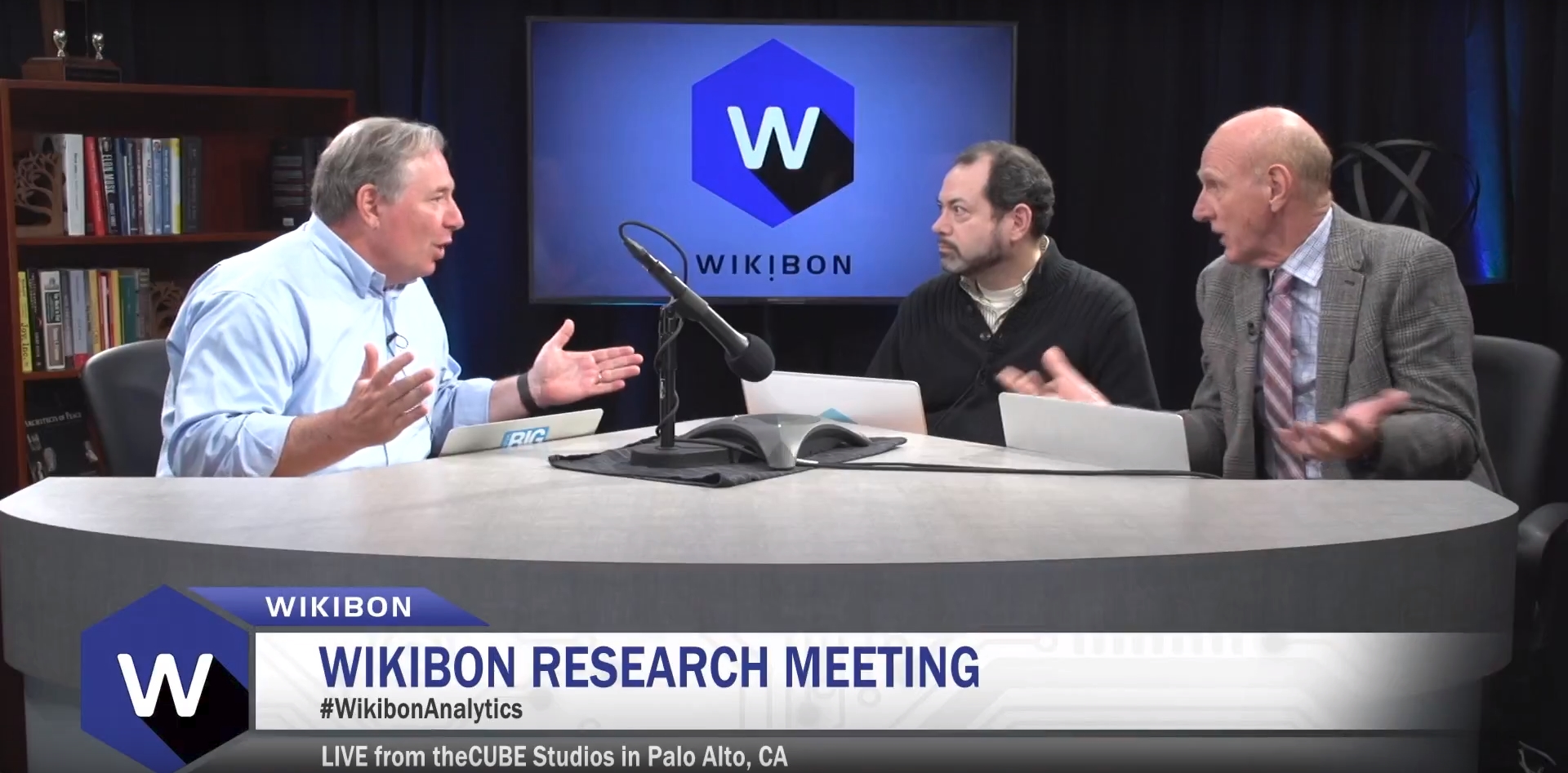
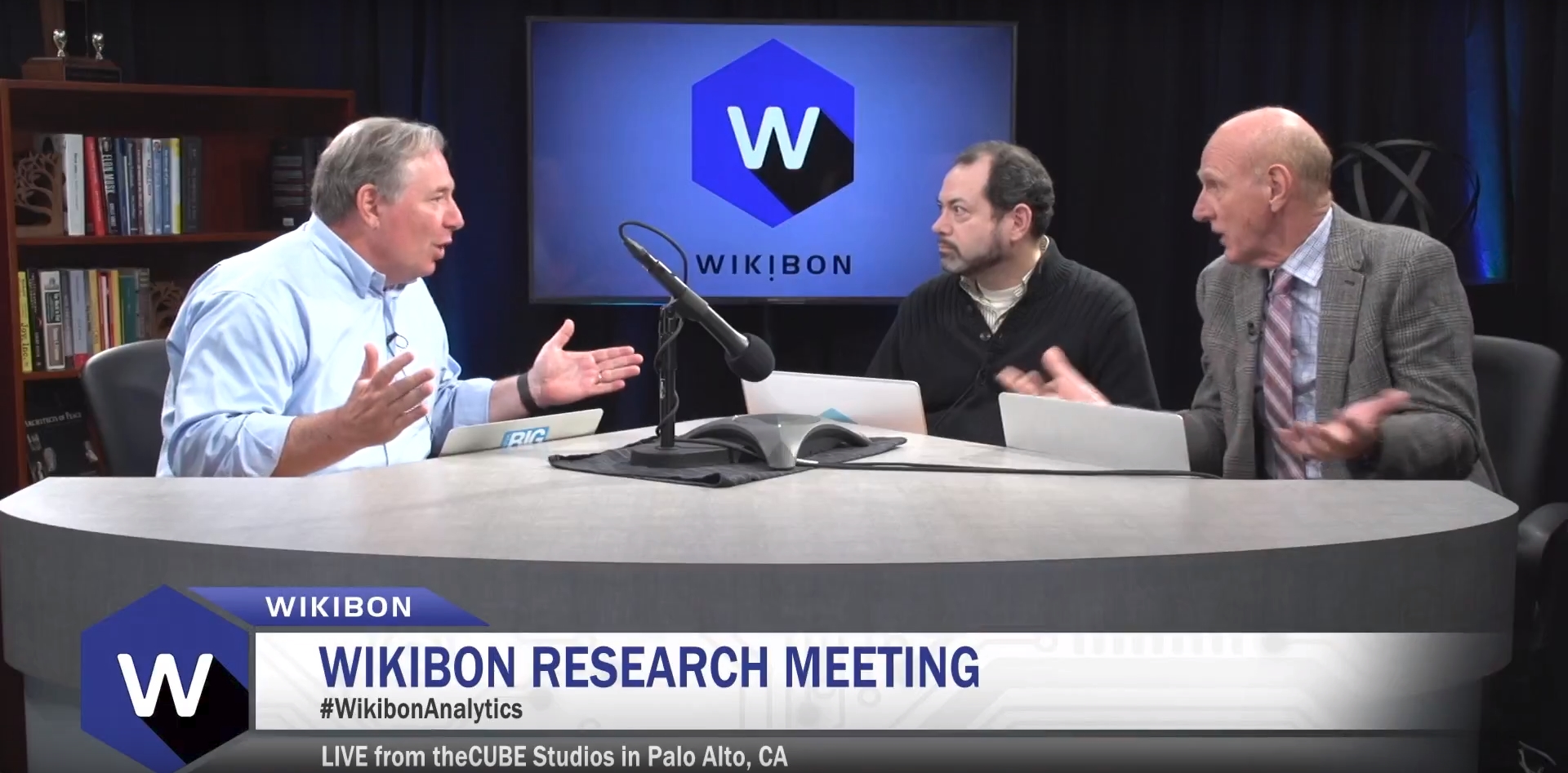
“Internet of things” edge devices are causing quite a few headaches for technology vendors and businesses alike. Their whole selling point — intelligent, real-time decision-making at the edge of computing networks — is defeated by the latency of moving data back and forth to a cloud or physical data center for analytics. But edge devices can’t hack all the compute work themselves. Will edge systems connecting primary, secondary and tertiary data levels with low latency evolve to solve this snafu?
“The software and the devices need to be a single unit. And for the most part, they need to be designed by vendors — not by individual IT teams,” said Wikibon Inc. analyst Neil Raden (@NeilRaden). Raden and several others debated what a workable edge system will actually look like during a special Wikibon Research Meeting.
Most IT personnel are out of their depth in the minutiae of edge devices, according to Raden. For one thing, edge sensors collect data in a manner and for a purpose that are both unprecedented. Unlike virtually all data in any given data lake today, sensor data does not find its way into an analytics model after first fulfilling some wholly different role.
“Sensor data is really designed for analysis; it’s not designed for record keeping,” Raden said. This presents obvious benefits for tailoring models; but there are also challenges to corralling and organizing copious sensor data. “The retention and stewardship of that requires a lot of thought,” he added.
In the long chain of distributed data from edge device to cloud, there are not only complex latency and compute problems to solve; there are also thorny sovereignty and ownership questions, according to Dave Vellante (@dvellante), Wikibon chief analyst. “There are significant IP ownership and data protection issues. Who owns that data? Is it the device manufacturer? Is it the factory, etc.?” Vellante asked.
These and other unsolved mysteries led Maribel Lopez, founder and principal analyst at Lopez Research LLC, to recently describe the state of edge device as “an absolute disaster.” Its problems are so varied, there is no telling where the solutions will come from, Lopez said in a recent interview on theCUBE, SiliconANGLE Media’s mobile live streaming studio. “The IoT camp, I think, is a wild card for everybody right now,” she said.
Indeed, the “solution” may not be possible without scrapping all legacy systems and building from scratch, according to Wikibon analyst George Gilbert (@ggilbert41, pictured, center). Retrofitting edge devices and analytics into existing systems may prove impractical or impossible. Some advanced research on the edge coming from places like the UC Berkeley AMPLab, birthplace of the Apache Spark big data processing engine, calls for a deep infrastructure overhaul, Gilbert added.
“They’re saying we have to throw everything out and start over for secure, real-time systems, that you have to build from the hardware all the way up,” Gilbert said.
Earlier this year, Berkeley launched the RISELab (Real-Time Intelligence with Secure Execution) to improve the ability of machines to make intelligent, real-time decisions. “We need to make it possible for data and code to be protected everywhere they are used, whether the computation runs on an edge device, a corporate data center, or in public cloud computing infrastructure,” said lab principal investigator professor Raluca Ada Popa.
RISELab is looking into hardware capabilities from Intel Corp., such as Intel Software Guard Extensions, or SGX, to enable this security.
Perhaps security, ownership and other questions about edge data can be answered according to which level they pertain to: primary, secondary or tertiary. Businesses and IT teams need to partner with vendors at the primary sensor level, according to Wikibon analyst and Chief Technology Officer David Floyer (@dfloyer, pictured, right).
“The devices themselves will be done by extreme specialists in those areas,” Floyer said. “They will need a set of very deep technology understanding of the devices themselves, the sensors themselves, the AI software relevant to that.”
Raw data ingested at the edge device will then go onto the secondary level. The primary data may branch off here into several secondary domains for use by different business units. The tertiary level is where all a business’ data is collected. The intelligent edge models ultimately require training by huge data sets that cannot fit on devices.
“So you’re doing optimizations of the model, and those flow back as context to inform both the gateway and the edge,” Gilbert explained.
About 80 percent of data at the final level is unstructured; this may include content such as Twitter messages. Processing this will require massive compute power, artificial intelligence and deep learning. Pairing the insights from the tertiary level with the sensor data will ultimately result in the best models that make the most accurate real-time decisions.
“Each level will have its own type of AI. So you’ve got AI at the edge, which is to produce a specific result, and then there’s AI to optimize at the secondary level, and then the AI optimize bigger things at the tertiary level,” Floyer said.
How much data should go back to the cloud or data center, how much should stay on the device, and where and when decisions are made remain hard questions. “If you’re pushing it all back to the tertiary level, there’s going to be a lot of latency, and the whole idea with [Complex Event Processing] was right now,” Raden said.
Sending all data back to the cloud will not be cost effective either. Floyer’s Wikibon research indicates that over a three-year period, a hybrid model, which keeps more data at the edge, costs 85 percent less than one that sends it all to cloud. Within 10 years, 99 percent of internet of things data will live and expire at the edge, Floyer predicted.
“These are not technology problems by themselves,” said Peter Burris (@plburris, pictured, left), head of Wikibon research. Ultimately, businesses will have to balance technology, latency, cost and value, he added.
“The business is going to have to decide: How much data do we have to capture off the edge to ensure that we have the kinds of models we want so that we can realize the specificity of actions and behaviors that we want in our business?” he said.
The business considerations will have to inform the design of the edge systems, Burris concluded. “It’s not going to be, throw a $300 microprocessor at everything,” he stated.
Watch the complete Wikibon analyst meeting below:
THANK YOU