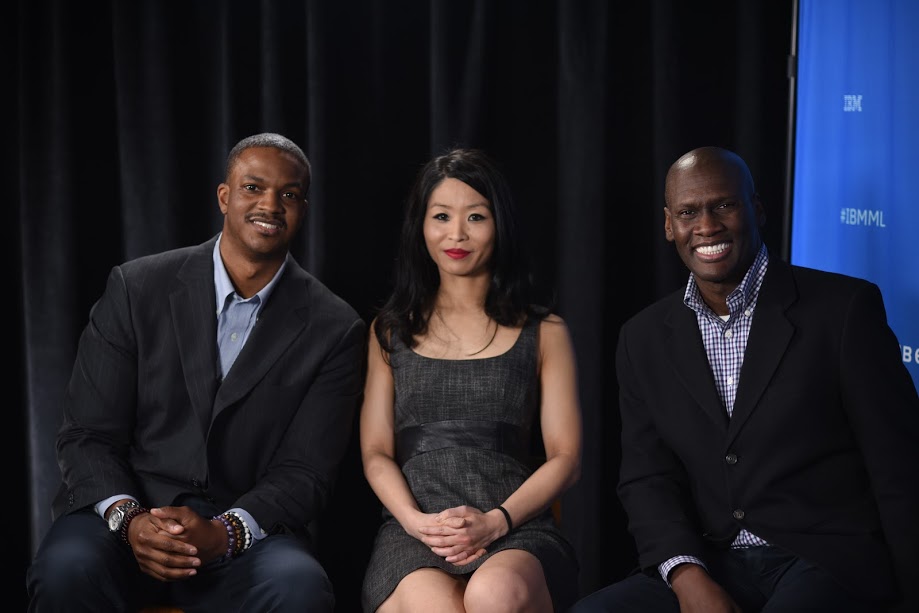
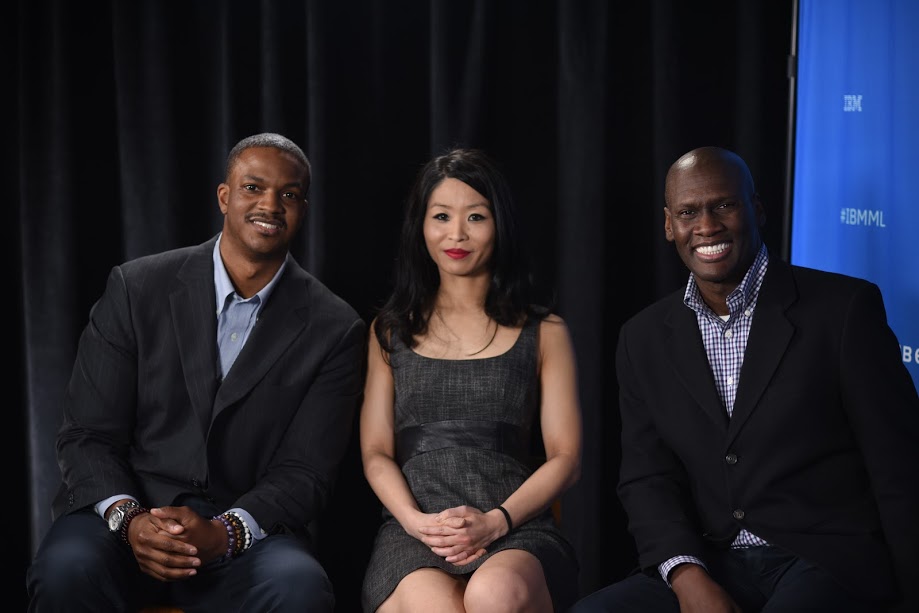
Artificial intelligence is creeping inevitably into daily life, and there is no shortage of opinions about what that may mean for the future.
Russian President Vladimir Putin issued a prediction last fall that whoever emerges as the leader in AI will rule the world. Tesla Inc. co-founder and Chief Executive Elon Musk has suggested in recent months that the threat from North Korea is a mere stroll in the park compared to the havoc that AI could bring upon civilization. And Masayoshi Son, chief executive officer of Japan’s SoftBank Group Corp., is on record as saying that the time when computers and AI will surpass mankind is nearly upon us.
Despite all of the noise surrounding AI, there are still a few important details to be worked out, a process that remains firmly in the hands of information technology architects, researchers and data scientists who are actually building AI solutions for deployment in the enterprise. They are grappling with the challenges of big data sets, flawed training methodologies, or, in some cases, what to do when there is little data at all.
Without the right processing or the correct data source, AI still becomes a technology with great promise but disappointing results. “Without those two things, you’ll either have a lot of great data that you can’t process in time, or you’ll have a great process or a great algorithm that has no real information, so your output is useless,” said Jennifer Shin (pictured, center), founder and chief data scientist for 8 Path Solutions LLC and instructor at Columbia University. “Those are the fundamental things you really do need to have any sort of AI solution built.”
Shin spoke with Dave Vellante (@dvellante) and John Walls (@JohnWalls21), co-hosts of theCUBE, SiliconANGLE Media’s mobile livestreaming studio, during the IBM Signature Moment — Machine Learning Everywhere event in New York. She was joined in a panel discussion by Colin Sumter (pictured, left), IoT architect at CrowdMole, and Craig Brown (pictured, right), senior big data architect and data science consultant. They discussed limitations and challenges confronting AI today, the role of robotic process automation, customer use cases and future development. (* Disclosure below.)
Recent forecasts show an upward curve for AI and machine learning in the technology world. Data reported last month by Forbes showed that machine learning patents are now the third-fastest growing category of all patents issued and spending on AI and machine learning is expected to increase from $12 billion in 2017 to $57 billion by 2021.
Yet, the intelligence space is still encountering growing pains that encompass both technology issues and customer understanding of how to effectively use the new tools. Rob High, IBM Watson’s chief technology officer, recently expressed concern that the biggest challenge for machine learning today was how to train the all-important models using less data. And Google’s top AI executive, John Giannandrea, echoed his colleague’s point when he described the issues surrounding AI’s ability to recognize human intent in conversational speaking.
“There aren’t that many very sophisticated documents you can find about how to implement it in real-world conditions,” Shin explained. “They all tend to use the same core data set, a lot of these machine learning tutorials you’ll find, which is hilarious because the data set is actually very small.”
In addition to the technology challenges, there are also questions about customer readiness or adoption. Panel participants described disconnects among customers between AI’s capabilities and how they can be effectively applied for use within organizations.
“I’m in these meetings with senior executives, and we have lots of ideas on how we can bring efficiencies and some operational productivity with technology,” Brown described. “And then we get in a meeting with the data stewards and we hear, ‘What are these guys talking about? They don’t understand what’s going on at the data level and what data we have.’”
If the technological challenges and customer readiness to properly adopt AI and machine learning as critical enterprise tools can be overcome, what are the potential applications for the future? One indication of where this might be headed involves robotic process automation, or RPA. It’s basically software that imitates a human activity in fulfilling repetitive, routine tasks, and its potential for freeing humans to work on the harder technology obstacles is one attraction.
Examples of enterprise applications where RPA could be used include report creation, payroll management or bookkeeping. “The way RPA plays into machine learning and data science is that it allows you to outsource business processes to compensate for the lack of human expertise,” Sumter said. “When you start automating some of these processes, you can keep pace with the innovation in the marketplace and allow the human expertise to gradually grow into these new data science technologies.”
Some industry sectors appear farther down the AI path than others. A report released by Cisco Systems Inc. showed that security executives are investing in and becoming more reliant on AI to defend against malware attacks and other cybersecurity threats. In the sales arena, another report showed that 84 percent of marketing organizations are implementing some form of AI and machine learning in 2018, driven no doubt by 75 percent who deployed the tools and saw a 10 percent gain in sales.
Even the federal government, traditionally slow to adopt new technologies, is apparently getting into the act. New guidance for research and development priorities in fiscal 2019 recently issued by the Office of Management and Budget includes investment in machine learning and autonomous systems.
“I work with the government, and the government’s trying to change things,” Shin said. “I feel like that’s kind of a sign, because they tend to be a little bit slower than, say, private industries or private companies. They have data; they’re trying to actually put it into a system.”
Use cases in the news and stories told by the IBM Signature Moment panelists demonstrate the advances that AI has made. Yet, for every success story there is a comparable tale where a new application never made it past the drawing board. What it will take to move AI and machine learning from development to value, a process often referred to in the industry as “crossing the chasm,” will take time and hard work.
“To close that chasm, we have to figure out who needs to be in the room to effectively help us build the right understanding around the use cases,” Brown said. “There’s a number of things that we have to overcome, but if we can get past those areas in the next decade, I don’t think there’s going to be much of a limit, in my opinion, as to what the technology can do and what we can ask the machines to produce for us.”
Watch the complete video interview below, and be sure to check out more of SiliconANGLE’s and theCUBE’s coverage of the IBM Signature Moment — Machine Learning Everywhere event. (* Disclosure: TheCUBE is a paid media partner for the IBM Signature Moment — Machine Learning Everywhere event. Neither IBM, the event sponsor, nor other sponsors have editorial control over content on theCUBE or SiliconANGLE.)
THANK YOU