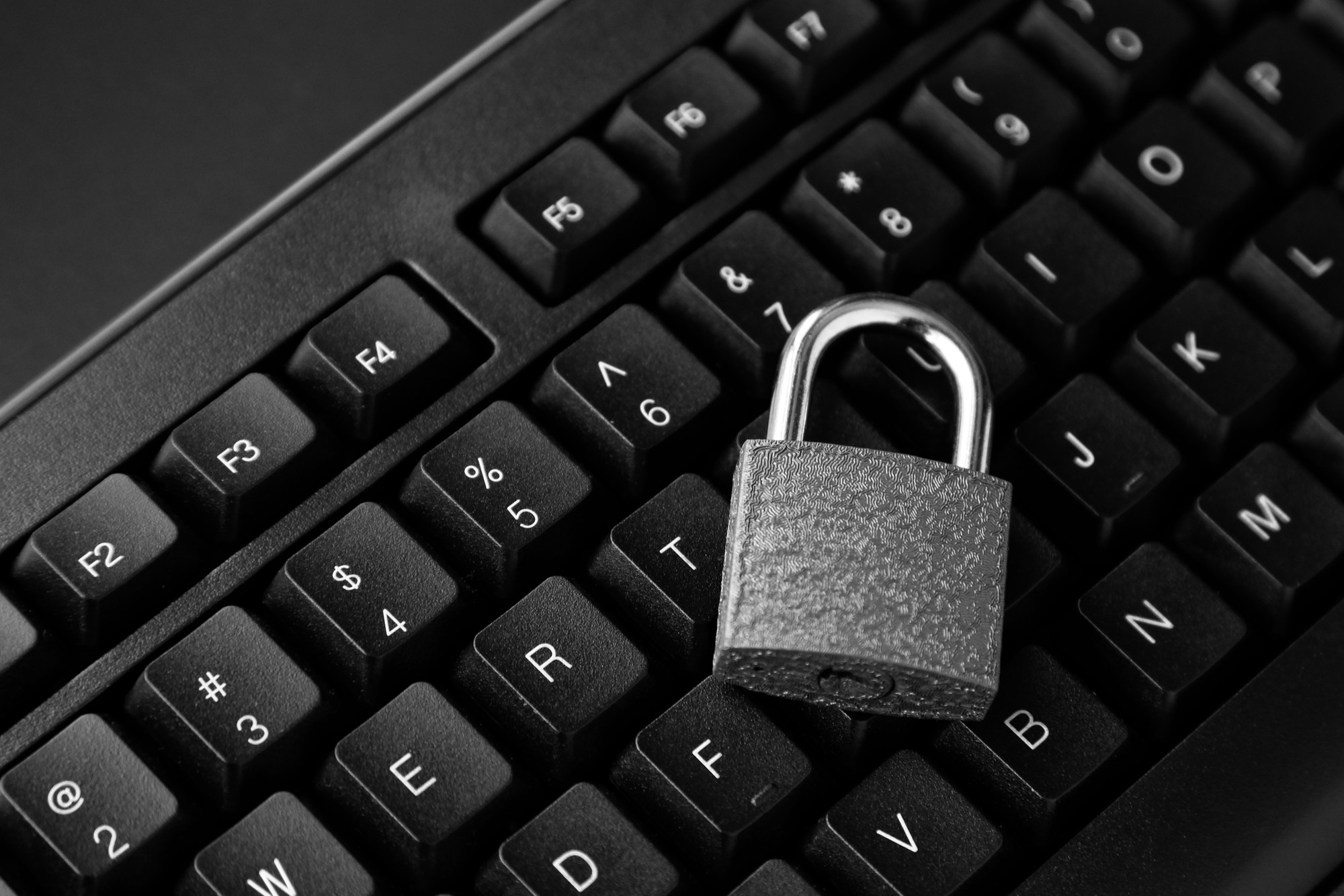
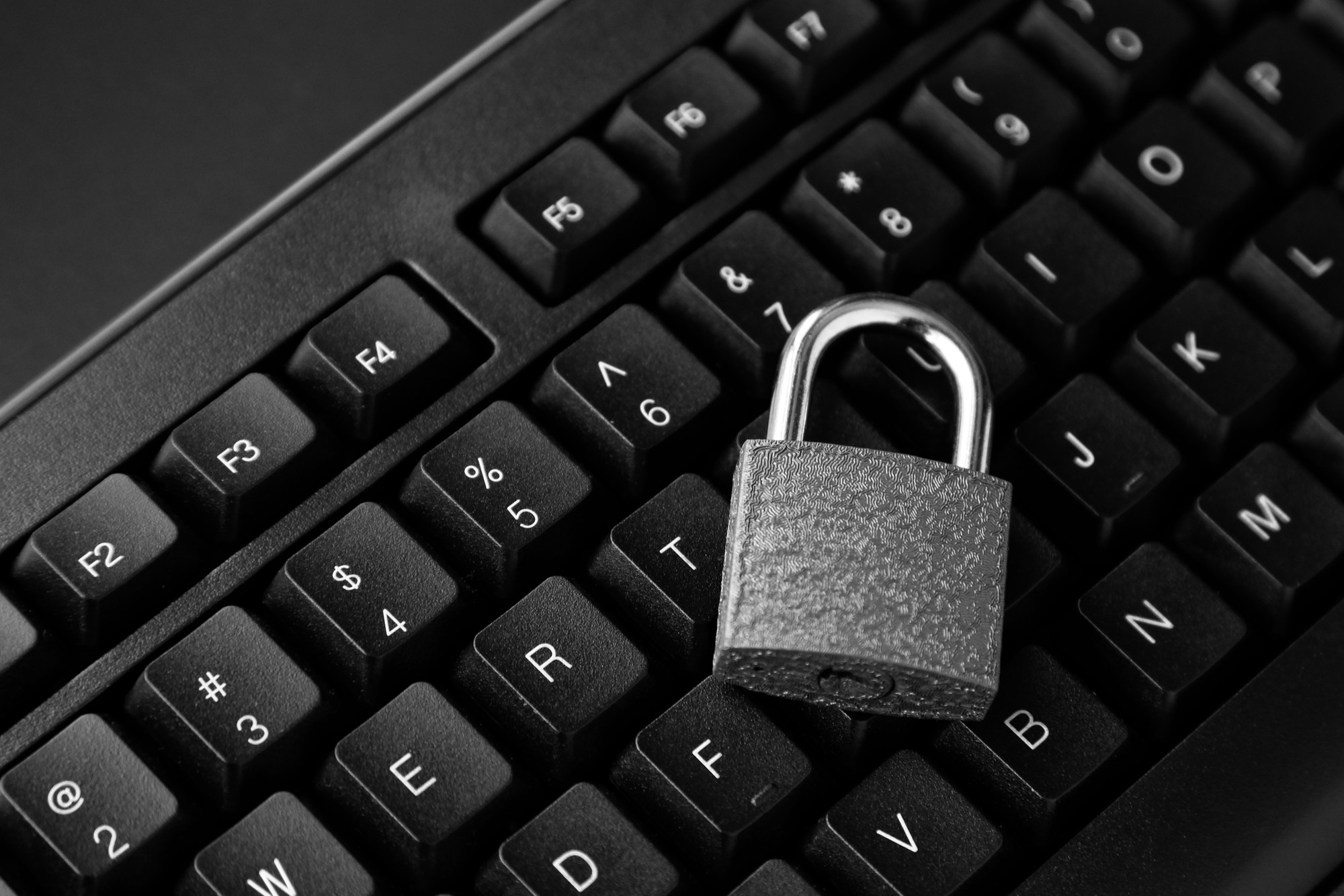
PVML Ltd., an Israeli startup that’s using artificial intelligence technology to enhance access to organizations’ most sensitive data, said today it has closed on an $8 million seed funding round.
The lead investor was NFX, and it was supported by FJ Labs and Gefen Capital.
The startup has created a secure data access platform that makes it possible to analyze and apply machine learning to the most sensitive data. Its system is based on the concept of mathematically guaranteed private outputs that are achieved by introducing randomization to its computations.
Through its platform, PVML can help companies to connect, provide access to, and guarantee privacy for multiple data sources, meaning it becomes possible to obtain live, real-time insights from even the most sensitive data.
According to the startup, just about every organization today struggles to process sensitive data due to the associated privacy risk. Although strong encryption systems exist to secure data that’s sitting idly in a server or being transmitted across a network, that information needs to be decrypted into its original, readable format when it’s actually being used by applications.
That presents a security risk that many companies deem unacceptable, which means they’re missing out on opportunities. One of the most obvious, according to PVML, is artificial intelligence, which can benefit immensely from being able to process data in real-time.
PVML’s system helps secure data as it’s being processed through its unique “Differential Privacy” data protection technology. The startup explains that Differential Privacy is a mathematical framework, pioneered by companies such as Google LLC, Apple Inc. and Microsoft Corp., that can safeguard data by adding just the right amount of “controlled noise” to its outputs, which obscures the information from anyone trying to listen in.
According to PVML, its technology provides the strongest mathematical guarantees that it can keep the private data of individuals safe and private. The statistical noise it introduces to computations is significant enough to protect data privacy, but also small enough that it doesn’t hurt the accuracy of data analytics operations and machine learning methods applied to that information. The startup has crated a short video that explains what this noise is:
This paves the way for companies to extract useful insights and train AI models on datasets that contain the most sensitive information. The algorithms it uses are performed on the analysis itself, on the fly, to ensure that the outputs are secure and can be safely used.
PVML was co-founded in 2022 by a husband-and-wife team – Chief Executive Shachar Schnapp and Chief Technology Officer Rina Galperin, a pair of computer science graduates with expertise in Differential Privacy, and natural language processing and AI, respectively.
“The pain point we originally wanted to address was streamlining access to data. We were motivated by our own experience, seeing how cumbersome accessing data can be even in the most sophisticated enterprises,” Schnapp said. “We thought there has to be a better way.”
Data that’s being processed can be secured using confidential computing techniques such as homomorphic encryption, which takes advantage of Software Guard Extensions or SGX, which is a set of security-related instruction codes built into some of Intel Corp.’s more advanced central processing units. SGX provides the ability to split off parts of a server’s memory into trusted execution environments, or TEEs, which can be thought of as enclaves that are isolated from the rest of the machine.
However, PVML argues that homomorphic encryption isn’t efficient enough for AI applications. The problem is that this technique comes with a large performance overhead that makes it extremely costly, and therefore unfeasible for many companies. PVML’s Differential Privacy tech eliminates these computation and memory overheads, and because it guarantees privacy at the output level, it also eliminates the risk of reverse engineering and attribute inference attacks.
As another advantage, PVML says it’s able to combine Differential Privacy with advanced retrieval augmented generation or RAG capabilities, meaning it can provide secure access to both structured and unstructured data such as images, handwritten notes, audio and video files, potentially enhancing the power of AI models.
NFX co-founder and General Partner Gigi Levy-Weiss said many organizations today are too afraid to connect their most sensitive data to AI systems because they fear it could be exposed. “They fear this for good reasons,” he said. “But PVML’s unique technology creates an invisible layer of protection and democratizes access to data, enabling monetization use cases today and paving the way for tomorrow.”
THANK YOU