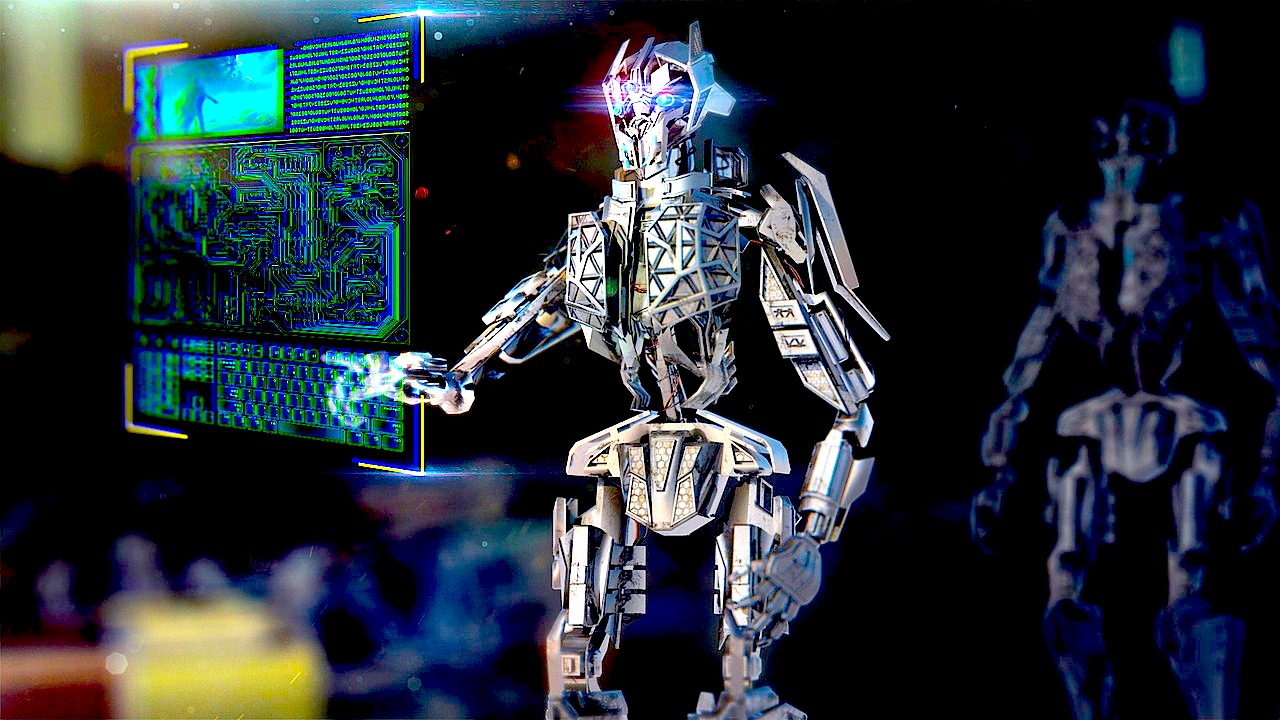
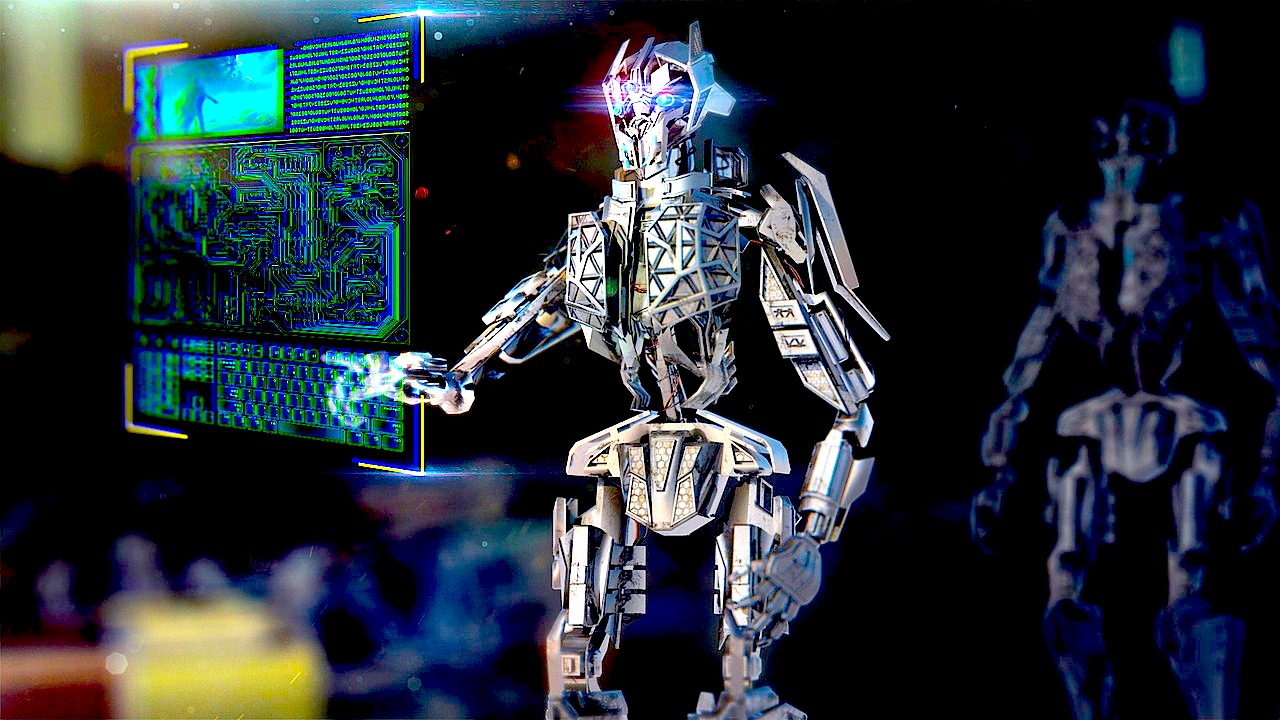
For a technology that’s decades old, artificial intelligence managed to emerge in the public imagination as one of the signature technologies of 2018 — if not always in a positive way.
On the upside, AI and its related sets of technology such as machine learning and deep learning enable now-taken-for-granted services such as speech recognition in smartphones and devices such as Amazon.com Inc.’s Echo and Google LLC’s Home. That’s on top of self-driving cars, better disease diagnoses and, less obvious but at least as impactful, more automated information technology infrastructure in the cloud and data centers.
At the same time, AI has been used to target people with fake news, discriminate against certain kinds of workers or customers, and stoked fears, if likely overblown, that machines could make most jobs obsolete before too long. Not least, some leading lights such as Tesla Inc. Chief Executive Elon Musk and the late physicist Stephen Hawking have raised concerns, still hotly debated, that runaway AI could threaten human existence.
Both for good and for ill, the coming year no doubt will see an acceleration in the use of AI and machine learning across a wide variety of products, businesses and everyday activities. Here are some predictions of what’s coming (and what’s not), along with what the experts think:
AI is already remaking the business intelligence, according to James Kobielus, lead analyst for AI, data, data science, deep learning and application development at Wikibon, SiliconANGLE’s sister market research firm. That’s allowing business users to do a lot of the analysis that once required a trained data scientist.
Then there’s robotic process automation, or software that emulates how people carry out tasks in a process, which has become one of the principal enterprise use cases for AI. AI is also becoming a critical foundation for managing information technology infrastructure, an emerging paradigm known as “AIOps.” The idea, as Kobielus has pointed out, is to make infrastructure and operations more continuously self-healing, self-managing, self-securing, self-repairing and self-optimizing.
Not least, machine learning is starting to transform software development itself, by enabling machines essentially to create applications rather than developers needing to program specific logic and rules. Look for this to become more apparent in 2019, especially as cloud computing giants offer more and more AI services. Almost half of businesses in a recent Dun & Bradstreet survey said they’re in the process of deploying AI systems and an additional 23 percent are in the planning phase.
Because of how well AI-driven services such as Amazon’s Alexa often work, there’s an assumption that AI will take over all manner of work. That’s far from the case, certainly anytime soon. McKinsey estimates that fewer than 5 percent of occupations can be entirely automated using current technology, but some 60 percent of occupations could see at least 30 percent of their activities automated.
All that means that for 2019 and several years beyond, some of the most successful applications will be those that help people do their jobs better, whether it’s clinicians parsing through MRI scans or factory workers alongside industrial robots or mortgage loan officers trying to process more prospects.
That said, some of the insistence that AI is just a tool rings a bit hollow, given that one person’s higher productivity often comes at the expense of someone else’s job. If AI is truly to benefit society without putting a lot of the people in that society out of work, AI providers and the companies that use it will need to start proving that case in 2019. And both private industry and governments will need to step up with solutions for the people who do lose jobs as a result of AI’s efficiencies.
One big knock on machine learning, especially the kinds such as deep learning that use artificial neural networks, is that the algorithms used to produce the results are a black box. You input a lot of data, and get a result whose provenance isn’t always clear — and sometimes is incorrect, such as when a self-driving car stops unexpectedly for a small, insignificant object on the road but then occasionally kills people it didn’t appear to see or comprehend correctly.
Indeed, almost half of respondents in a recent Dun & Bradstreet survey said AI explainability is an issue in their organizations, and 46 percent said they have at least some trouble figuring out how their AI systems come up with answers.
Just as bad, the data on which AI systems are trained are faulty or biased. For example, Amazon.com Inc. had to scrap its AI-driven recruitment tool in 2015 after it became apparent it was favoring men over women because it assumed that the fact that men were most of the applicants who got hired meant they were superior. This year, that realization will likely turn to more action to avoid this kind of thing — by legislation if necessary.
Although there’s only so much that can be done to open up that black box, any more than we can see into people’s brains to analyze their decisions, there’s a growing demand especially by lawmakers to shed more light on AI’s inner workings.
No doubt some tech companies that view their data and the algorithms to wrangle it as a proprietary advantage won’t be leading the way here, but some such as Google are already taking a crack at it. Governments likely will mandate some level of transparency, though it’s not clear how they can do it yet. But this will become an even bigger issue this year.
Whether it’s “deep fake” pornography, more capable AI-powered cyberattacks or a continuation of nation-states such as Russia targeting people on Facebook and other social media to influence elections, AI has just begun to show how much of a threat it can be in the wrong hands.
And like most technologies, it’s impossible to keep them out of those hands. So look for more bad stuff to emerge from the use of AI and machine learning in 2019. “There is a perfect storm of AI nasties just waiting to happen,” says Wikibon’s James Kobielus. “The human race has barely begun to work through the disruptive consequences of this bubbling cauldron of risk.” Problem is, we’ve only begun to understand the enormity of the problem, let alone find ways to ameliorate it. That job has barely begun, but a lot of attention will be paid to it this year, both in private industry and by governments around the world.
Nvidia Corp.’s graphics processing unit chips have dominated computers for doing machine learning thanks to their ability to process many operations in parallel. But that was a bit of a happy accident for the chips, which were developed originally to speed up gaming.
Now, a raft of alternative chips is about to hit the market from startups and big chipmakers such as Intel Corp. that have bought a number of those startups in recent years. Like Google’s Tensor Processing Unit chip that’s available via its cloud service, they are tuned to run machine learning algorithms purportedly even faster than GPUs. This year will show whether they can deliver on the promise.
So far, machine learning has been dominated by tech giants with a lot of data, such as Google, Amazon, Microsoft and Facebook — some of which also are among the leaders of cloud computing, so they can also sell their data-driven services to others as well. That has led to fears that small companies will fall further behind because they simply don’t have access to nearly as much data that powers modern AI.
Those fears may not be as justified as it appears, for a couple of reasons. For one, companies that lead in particular industries, products and services, such as, say, General Electric Co. in engines, have plenty of data of their own that even the Googles and Amazons don’t have. For another, there’s a growing number of open data sources as well as organizations pushing them that may well help arm the little guys. Whether they succeed will become apparent in the next year or so.
There wouldn’t even be trials of self-driving cars were it not for the machine learning that can make sense of all the data from myriad sensors and at the same time make split-second decisions on what the vehicle should do. But the technology is far from perfect, as the deaths of a couple of drivers or pedestrians in the past couple of years proves.
More than that, though, many people clearly aren’t ready for fully self-driving cars. In Arizona, some people have been vandalizing and throwing rocks at Waymo vehicles. And companies, let alone governments, aren’t even close to figuring out accident liability and many other legal issues starting to arise. As a result, despite all the testing and promise, self-driving cars as any kind of mass phenomenon remain years away.
That said, big and well-funded companies from Waymo and General Motors Corp. to Tesla, Uber Inc. and Lyft Inc. are driving full speed ahead to perfect the technology side. At the least, AI-driven vehicles may start becoming much more common for last-mile deliveries of products, either from drones or from ground-based machines. Don’t be surprised to see them rolling or flying to your doorstep in the coming year.
THANK YOU