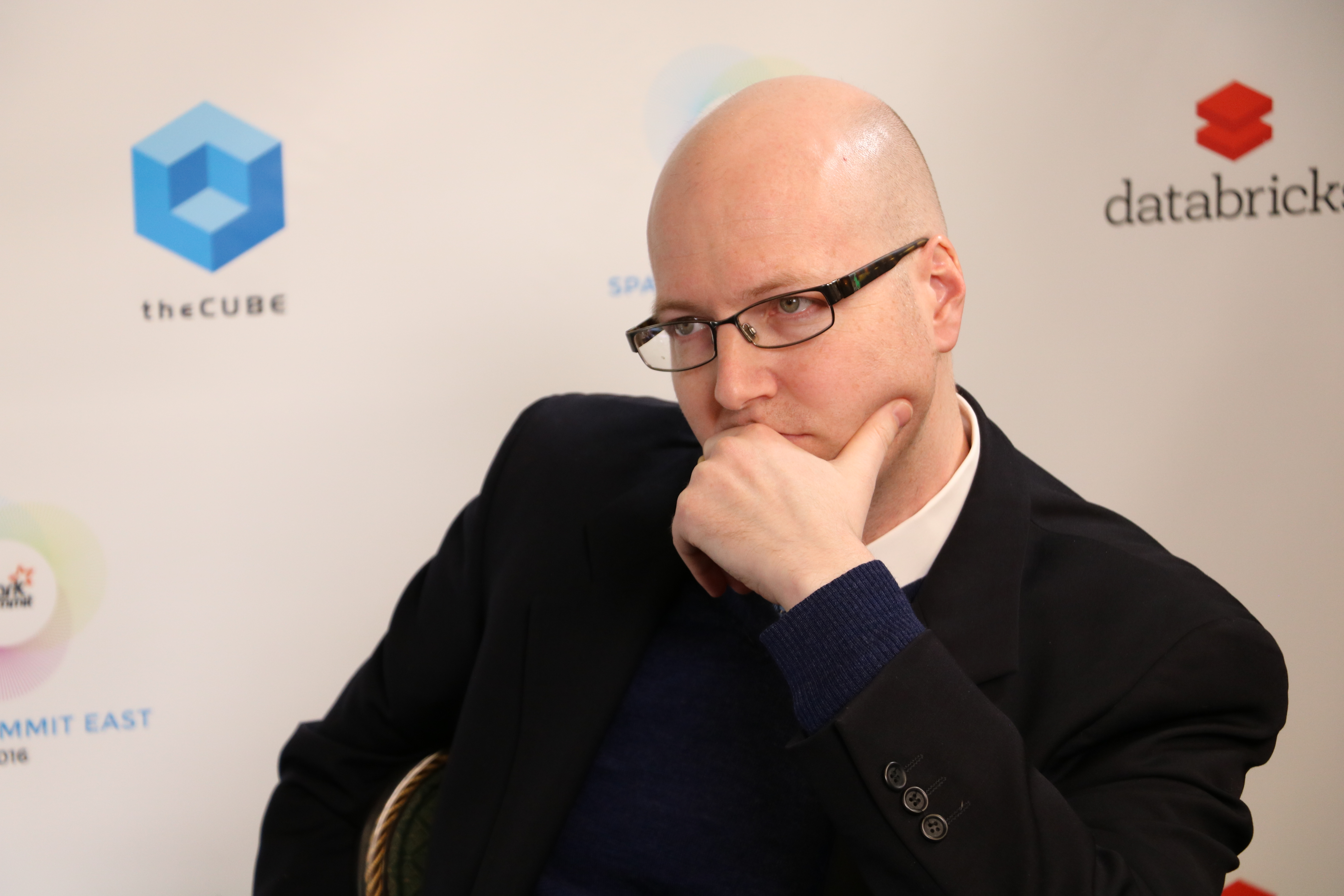
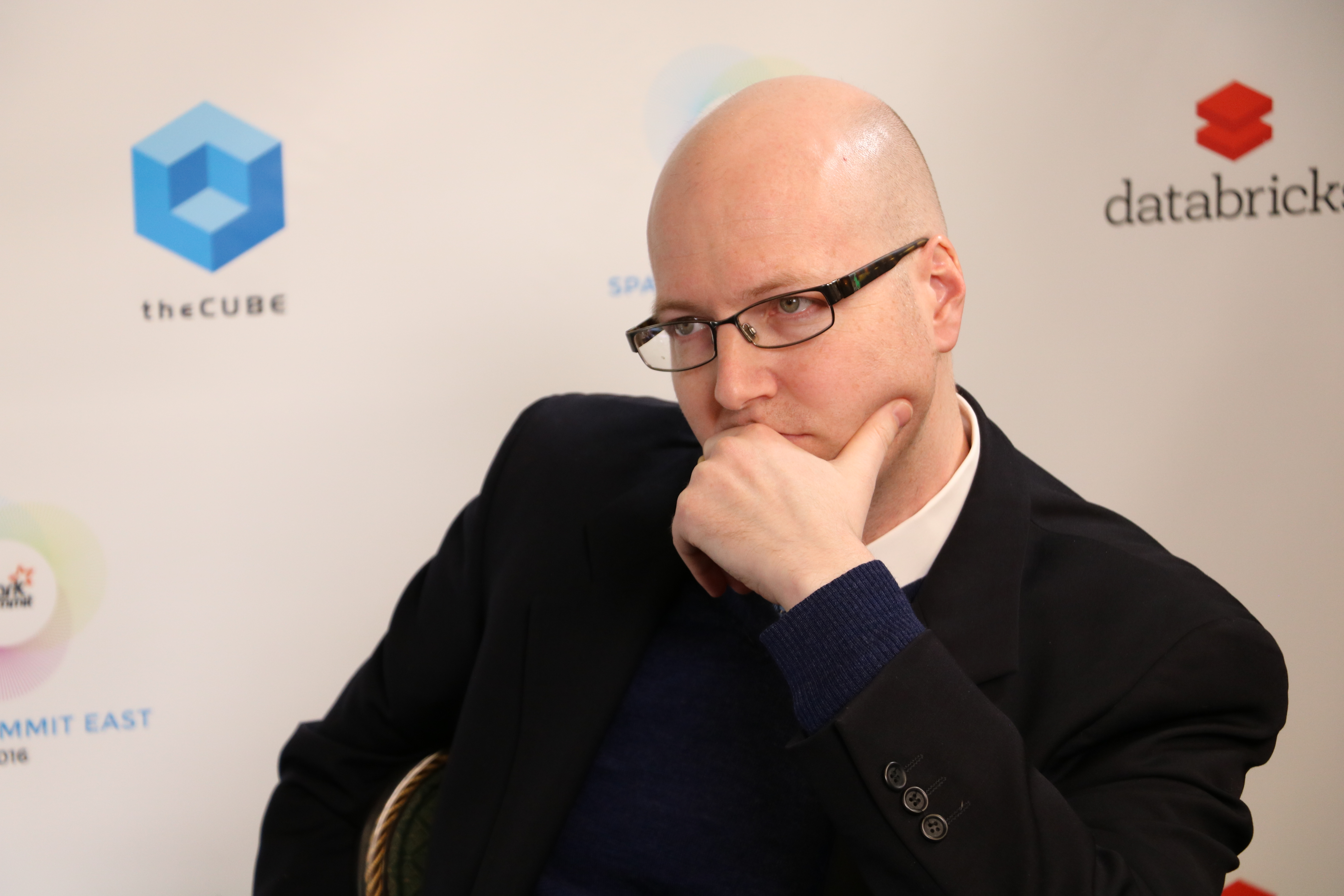
There is a lot of confusion around about Spark and Hadoop, but Matthew Hunt, head of Big Data at Bloomberg LP, thinks this is only due to a lack of understanding.
In an interview at Spark Summit East 2016 at the New York Hilton Midtown in NYC, Hunt talked with Jeff Frick and George Gilbert, cohosts of theCUBE from the SiliconANGLE Media team, to demystify the complexities of working with Spark and Hadoop, as well as provide an analysis of how Spark and Hadoop fit within the market.
In a conversation that delved deep into the mechanics of Big Data frameworks, Hunt explained the differences between Spark and Hadoop, answering where they come from, where they are going, what they do today and how they fit together.
For example, Hadoop was created to resolve a practical problem — how to download and index the web economically — by engineers rolling up their sleeves to solve real issues. As the platform grew, layers were added on top and the complexity and number of tools grew, yet the instruction set remained basic. Spark was developed with a more complicated instruction set that increased its speed yet simplified the many tools of Hadoop into one.
Hunt gave a practical example to help viewers create a mental model of Spark: When you compile a program, you write code, hit a button and the computer turns it into machine-level instructions. The same thing happens in Spark — it has an instruction set under the hood where whatever you are writing in is transformed.
Gilbert summarized this as Spark “taking language constructs and making it performant.”
People assume what will be in a fast computation engine, but they are often not accurate and this causes confusion, said Hunt. “There is a mental model shift, and there are pieces that haven’t come together yet to make that happen.”
Watch the full video interview below, and be sure to check out more of SiliconANGLE and theCUBE’s coverage of Spark Summit East 2016. You can also join in on the conversation by CrowdChatting with theCUBE hosts.
THANK YOU