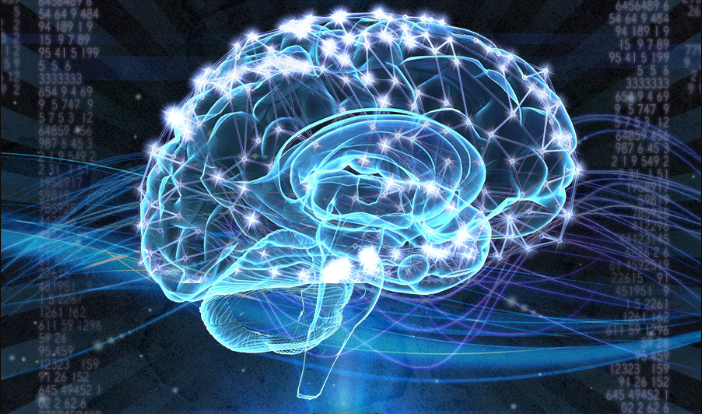
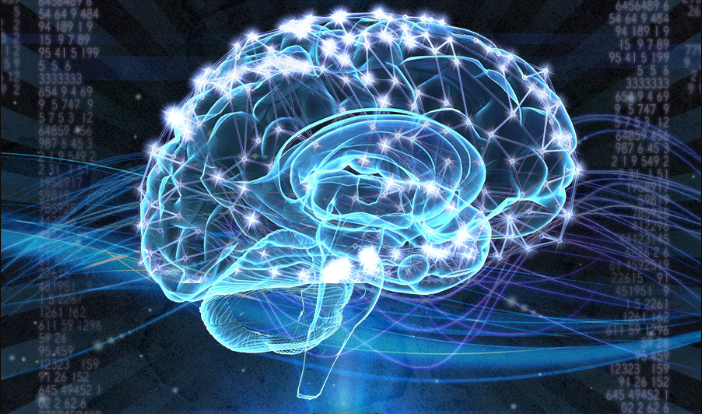
Large-scale data gathering and quantum leaps in processing power have set the table for major advancement in artificial intelligence. Yet there’s a growing body of evidence that the field of AI is poised to move into a whole new dimension, one where AI not only imagines the real world, but can begin to make accurate decisions on what’s real and important, what’s not — and thus predict what’s coming next.
“Computers are really good at memorization,” Carl Vondrick, research scientist at Google LLC, said during a presentation at the Re-Work Deep Learning Summit in San Francisco Thursday. “The problem is teaching them how to forget.”
Vondrick’s research has focused on one of the most vexing challenges in today’s online world: how to make use of the massive database of unlabeled videos that clog nearly every corner of the web. It’s one thing to swoon over a cute baby or funny cat video. It’s another to learn from it.
The Google research team decided the best approach was to use millions of unlabeled video hours to train deep learning neural networks to reach a better understanding of the world. By drawing on the vast cache of freely available footage, the AI-enabled network could correctly interpret not only what it saw, but what would happen next.
In examples presented at the conference, Vondrick showed videos of people approaching each other and then the network decided, mostly correctly, what action would occur as a result. A hug, handshake or “high five” was often the behavior based on the human interaction captured on video.
The deep learning research is important because growing dependence on robots will demand that the machines be able to interpret human actions as observed. If a human reaches for a doorknob, it would be highly inconvenient if the robot decides to slam the door.
Intriguingly, the Google researchers have been extending the deep learning model to include sound as well. Summit attendees heard a clip of people singing “happy birthday” and as the video image was revealed, it showed that the network correctly predicted there would be an image of a candle in the segment. At 74 percent accuracy, deep learning systems are coming along well at predicting action, getting closer to human rates approximately 10 percentage points higher, according to Vondrick.
“This task is still pretty hard and we don’t always get it right,” Vondrick admitted.
Vondrick’s research is based on a methodology known as adversarial learning, which essentially pits two networks in competition with each other. One network generates real images and the other is tasked with analyzing them and rendering a decision on whether they are genuine or fake. This technique has also been recently employed by Ian Goodfellow, staff research scientist at Google Brain, who has become a leading authority in “generative adversarial networks” or GANs.
In Goodfellow’s work, GANs create photos and sounds of the real world. “GANs are generative models based on game theory,” Goodfellow explained. “They open the door to a wide range of engineering tasks.”
These tasks encompass a variety of deep learning models where machines can be asked to turn a brown horse into a zebra. In a video clip shown at the gathering on Thursday, a horse prancing in a corral becomes perfectly represented with zebra stripes, complete with browner grass in the background since the computer sourced its zebra from images taken in the drier African savanna.
Perhaps even more significant are advances in deep learning that are training computing models to understand human context. At the Allen Institute for AI, researchers are training large scale language models by reading lots of unlabeled text data from online databases.
The key approach here incorporates embeddings from language models or ELMo representations. “ELMo representations are contextual, they depend on the entire sentence in which they are used,” said Matthew Peters, research scientist at the Allen Institute.
In a sample presented during the conference, Peters showed how this technique allowed networks to correctly decipher the intent behind a simple word such as “play,” which can have multiple meanings depending on how it is used in a sentence. A “three-point play” can mean something entirely different from “representatives who play to the party base.” By training on full sentences instead of limited word definitions, computers are learning to get this right.
AI is being extended to mobile devices as well. Facebook’s AI Camera Team has developed a new technology, Mask R-CNN2Go, that detects body poses and can accurately separate an image from its background. This is not an easy problem to solve since detecting body movements in real time is a chaotic process at best. Dress, movement and the nearby presence of other people or objects all interfere. The AI network must be able ultimately to de-identify other parts of the image in order to detect and track the body pose accurately. In other words, it must learn to forget.
Facebook AI Research or FAIR recently published the Mask R-CNN platform, which is based on open-source code, according to Andrew Tulloch, research engineer at Facebook Inc. The potential for using this application among Facebook’s vast community of mobile users offers promise for the future. “There’s a huge opportunity here,” said Tulloch.
Just how far is deep learning going to reach? At the Consumer Electronics Show held earlier this month in Las Vegas, attendees were pitched on everything from an AI-controlled pet toy for cats to an AI-powered standing desk. Even pop culture icon Justin Timberlake has joined the party with the recent release of a video that incorporates an AI theme.
“Deep learning is now almost a commodity,” said Clement Farabet, vice president of AI infrastructure at Nvidia Corp. That suggests we’ve only seen the beginning of how deeply AI technology will change our daily lives.
THANK YOU