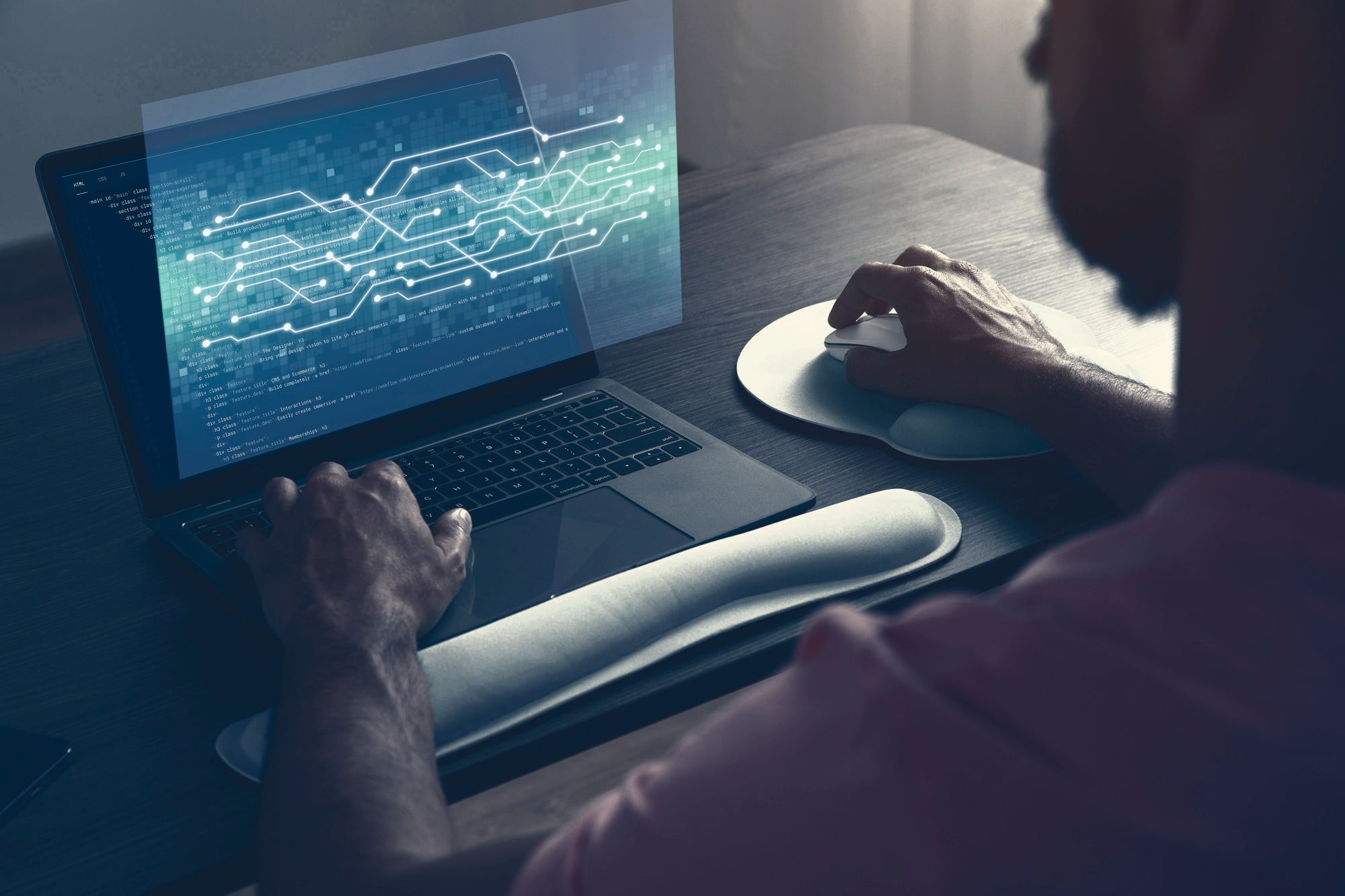
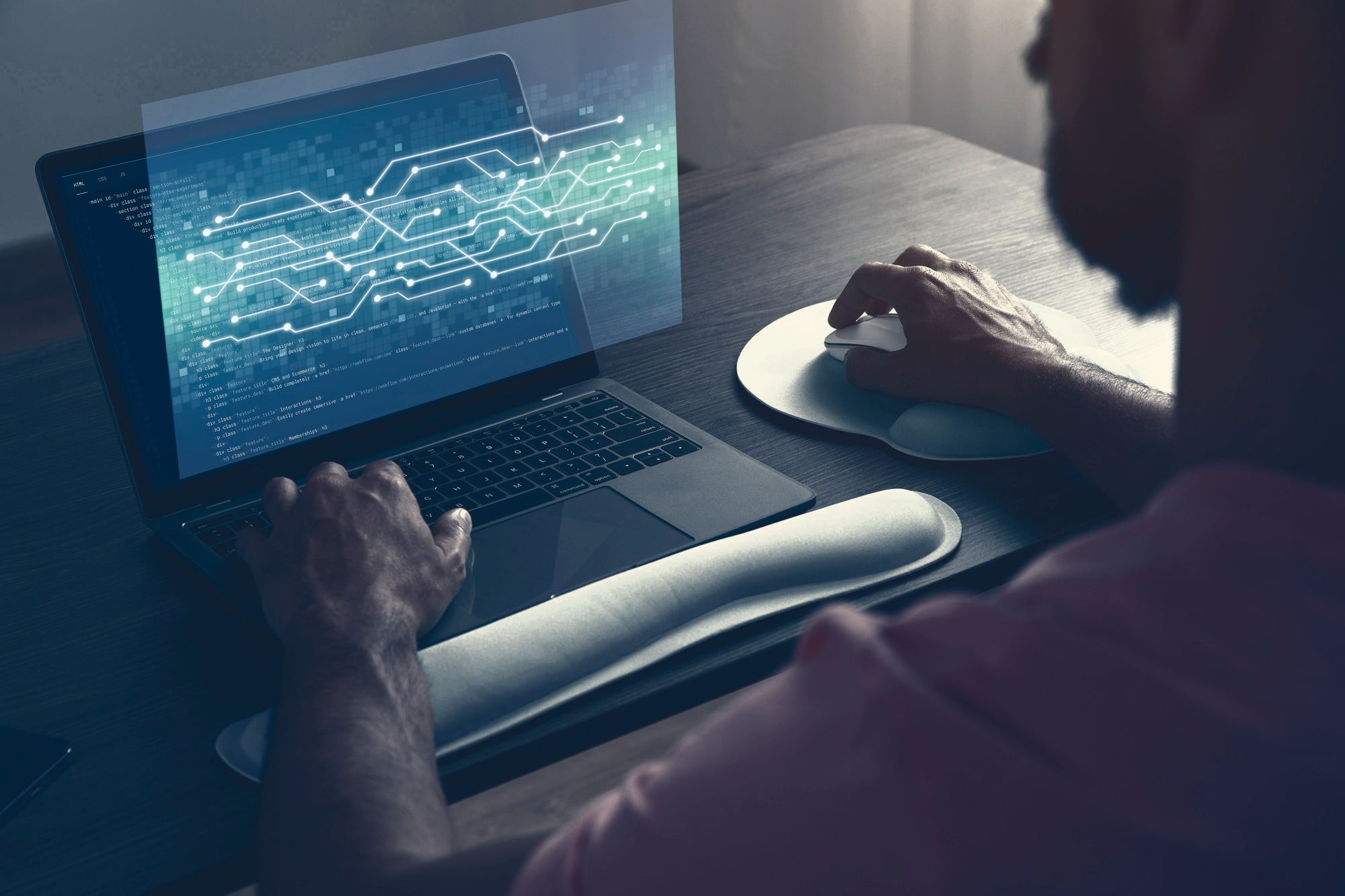
Kinetica DB Inc., which sells a real-time analytics database for time-series and spatial workloads, took to the stage at Nvidia Corp.’s GTC conference today to unveil a new generative artificial intelligence tool for enterprise customers that delivers big advantages for retrieval-augmented generation workloads.
RAG is a technique that allows generative AI models such as OpenAI’s GPT-4 to pull up-to-date information from external databases, so they can provide responses that go beyond the data they were originally trained on. RAG makes generative AI much more powerful, but companies have had trouble in taking advantage of this technique, Kinetica says.
The problem is that existing methods of enriching context, which use vector similarity search methods, are inadequate for quantitative data, as they’re primarily optimized for text-based data. In addition, most RAG offerings are hindered by high latency, since they need to reindex information before they can make it available for vector similarity searches. That means RAG is not suitable for generative AI models that need to tap into real-time operational data.
Kinetica aims to change this with a new solution that’s powered by Nvidia’s NeMo technology, which is available through the Nvidia AI Enterprise platform. The new offering is based on two components – namely low-latency vector search that leverages Nvidia’s RAPIDS RAFT technology, and a query engine that allows it to perform complex data queries in real-time. By combining these two technologies, Kinetica says it can uniquely and instantly enrich generative AI applications by giving them access to real-time and domain-specific analytics insights derived from the most up-to-date datasets.
More specifically, Kinetica said the technologies eliminate the need to reindex vectors before making them available to the query engine. In addition, it’s able to ingest vector embeddings as much as five-times faster than existing databases, according to the widely-used VectorDBBench benchmark.
What’s more, Kinetica says, it’s combining this new capability with new, native database objects that make it possible to define semantic context for enterprise data. The company explained that generative AI needs context about the structure of data in order to understand it well. With its new database objects, large language models can better grasp the referential context required to understand data in a context-aware way.
Kinetica co-founder and Chief Executive Nima Negahban said the new offerings overcomes the limitations of traditional RAG techniques. “This innovation helps enterprise clients and analysts gain business insights from operational data, like network data in telcos, using just plain English,” he explained. “All they have to do is ask questions, and we handle the rest.”
The new features are being made available to database users via a relational Structured Query Language application programming interface, and through LangChain plugins, the company said. This will allow developers building generative AI apps to utilize all of the features that come with traditional relational databases, enabling role-based controls over who can access the data, and the ability to reduce data movement from existing data lakes and warehouses, to preserve existing relational schemas.
Kinetica’s specialized database is novel in that it doesn’t require data to be pre-engineered. Instead, it uses a vectorized query engine that stores information in fixed-size blocks known as vectors, which can be processed in parallel. This means its query engine runs on multiple data elements simultaneously, in order to retrieve rapid results on datasets that scale to hundreds of billions of data points. The software supports the use of Nvidia’s graphics processing units to boost performance, but can also deliver rapid speeds when running on central processing units, enabling customers to use lower cost hardware if they desire.
Constellation Research Inc. analyst Doug Henschen told SiliconANGLE that the novel architecture of Kinetica’s database offering means it’s in a unique position to take advantage of Nvidia’s most advanced software. He explained that it was “designed from its inception to leverage GPU processing power.”
It’s unique design meant that Kinetica was one of the first database vendors in the industry to extend support to generative AI workloads, integrating with OpenAI’s ChatGPT as early as May 2023. Since then it has added further innovations, including a database-native LLM in September and a real-time vector similarity search feature last December.
Henschen said today’s announcement builds on the vector-based similarity search feature, enabling users to define semantic context for industry-specific enterprise data. “In short, it’s building advanced RAG capabilities on a uniquely performant database to deliver breakthrough analytical capabilities for industry-specific verticals,” Henschen added. “It’s starting with telecommunications, but the offering is clearly extendable to other industries served by Kinetica, including the public sector, financial services, energy, automotive and others.”
The company said its new capabilities will have a big impact in several scenarios, including the telecommunications industry, where it can be used to explore and analyze something called “pcap traces” in real-time in order to troubleshoot network problems. Presently, most telcos use tools such as Wireshark to do this, but they require a sophisticated level of protocol expertise to deploy and use. Kinetica, on the other hand, will allow users to analyze network traffic and ask questions in plain English to pinpoint problems.
A second telco use case involves using two data inputs – a stream of L2 or L3 radio telemetry data, combined with a vector database that stores telecoms-specific rules and definitions plus their vectorized embeddings. The company explained that telcos can use this to train LLMs on their own proprietary telecommunications data, and then integrate this with Nvidia’s NeMo framework to create more powerful chatbots. The chatbot will convert user’s questions into queries that can be executed in real-time, with the results delivered to NeMo, which transforms them into natural language responses.
Ronnie Vasishta, Nvidia’s senior vice president of telecom, said enterprises are especially eager to use real-time data in their generative AI applications. “Kinetica uses the Nvidia AI Enterprise platform and its accelerated computing infrastructure to infuse real-time data into LLMs, helping customers to transform productivity,” he said.
THANK YOU