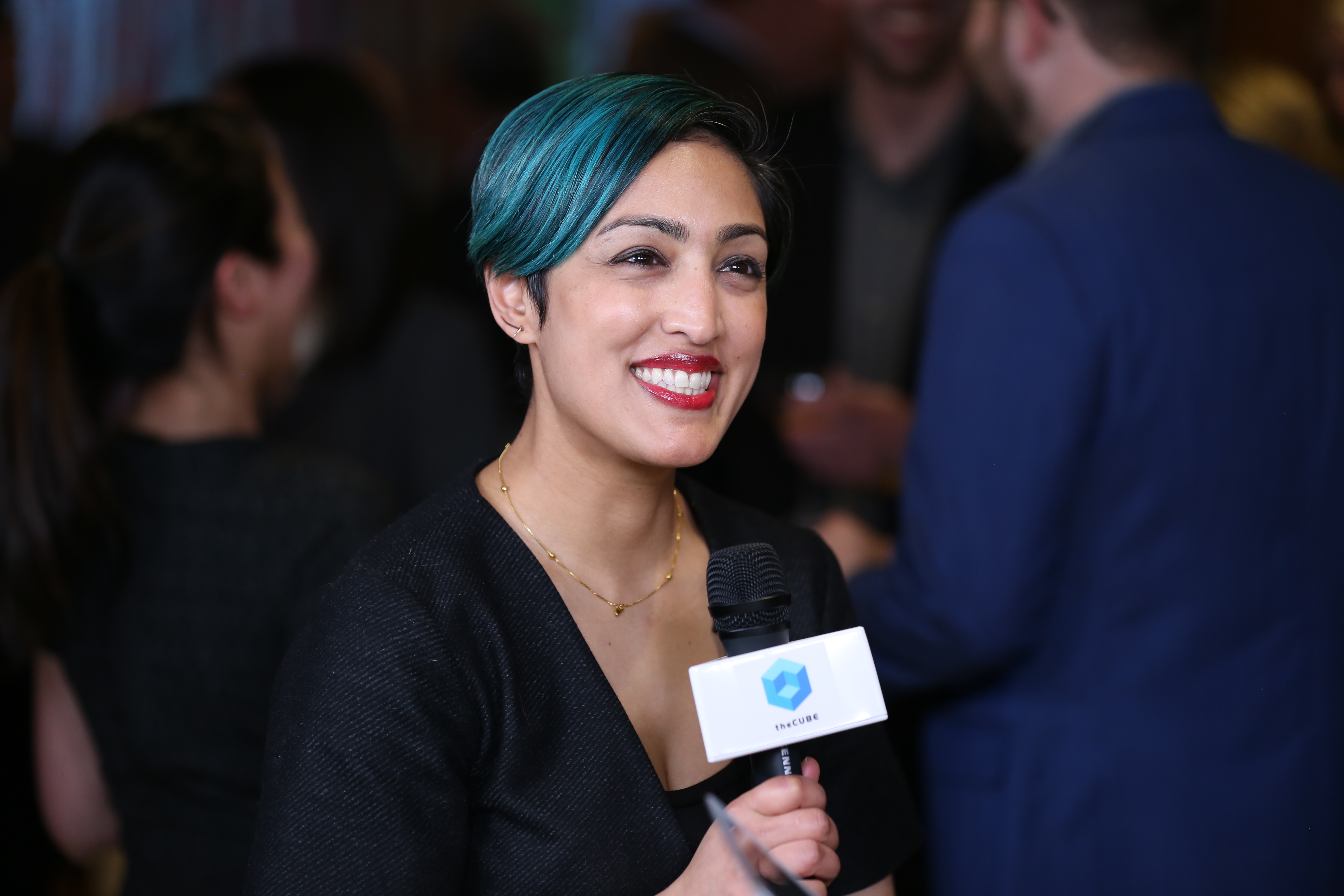
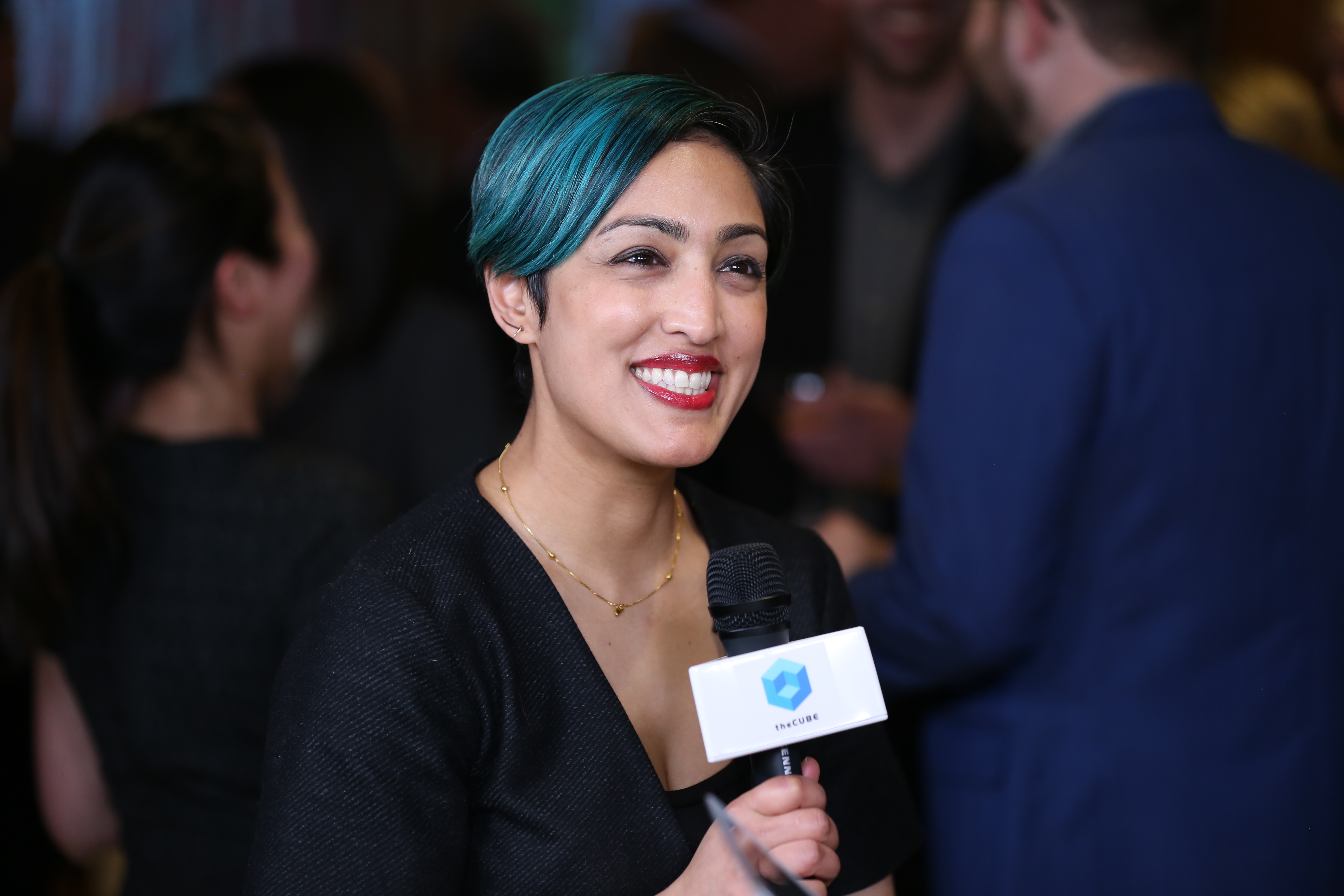
The rallying cry driving the era of digital transformation has been to “move fast and break things” in the name of rapid progress. But breaking things has consequences, and often ones that extend beyond the bottom line — especially when automated machines are the ones moving fast.
Artificial intelligence has become indispensable to a tech future outgrowing the human capacity for work. But despite the assumption that a non-human worker could offer a greater level of impartiality, the emerging reality is that AI tools developed by a tech industry with a known diversity issue can be imbued with harmful biases.
As the industry begins to see the results of its machine-learning experimentation period for better and worse, AI responsibility advocates are calling for a prioritization of ethics in innovation.
“As we think about ethics and AI, it’s not just about improving the technology; it’s about improving the society behind the technology,” said Dr. Rumman Chowdhury (pictured), global lead for responsible AI at Accenture Applied Intelligence.
Chowdhury spoke with Jeff Frick, host of theCUBE, SiliconANGLE Media’s mobile livestreaming studio, during the recent Accenture Technology Vision 2019 event in San Francisco.
This week, theCUBE spotlights Dr. Rumman Chowdhury in its Women in Tech feature.
Chowdhury comes to her work in AI by way of social science, a background that has led to an ethos intersecting humanity and tech. The data scientist is primarily concerned with the humanity that informs AI, a rare perspective in an industry propelled by an objective of constant innovation.
“When I think of AI or data science, I literally think of it as information about people meant to understand trends in human behavior,” Chowdhury said.
As AI utilizes an increasing amount of sensitive data to power the technologies that businesses and consumers use every day, conversations around its potential for inherent bias are coming to the fore. Facial recognition systems fail to identify people with darker skin. Predictive policing technologies informed by systemic racial inequalities are reinforcing sentencing determinations through algorithmic predictions of “future crimes.” Microsoft was forced to shut down its AI chatbot after the world taught it to be a conspiracy-theorizing racist.
While not every machine learning tool’s intelligence is crowdsourced by internet trolls, the potential for insidious prejudice is clear. Concerns that products and services developed by an industry with wide demographic disparities are valid, and the built-in biases trickle in from outside Silicon Valley as well.
Rep. Alexandria Ocasio-Cortez, D-NY, recently called attention to AI’s potential for unintentional discrimination. “Algorithms are still made by human beings, and those algorithms are still pegged to basic human assumptions,” she said. “If you don’t fix the bias, then you are just automating the bias.” While her comments were derided by many as paranoia, examples of AI bias are already proliferating in daily-use technologies.
According to Chowdhury, truly responsible AI will address not only the issues built into its technologies, but the social issues underlying those oversights as well. “You can have great data and a perfect model, but we come from an imperfect world. The world is not a fair place. We don’t want to codify that into our systems and processes,” she said.
As machine-learning opportunities scale with an influx of increasingly intricate data, regulations that could limit the impact of AI become a harder sell in the enterprise. Concerns that AI technologies may be operating without ethical consideration can easily become outweighed by the sense that a singular voice can’t make an impact, perpetuating a cycle of exponentially compounding built-in biases.
“Often, we feel like a cog in a machine. There’s often not enough accountability because everybody feels they’re contributing to this larger machine: ‘The system will crush me anyway,’” Chowdhury said.
Chowdhury calls this concept “moral outsourcing,” a phenomenon that enables each person within the community to pass responsibility on to the whole. Unlike AI, however, humans are more than cogs in machines and have the capacity for independent thought and action. The data scientist argues that responsibility in AI should not just be given to the industry at large, but every participating individual.
“We need to empower people to speak their minds and have an ethical conscience,” Chowdhury stated.
In a market obsessed with innovation, the incorporation of social responsibility in development might sound like restriction to some. Though the ethical importance of conscious AI is arguably reason enough, regulation has benefits on business innovation and the community at large, according to Chowdhury.
“Brakes help a car go faster,” she noted. “If we have the right kinds of guard rails to tell us if something is going to get out of control, we feel more comfortable taking risks. It sounds contradictory, but if I know where my safe space is, I’m more capable of making true innovations.”
At AI’s current scale, it seems warnings of the widespread ramifications of bias don’t have quite the impact necessary to incite the shift toward the social awareness AI needs. Responsible AI strategies start with a fundamentally ethical business culture and more comprehensive insights that can identify potential unintended consequences of technology, Chowdhury pointed out.
An effective method for expanding corporate perspectives is the inclusion of diverse, interdisciplinary voices. “Often technologists will say, and rightfully so, ‘How was I supposed to know thing X would happen?'” she said. “It’s something very specific to a neighborhood or a country or a socio-economic group. What you should do is bring in a local community, the ACLU, some sort of a regional expert.”
At Accenture, Chowdhury and her team have implemented the use of an AI Fairness Tool as a part of the company’s machine-learning transparency initiative. The tool addresses quantifiable AI bias issues by identifying sensitive variables, such as age, gender and race, and adjusting for them in the statistical models that guide AI technologies.
Bias is a sensitive issue, and the Fairness Tool takes on the responsibility of calling attention to potential inequities objectively. “The way we think about it is not as a decision maker, but a decision enabler … to explain the potential flaws and problems and then take collective action,” Chowdhury said. “It helps smooth conversation and pinpoint where there might be unfairness in your algorithm.”
An ethical, inclusive business is proven to have more successful teams that create better products and rank higher in the market at large. While AI may empower organizations to pursue unlimited innovation, the long game in AI success for both businesses and communities requires a strategy built on social responsibility.
“If we build something that is fundamentally unethical, we need to stop and [say], ‘Just because we can doesn’t mean we should,’” she said.
Here’s the complete video interview, part of SiliconANGLE’s and theCUBE’s coverage of Accenture Technology Vision 2019.
THANK YOU