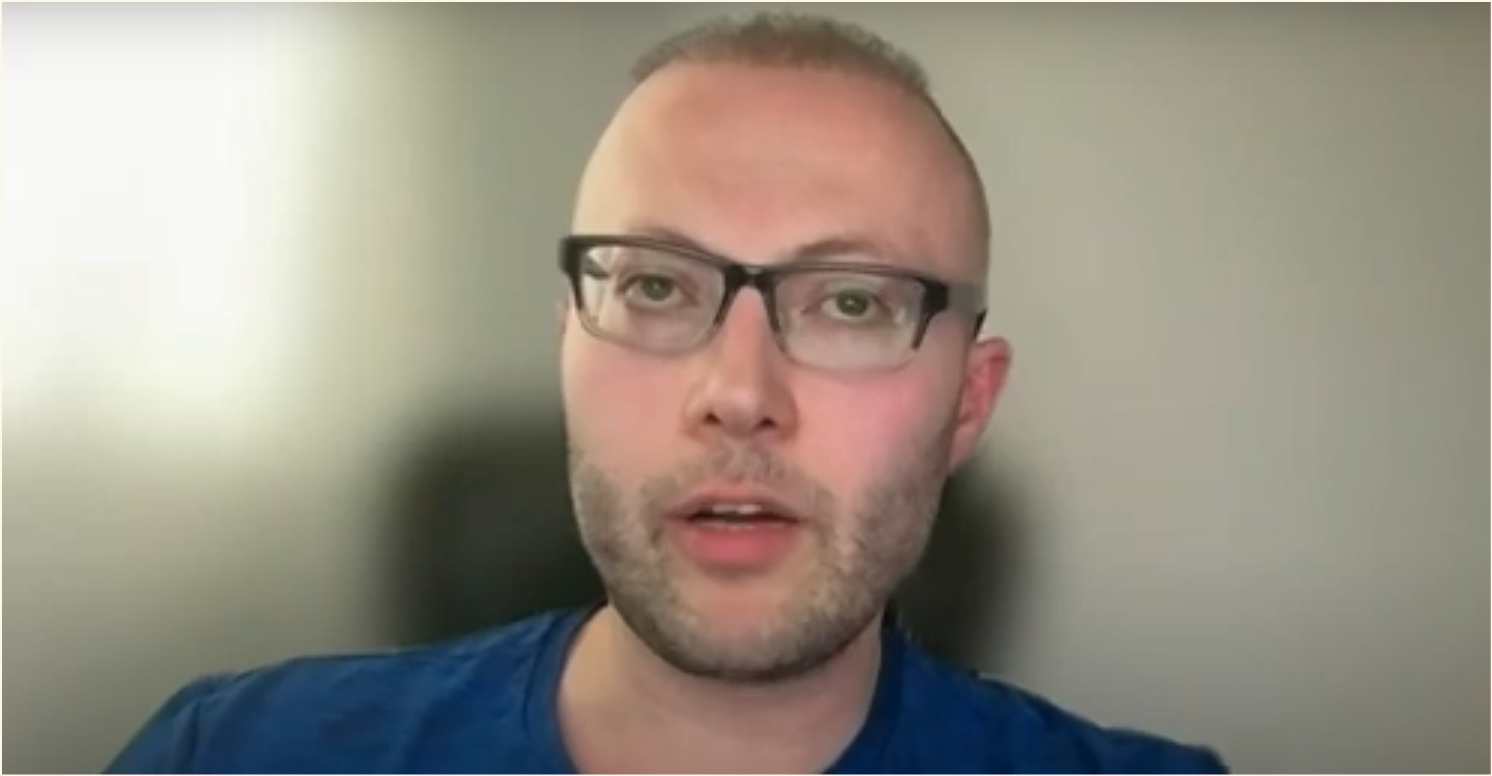
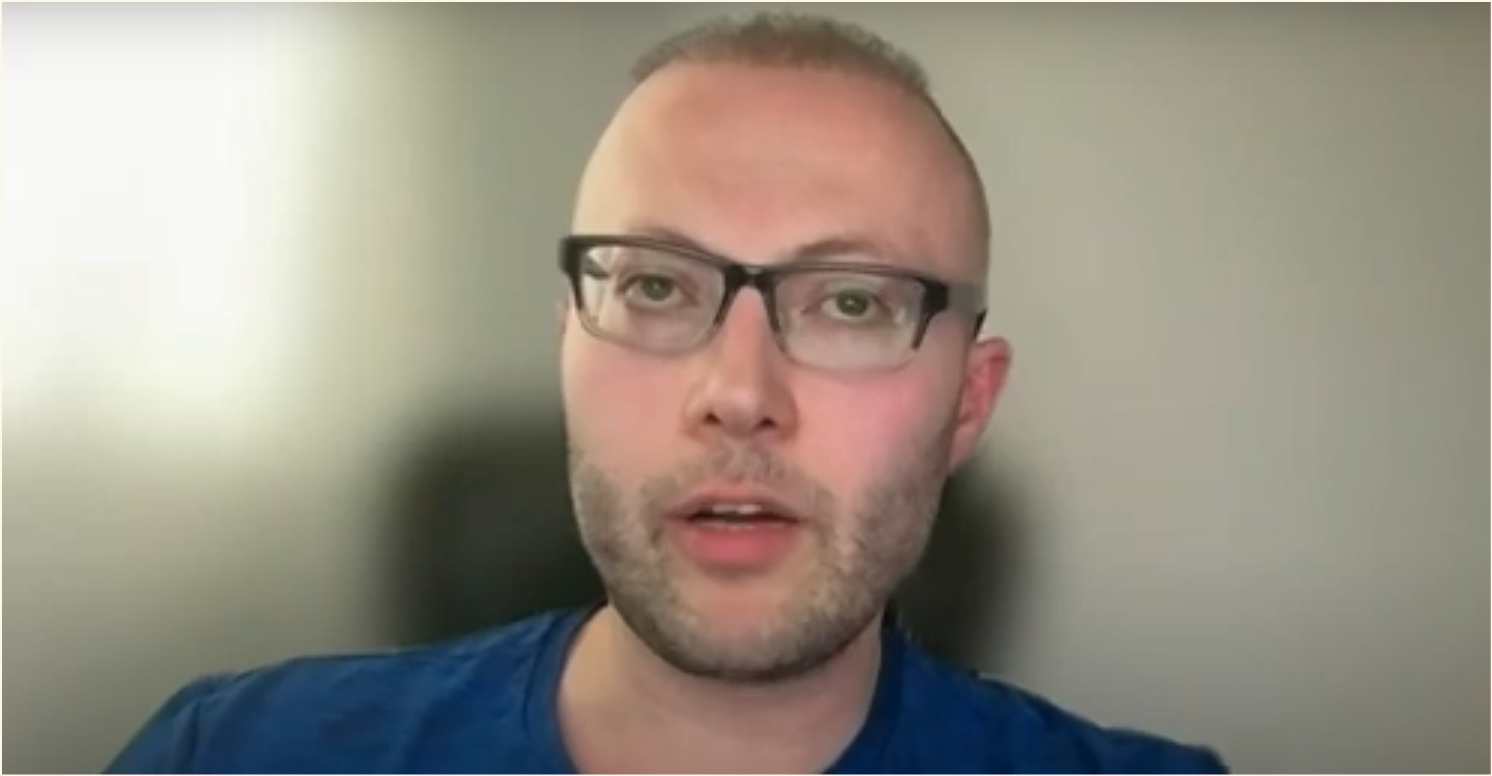
The dawn of accelerated computing is underway, marking a transformative era in the tech world.
As artificial intelligence and machine learning take center stage, innovative hardware solutions and novel architectures are outpacing traditional computing methods.
“Traditional general-purpose computing is like a Swiss Army Knife,” said Shehram Jamal (pictured), director of product management for AI applications software at Nvidia Corp. “It can do many things, but none of them extremely well. It’s a one-size-fits-all approach where the same processor is used for various tasks from browsing the web to editing videos. Accelerated computing, on the other hand, is like a specialized tool. It’s designed to do one thing exceptionally well.”
Jamal spoke with theCUBE Research’s John Furrier at the AI Infrastructure Silicon Valley – Executive Series event, during an exclusive broadcast on theCUBE, SiliconANGLE Media’s livestreaming studio. They discussed the evolution of AI infrastructure, how accelerated computing is reshaping industries and what the future holds for enterprise AI systems.
Specialization and efficiency drive the hardware underpinnings of accelerated computing. The architecture is built around specialized hardware, such GPUs and tensor processing units. These processors excel at parallel processing, making them better suited for AI tasks such as machine learning, data analytics and scientific simulations. This architecture leads to faster processing times, better energy efficiency and lower costs, making accelerated computing essential for modern AI workloads, according to Jamal.
“General-purpose computing can panel a broad range of applications but may struggle with high-performing tasks due to limited parallel processing capabilities, whereas, accelerated computing has three main concepts, such as heterogeneous architecture, parallel processing and efficiency,” he said. “Combining CPUs with specialized accelerators, like GPUs and TPUs, to handle specific types of workloads more efficiently is a heterogeneous architecture in accelerated computing.”
The demand for AI-driven applications has exposed the limitations of traditional computing. Modern AI systems are designed differently from previous iterations, requiring specialized hardware and software configurations. For instance, applications such as self-driving cars, medical diagnostics and virtual assistants, such as Siri or Alexa, rely on the capabilities of accelerated computing for real-time performance and accuracy, Jamal explained.
“Basically, you can do faster and smarter applications with accelerated computing,” he said. “You can enhance healthcare with AI-powered diagnostics. You can also improve entertainment as well. And then there are smarter home devices as well.”
In the context of AI systems, the two dominant processes are training and inference. Training is akin to teaching a model to recognize patterns, such as animals in pictures. This process requires vast amounts of data and computational power, making it a resource-intensive task. Inference, on the other hand, involves using the trained model to identify patterns in new data, a much faster and less compute-intensive process.
While training is essential for developing accurate AI models, inference will become the dominant use case in the future, according to Jamal. As AI models become more efficient through techniques such as transfer learning, the need for extensive retraining will diminish. However, ongoing model updates and refinements will still require a robust training infrastructure, Jamal pointed out.
“I would say they’re training the S-curve to flatten as models become more efficient and specialized and techniques such as transfer learning and few-shot learning become more prevalent,” he said.
Here’s the complete video interview, part of SiliconANGLE’s and theCUBE Research’s coverage of the AI Infrastructure Silicon Valley – Executive Series event:
THANK YOU