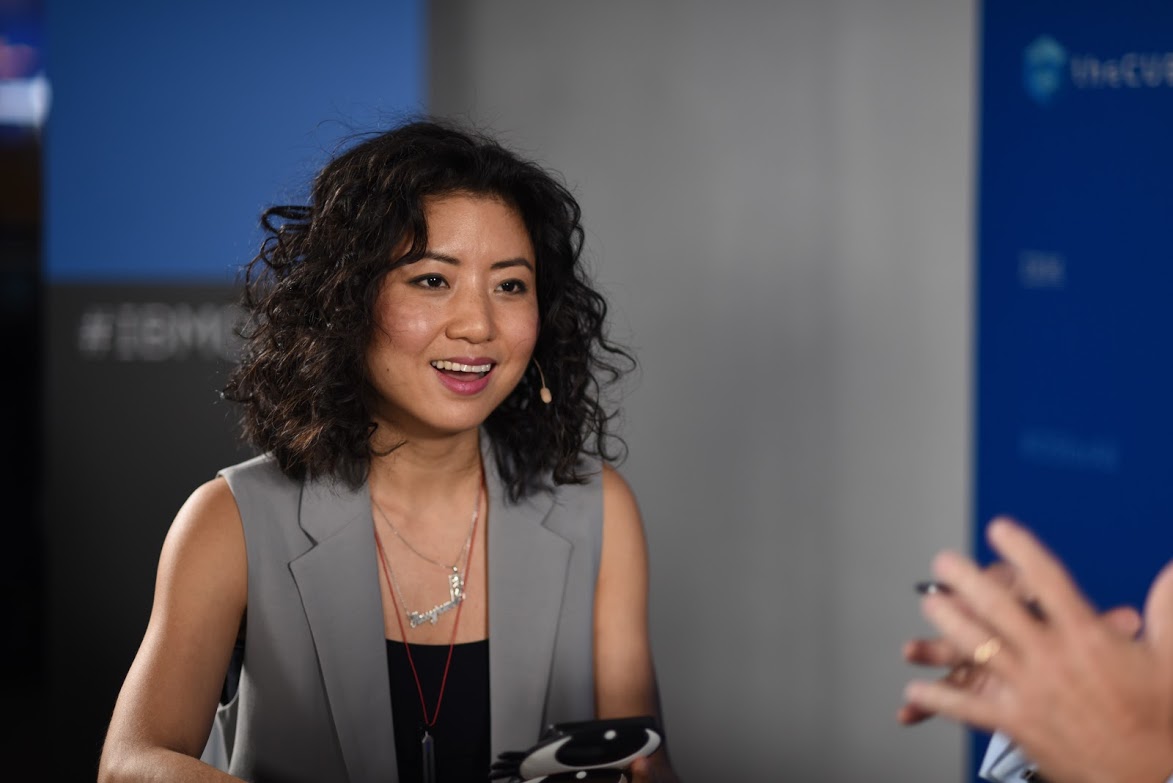
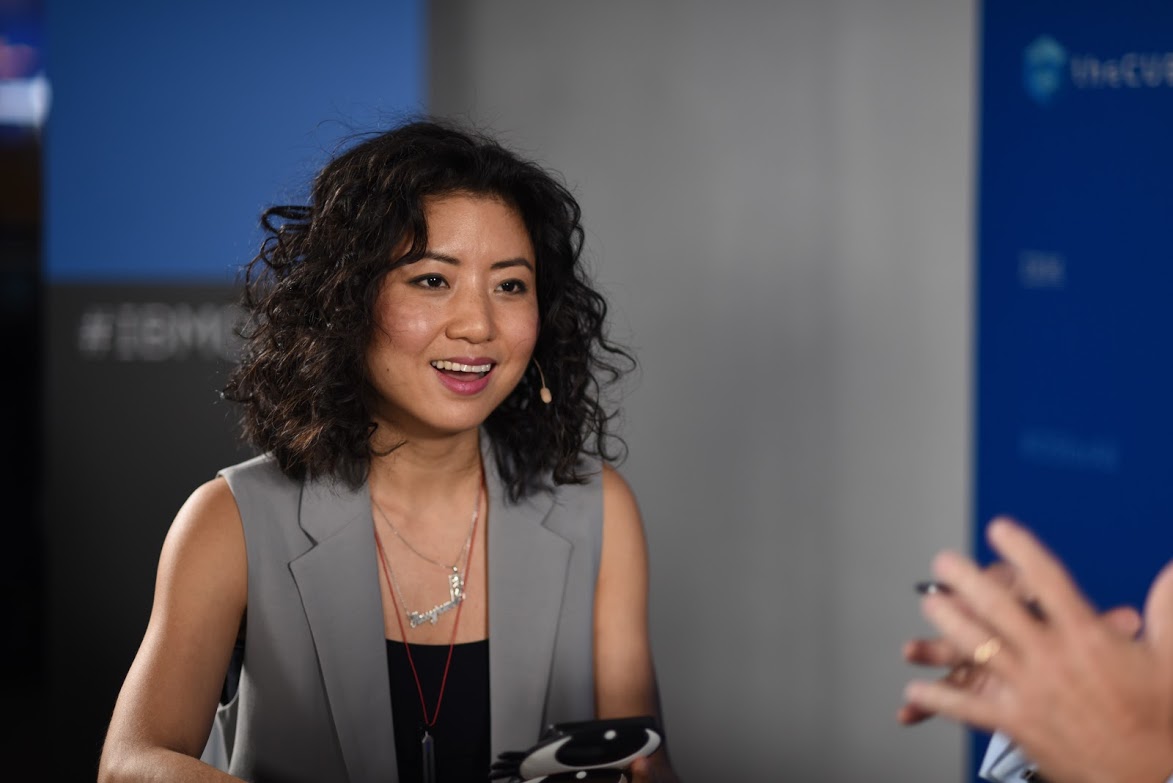
Data analytics is a numbers game, right? Adding more data points solves inaccurate algorithm outputs. Actually, no. Outputs will not predict human behavior until inputs are as complex, unexpected and sometimes as contradictory as humans themselves.
“Customers are really the most unpredictable, the most unknown, and the most difficult-to-quantify thing for any business,” said Tricia Wang (pictured), ethnographer and co-founder of Sudden Compass, a New York City-based consultancy that helps companies figure out why their best efforts in quantitative data analytics fall short of expectations.
It’s crucial to marry big data with “thick data,” which is difficult to quantify but may hone in closer to consumers, according to Wang. Ye olde marketing data variables like education and income are so-so predictors of consumer behavior, Wang — a self-described “global tech ethnographer” — said in an interview during the IBM Data Science for All event in NYC She spoke with Dave Vellante (@dvellante) and John Walls (@JohnWalls21), co-hosts of theCUBE, SiliconANGLE Media’s mobile livestreaming studio. (* Disclosure below.)
“The new networked customer of today has multiple identities and is better understood when in relationship to other people,” Wang said. Quantitative data science is indispensable for sca; however, thick data takes individual consumers’ temperature more precisely and offers depth. “That’s why you need to combine both to be able to make effective decisions,” she added.
This week, theCUBE spotlights Tricia Wang in our Guest of the Week feature.
Clearly, some ingredient is missing in the data analytics stew at most companies. Gartner Inc. researchers have predicted that 60 percent of big data projects begun this year will fail. This explains the data software and employee shopping sprees now common in enterprises. Seeking a magic wand in a software tool or outside expertise will only get a company part of the way to success, according to Wang. At the last mile, it’s an inside job.
“You’ve hired the right expert; you have bought the right tools — but we now need to make sure that we’re creating data literacy among decision makers,” Wang said. The data-literate are able to tell stories. These stories may combine quantitative big data with some unquantifiable observation from real life.
Rendering qualitative data into a form that can hold its own can be tricky. Social media content like tweets or venue reviews are qualitative data that may be juiced into some definite or quantitative insight. Supporting data literacy efforts, Yelp Inc. has opened its massive store of reviews to technology students in its Yelp Dataset Challenge. The user-generated reviews site awards researchers who find innovative ways to use the data in natural language processing and sentiment analysis, among other things.
“Some decisions need to be made (which can be subjective) when deciding how to transform the qualitative data into quantitative form,” wrote Dr. Kirk Borne, principal data scientist at Booz Allen Hamilton, in a blog post for MapR Technologies Inc. “That is a challenge, but it is also a rich opportunity — there are far more subtleties and intricacies in language that we can use to extract deeper understanding and finer shades of meaning from our qualitative data sources about our customers, employees and partners.”
Sometimes qualitative data is best in the raw. Working as an ethnographer for Nokia Corp. in 2009, Wang experienced the predictive power of unquantifiable data first hand. In her fieldwork studying emerging customer trends in China, it soon became clear that flip phones were not long for this world.
“Look, your company’s about to go out of business, because people don’t want to buy your feature phones anymore. They’re going to want to buy smartphones,” Wang stated. But with no quantitative data in hand, she had a tough time capturing the attention of the corporation’s data scientists. “Back then, they were saying, ‘No, we don’t even consider your data set to be worthwhile to even look at,” she said.
This type of on-the-ground intel can be a huge differentiator and potentially a life-saver for companies. But it is not likely to show up in quantitative data and surveys, according to Wang. “Big data’s great if you want to optimize your supply chain and systems that are very contained and quantifiable,” she said. This approach may result in one to five percent growth; of course, sensible executives will take that if they can get it, Wang added. But more robust growth and the predictive insight that ensures longevity in a tumultuous market demands more.
“You cannot just rely on your quantitative data to tell you how to do that. You actually need thick data for discovery. You need to find the unknown,” Wang said.
It’s not necessary to hire a Ph.D. anthropologist skilled in studying a particular consumer base (even if he or she exits). Customer ethnography, or the qualitative analysis of customers, can be taught, according to Wang. Individuals trained in this way need to hunker down with the data scientists and mesh their insights. “The problem is we don’t teach these skills. We’re not graduating data scientists or ethnographers who even want to talk to each other. In fact, each side thinks the other side is useless,” she said.
This may be changing thanks to the efforts of chief information officers who see the value of cross-pollinating. “I think the new CIO is on the cutting edge,” she said.
Wang recently spoke at Constellation Research’s Connected Enterprise 2017 conference. The CIOs and chief data officers attending clearly wanted to push the envelope on innovation with more qualitative analytic work, she explained.
Even heavily hyped artificial intelligence will not replace human quantitative data analysis, Yang stated. “Clearly, the best AI systems will be integrated with human intelligence, and we need a human training the data with machine learning systems,” she said.
Despite vendors’ claims, their AI products cannot really automate any but the most rudimentary decisions, Wang believes. Over-reliance on data algorithms caused the marketing misfires at Target Corp. and other companies, she added.
“We’re going to see more AI or ML disasters when their data models are really far from their actual human models,” Wang concluded.
Watch the complete video interview below, and be sure to check out more of SiliconANGLE’s and theCUBE’s coverage of IBM Data Science for All. (* Disclosure: TheCUBE is a paid media partner for the IBM Data Science for All event. Neither IBM, the event sponsor, nor other sponsors have editorial control over content on theCUBE or SiliconANGLE.)
THANK YOU