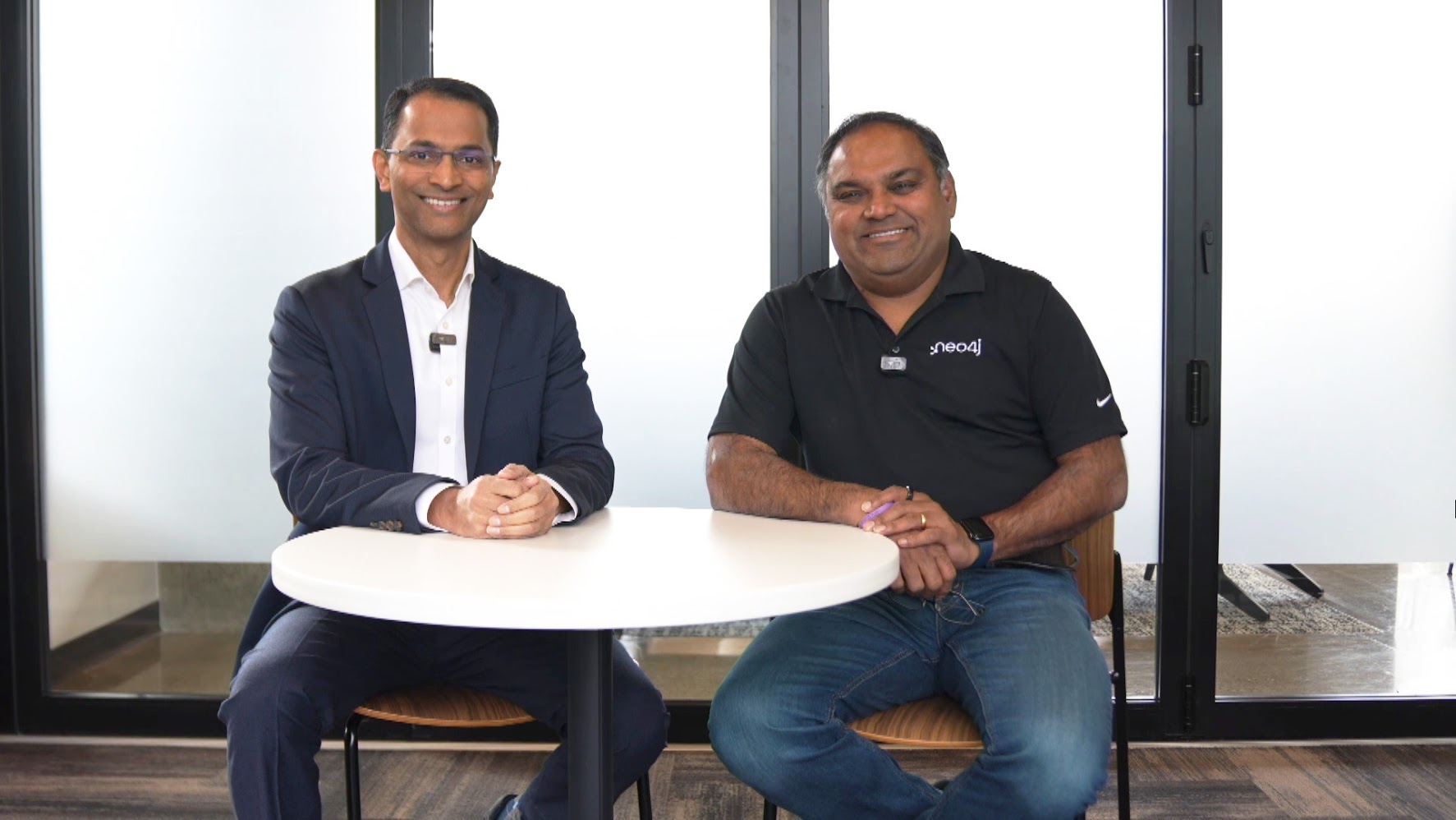
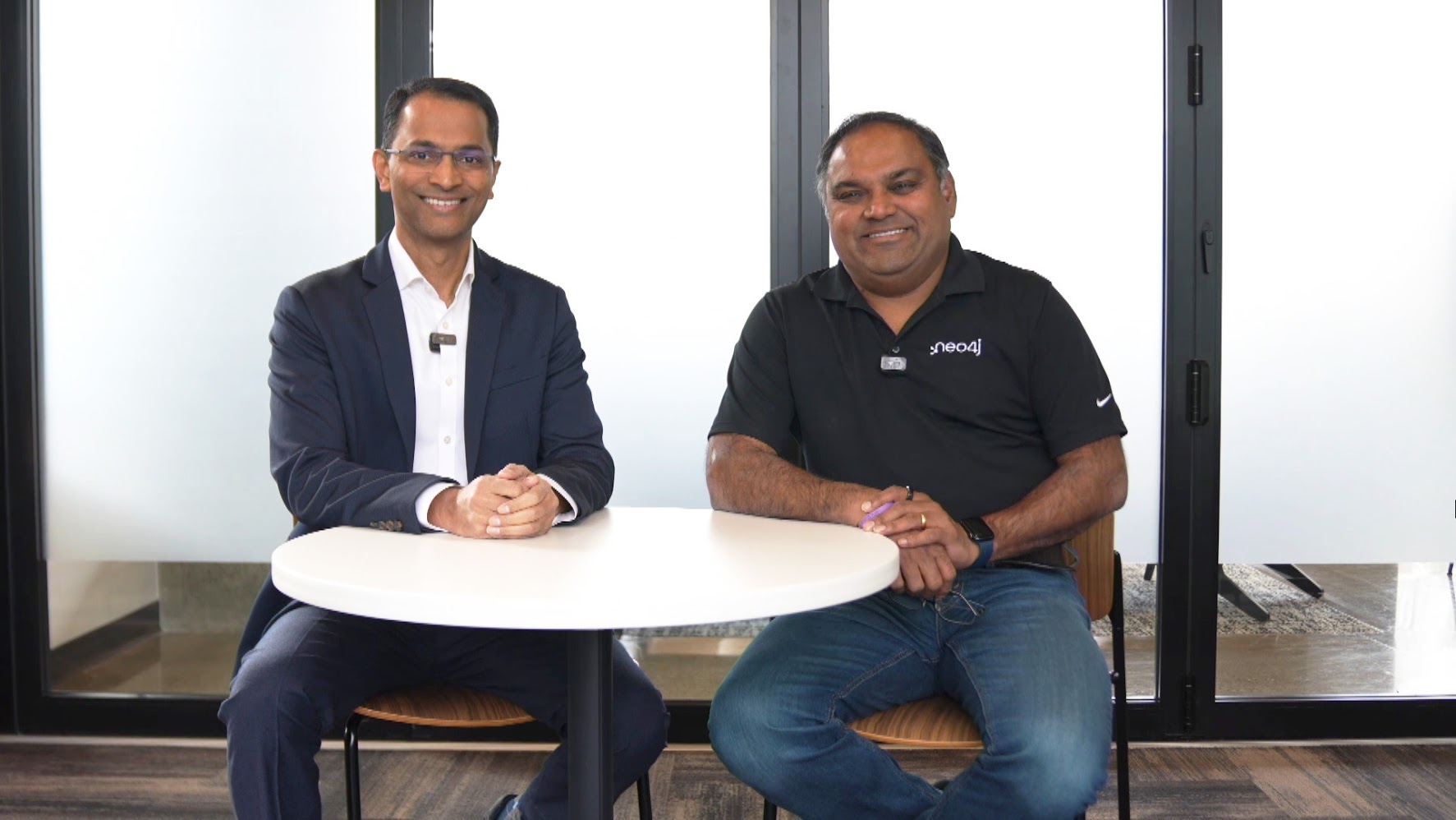
The rise of generative artificial intelligence has sparked renewed interest in knowledge graphs, and this has become a major opportunity for Persistent Systems Ltd. and Neo4j Inc.
Knowledge graphs use graph-structured data models to describe entities and their relationships, an important factor in enabling large language models to provide the correct level of insight in generative AI applications. Persistent Systems and Neo4j are collaborating on a set of initiatives surrounding graph databases and their use in enterprise AI to leverage large data stores.
“The opportunity for enterprises now, as large language models and generative AI technologies are coming in, is to break [down] the data silos within organizations,” said Sudhir Hasbe (pictured, right), chief product officer of Neo4j Inc. “Having this knowledge graph that can represent all the knowledge out of all these systems into a single framework helps LLMs get the right information. We are seeing this interest in knowledge graphs to be the backbone for a lot of generative AI applications that people want to build.”
Hasbe spoke with theCUBE Research analyst John Furrier during an exclusive broadcast on theCUBE, SiliconANGLE Media’s livestreaming studio. He was joined by Pandurang Kamat (left), chief technology officer of Persistent Systems, and they discussed how knowledge graphs are becoming a vital part of the AI ecosystem. (* Disclosure below.)
Knowledge graphs can provide a framework for identifying patterns in data and understanding the context of questions being asked. This becomes more important as enterprises seek to drive insight from multiple data sources using generative AI tools.
“What graph databases and knowledge graphs bring to the table is they allow us to … pull in data from disparate data sources within the enterprise, build out those relationships so that you can pull a deeper context and insight to give to the LLM,” Kamat said. “The other is finding themes and reasoning across data sets and volumes of data that’s inherently harder for an LLM to do by itself. Graph databases and knowledge graphs have been a big boon in driving up the quality, accuracy and contextual relevance of the answer that we are giving with these AI services.”
Large language models can have limitations because they are largely dependent on the information used in training them. Hasbe cited the example of an automobile where an engine oil light might suddenly appear on the dashboard. What does the light mean?
“Large language models were never trained for it … in this scenario, you also have the unstructured parts manuals along with the engine and its build of materials,” Hasbe said. “You convert all of that into a knowledge graph that can then be accessed and you get all facts from there. You still use large language models for explaining the answer to the technician, but the facts have to come from the knowledge graph. Combine both of them and you get the best of both worlds.”
Companies such as Neo4j and Persistent Systems are finding that knowledge graphs can provide enhancements for multiple technology solutions. One of these involves vector databases, which can index and store embedded data for fast retrieval and search.
“Vector databases are a way in which you bring structured information, put it in a mathematically representative form that makes it easier for your application to retrieve, and bring the right context for the LLM to reason on it,” Kamat explained. “Knowledge graphs provide a much richer relationship between the various key entities and how they tie to each other and allow to you infer second order, third order, fourth order insights that are not intuitively captured in a vector database.”
There is another advantage for knowledge graphs in an area of increasing importance to generative AI users. The technology can provide a level of transparency for AI at a time when there remains a great deal of mystery around how models actually work.
“[LLMs] are inherently a little less explainable than where the industry has been trying to go with building explainability into the inference AI provides,” Kamat said. “Knowledge graphs provide that traceability, the visibility that you can give even to the end user depending on the user experience you’re creating to build further trust into the system.”
Persistent Systems and Neo4j are collaborating on a project to streamline the integration of unstructured data into knowledge graphs, according to Hasbe. It is part of a broader effort to deal with the scale and complexity of data management that adoption of generative AI has brought to the forefront.
“We are working on tools that will be accessible to our customers so you can take an existing knowledge graph or build a new knowledge graph from unstructured data, and we can automatically reduce the time to value significantly for customers for any kind of use case they want to enable,” Hasbe said. “The scale of data is only to grow bigger in organizations. So, building technology that allows you to represent that complexity in the form of knowledge graphs, powered by Neo4j, is a big focus.”
Here is the complete video interview with Kamat and Hasbe:
(* Disclosure: Persistent Systems Ltd. sponsored this segment of theCUBE. Neither Persistent Systems nor other sponsors have editorial control over content on theCUBE or SiliconANGLE.)
THANK YOU