyond the realm of solid mechanics,” Li said. “Our methodology provides a blueprint for a computational design that can be adapted to diverse fields such as polymer chemistry, fluid dynamics, meteorology, and even robotics.”
Traditionally, composite materials are designed through old-fashioned trial-and-error methods, but it has always been a slow process given the discrepancies between theoretical models and the practical results. The difficulty stems from the fact that the strength and durability of composites is determined by their atomic and molecular structure. As Li explains, these building blocks must be integrated extremely carefully in order to achieve the desired balance between stiffness and toughness, which are the two most critical properties sought by vehicle manufacturers.
The researchers detailed their methodology in a paper published in Science Advances. Their work involved a large design space and two kinds of base materials – one hard and brittle, the other soft and durable – which were merged together in different ways in order to create the most optimal microstructure for a composite.
Li’s team began by first crafting 3D printed photopolymers that were about the size of a smartphone but much slimmer, before adding a small notch and triangular cut to each one. The photopolymers underwent ultraviolet light treatment before being evaluated for their strength and flexibility using a standard industrial testing machine.
These physical tests were conducted alongside sophisticated simulations using a high-performance computing framework that allowed the team to predict and refine the characteristics of each composite before they were created. Li explained that the simulations allowed his team to experiment with binding different materials at a microscopic scale, fusing rigid and pliant substances in just the right way to create the ideal balance. They found that the simulations were incredibly accurate, matching the physical test results to validate their effectiveness in the real world.
A detailed schematic of the team’s workflow
The actual composite design was performed by Li’s Neural-Network Accelerated Multi-Objective Optimization algorithm, which was tasked with formulating the molecular structure to exhibit the most optimal mechanical attributes. Li said the algorithm operates like a “self-correcting mechanism,” creating new composites, testing their properties, then refining their structure at the molecular level to ensure the simulations align with the results of their physical experiments.
“This evolutionary algorithm, accelerated by neural networks, guides our exploration, allowing us to find the best-performing samples efficiently,” Li said.
Holger Mueller of Constellation Research Inc. said materials design is one of many promising use cases where AI can have a really transformative impact. “Due to limited simulation options, materials design has traditionally always been a slow and complex process, and it’s going to be interesting to see what the first AI-bonded composites can do for the industry,” he said.
Li admitted that the work didn’t proceed entirely smoothly, as his team faced some major headaches in maintaining consistency between the 3D printed composites and the neural network simulations. However, he said he has high hopes for the system he has created. His team is now focused on making the workflow more practical and scalable, so it can be used in real-world materials science.
“Our goal is to see everything, from fabrication to testing and computation, automated in an integrated lab setup,” Li said.
THANK YOU