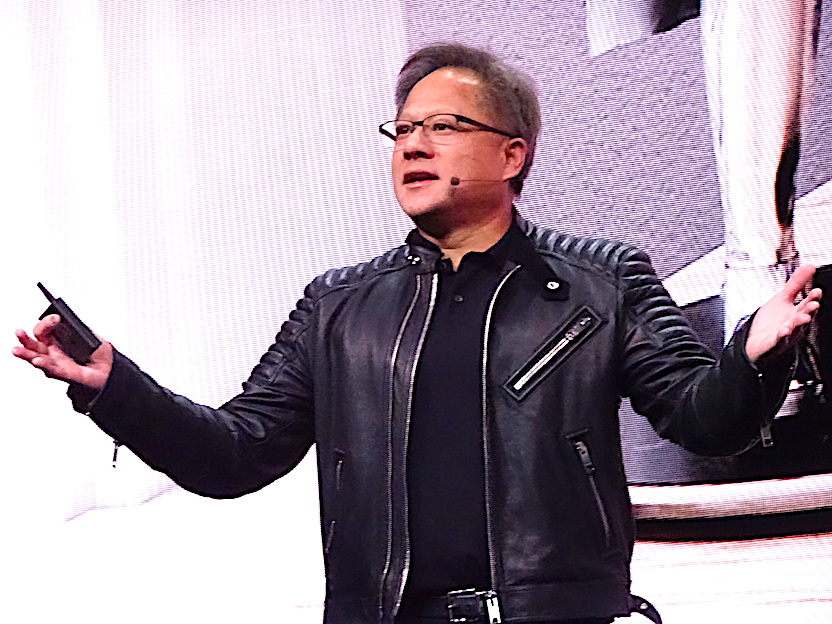
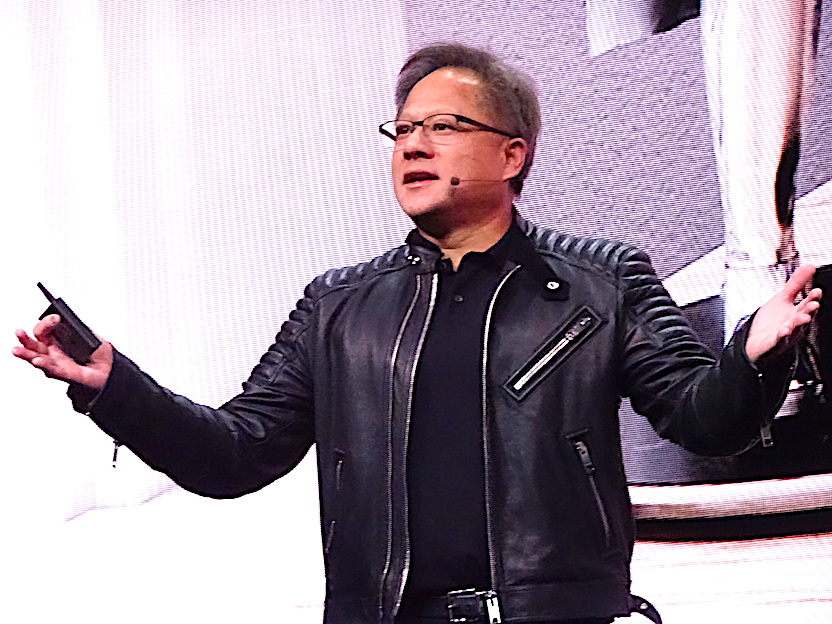
Nvidia Corp. has been riding a surging business in recent years as its graphics chips turned out to work really well for a branch of artificial intelligence that has led to breakthroughs in speech and image recognition and self-driving cars. Now, it’s aiming to stay atop what has become a scramble to provide everything from chips to cloud services tuned for deep learning neural networks.
To that end, the company today debuted a raft of new products and services at its GPU Technology Conference in its hometown of San Jose, California, all focused around its graphics processing unit chips and related software. The new products both boost the power of its chips and computers and the cloud services using them and also extend deep learning to potentially billions more products, from self-driving cars to consumer electronics to mobile phones.
“The number of people using GPU computing has grown exponentially,” said Jensen Huang (pictured), Nvidia’s founder and chief executive. “We’re at the tipping point.”
One thing notably absent in all these announcements was a new generation of GPUs, often a staple of the conference. Nvidia introduced its Tesla V100 chip based on its Volta technology at the same conference held last May. But it was only last November that its most powerful chip was available through all the major high-performance computing and cloud computing providers, and momentum is still building.
Huang did announce what he called the world’s largest GPU, the Volta-based Quadro GV100 (below). It has 16 Tesla V100 chip cores as well as more memory and a new technology to connect processing cores in the chip. It also uses a new graphics technology called RTX introduced last week at the Game Developers Conference. For the first time, Nvidia said, this enables ray tracing, the process of creating realistic images, to be done in real time. “It’s a giant leap for real-time computer graphics,” Huang said.
But the main focus, as in the last couple of years, was deep learning, and Nvidia had no lack of new technologies around the Volta chips that support the use of deep learning. For one, Huang introduced a series of improvements to Nvidia’s platform that collectively boost performance on deep learning workloads by eight times over just six months ago.
One reason is that the company has doubled the amount of memory on the V100, to 32 gigabytes, which boosts the performance on memory-constrained high-performance computing applications by half. Another reason is a new “fabric” to connect GPUs called NVSwitch, which allows up to 16 V100s to communicate very rapidly to run much larger datasets, and an updated “stack” of software.
DGX-2 (Photo: Nvidia)
Not least, there’s a new server, the DGX-2, which Nvidia said is the first single server that can deliver computing power of 2 petaflops, or quadrillion floating point operations per second. The machine, which will cost $399,000 when it’s available in the third quarter, can process the standard FAIRSeq machine translation model in two days instead of 15. “We are dramatically enhancing our platform’s performance at a pace exceeding Moore’s Law,” the doubling of chip performance every couple of years, Huang said.
“The biggest feature in DGX-2 is the new NVSwitch,” said Patrick Moorhead, president and principal analyst at Moor Insights & Strategy. “This improves performance as well as reducing latency as the GPUs don’t need to go to main memory as much… which is a very big deal.”
Nvidia, whose chips have been used by most companies to train deep learning models for applications such as speech and image recognition, also announced new technologies for the process of running those models in action, known as “inferencing.” Ian Buck, vice president and general manager of Accelerated Computing at Nvidia, said during a press briefing Monday that GPUs are getting used more often for inferencing.
Kansas City, for instance, is using deep learning to predict with 76 percent accuracy when a pothole will appear in a road, and it expects to get to 95 percent accuracy. Pinterest Inc. uses GPU-based systems to do real-time image classification and recommendation systems. “We’re creating a new computing model,” Buck said. “It’s literally software writing software.”
To boost its presence in inferencing further, Nvidia announced a new version of its TensorRT inference software, including an integration with Google LLC’s popular TensorFlow machine learning software framework. Nvidia has also worked with Amazon Web Services Inc., Facebook Inc. and Microsoft Corp. to make sure other frameworks such as Caffe 2, MXNet CNTK, Chainer and Pytorch can be run easily on Nvidia’s platform. Not least, there’s now GPU acceleration for Kubernetes, the popular “orchestration” tool for software containers, which allow applications to run on many kinds of computers, operating systems and clouds.
Nvidia aims to bring deep learning to “internet of things” devices from cars to smartphones. To that end, it has forged a partnership with Arm Holdings, which makes energy-efficient chips used mostly in mobile devices, to integrate Nvidia’s open-source Deep Learning Accelerator architecture into Arm’s Project Trillium machine learning processors.
“This announcement could enable Nvidia machine learning tech to be in even smaller IoT devices like home automation and even smartphones,” Moorhead said. “Partnering with Arm doesn’t guarantee Nvidia NVDLA success at the ‘very small edge,’ but increases its chances greatly.”
Not least, Nvidia is tackling the critical task of improving self-driving cars, one of which from Uber Technologies Inc. last week killed a pedestrian in Arizona in an accident that appeared preventable. Danny Shapiro, Nvidia’s senior director of automotive, noted that Rand Corp. believes billions of miles of training will be needed to make self-driving cars safe. The only way to do that in a reasonable time frame, he said, is through fast, hyperrealistic simulation.
That’s what Nvidia announced today. DRIVE Constellation is an autonomous vehicle simulator using virtual reality that simulates collections of cameras, LiDAR and radar used for car operations. Data from that simulator is fed into a second server, DRIVE Pegasus, in the car itself for processing, and the driving commands are sent back to Constellation 30 times a second to validate that the vehicle is operating properly. A wide variety of rarely encountered “weird” driving situations can be simulated over and over to improve the system.
Photo: Nvidia
That could be especially key since Nvidia announced today that it’s temporarily suspending self-driving car tests in response to the recent accident death, following Uber’s own suspension of testing in Arizona. Hundreds of companies working in autonomous cars use Nvidia’s DRIVE technology.
“DRIVE Constellation makes so much sense as Nvidia can provide as realistic images as one would want given their background in games and movie creation,” Moorhead said. “I like that car companies can simulate tens of billions of miles driven versus actually having to do it in a real car potentially endangering lives.”
Added up, the AI-related announcements appear to keep Nvidia at the cutting edge of chips and software needed for the training and running of machine learning models. That’s despite more aggressive competition lately. Intel Corp., for instance, in November announced a surprise partnership with longtime rival Advanced Micro Devices Inc. to make chips for high-end laptop personal computers that combines Intel’s central processing unit with AMD’s GPU. And Google has made hay in its cloud with services that use its own Tensor Processing Unit chips that are specially tuned for machine learning.
Still, Buck noted that because it’s so early in the evolution of machine learning and new models are constantly emerging, “having a programmable program to deal with those is really important.” So far, he’s right, according to Karl Freund, consulting lead for high-performance computing and deep learning at Moor Insights & Strategy.
“I don’t think any of the competitors is getting any meaningful traction at this point,” Freund said. “This could and probably will change, but all their efforts to date look pretty weak when compared to Nvidia.”
And there’s no slowdown apparent in the uptake of machine learning. “Deep Machine Learning is front and center for every enterprise application and for every consumer application, and hence investments in Nvidia GPUs is not stopping anytime soon,” said Trip Chowdhry, an analyst with Global Equities Research.
THANK YOU