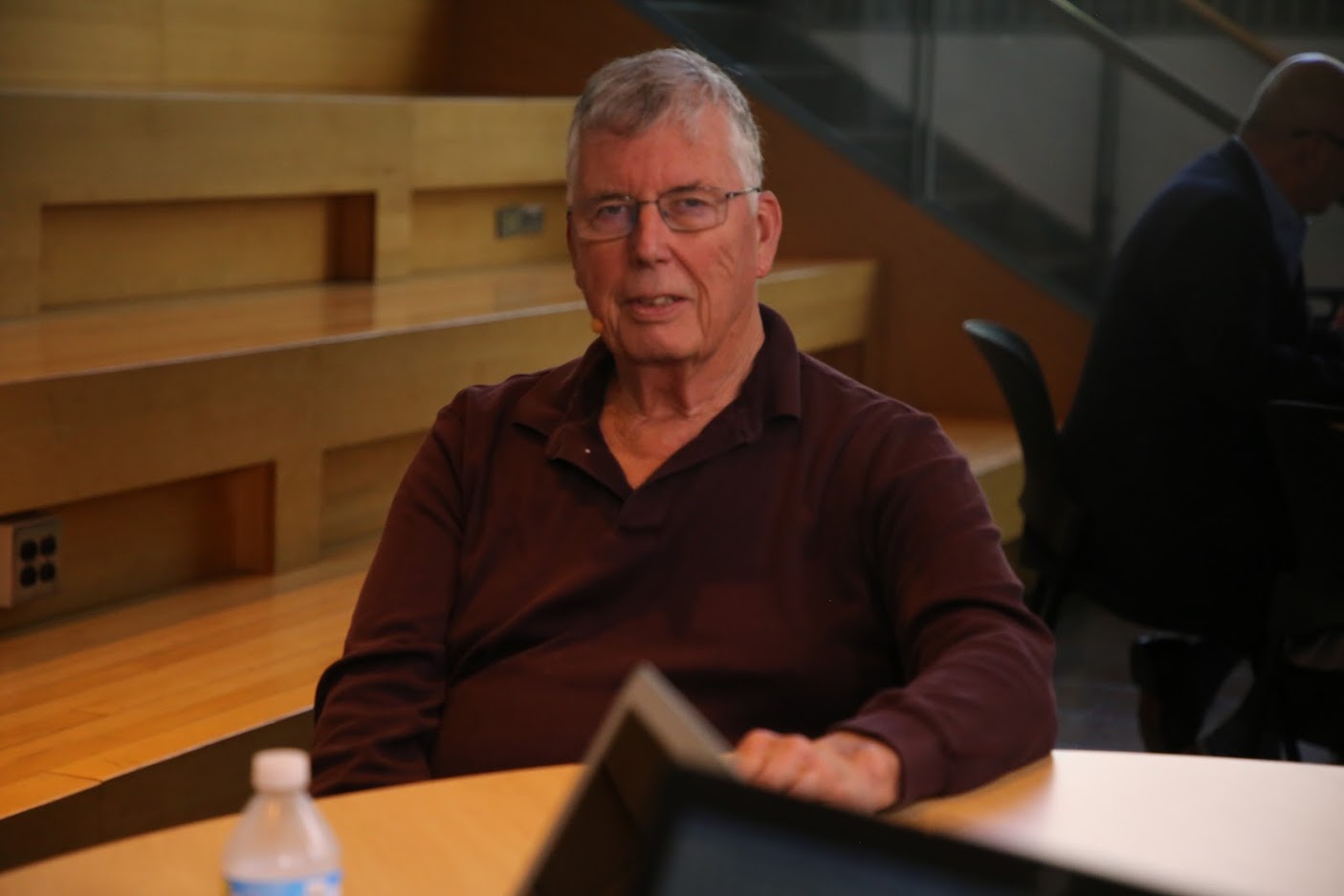
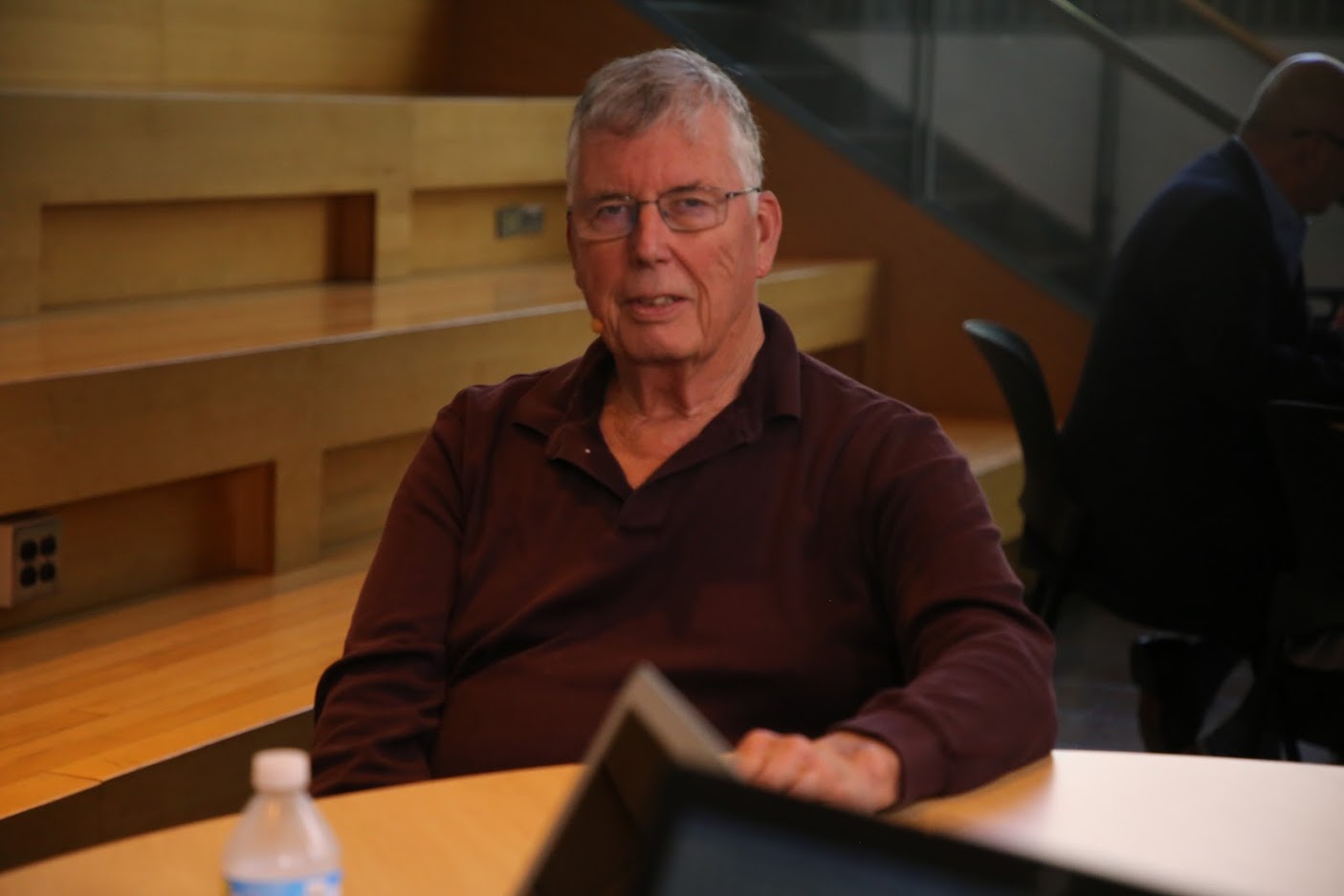
Why do so many companies still struggle to build a smooth-running pipeline from data to insights? They invest in heavily hyped machine-learning algorithms to analyze data and make business predictions.
But then, inevitably, they realize that algorithms aren’t magic: If they’re fed junk data, their insights won’t be stellar. So they employ data scientists who spend 90% of their time washing and folding in a data-cleaning laundromat, leaving just 10% of their time to do the job for which they were hired.
What’s also flawed about this process is that companies only get excited about machine learning for end-of-the-line algorithms. They should apply machine learning just as liberally in the early cleansing stages instead of relying on people to grapple with gargantuan data sets, according to Andy Palmer, co-founder and chief executive officer of Tamr Inc., which helps organizations use machine learning unify their data silos.
Lots of companies have spent large amounts of money on systems for big data collection. Their emphasis on data quantity over quality is readily apparent. “Anybody that’s worked at one of theses big companies can tell you that the data that they get from most of their internal systems sucks, plain and simple,” Palmer said.
Palmer and Michael Stonebraker (pictured), co-founder and chief technology officer of Tamr, spoke with Dave Vellante and Paul Gillin, co-hosts of theCUBE, SiliconANGLE Media’s mobile livestreaming studio, which covered the recent MIT CDOIQ Symposium in Cambridge, Massachusetts. They discussed machine learning in big-data cleansing and why Tamr not surprisingly believes startups offer better, more scalable big-data solutions than do legacy companies (see the full interviews with transcripts here and here).
This week, theCUBE spotlights Tamr Inc. in its Startup of the Week feature.
Palmer and Stonebraker have been trying to deflate the big-data hype bubble for years. All the way back in 2007, they predicted that the Apache Hadoop big-data framework wasn’t going to deliver the results so many expected of it.
“Mike actually was really aggressive in saying that it was going to be a disaster,” Palmer said.
It’s not that large data sets are bad. They’re obviously necessary for training analytics models and artificial intelligence. It’s the notion that as long as data is big, the rest of the analytics or AI pieces will fall into place that’s left so many companies disillusioned.
Organizations now realize that data quality is not negligible. They also know that a data scientist shouldn’t have to spend 80% to 90% or more of his or her time cleansing and wrangling data. There has to be a better, faster way to get data ready for use in analytics and AI.
The answer is to start looking at machine learning as a highly practical tool for doing these bulky, unglamorous tasks, according to Palmer. So many vendors use machine learning to make more appealing the marketing of software for prediction, recommendation engines, etc. Tamr is using it for the least glamorous thing there is: cleansing and organizing big data before anyone analyzes, predicts, markets or sells anything with it.
Here’s the complete video interview with Palmer:
The market is not exactly lacking proposed solutions to the data-swamp problem. Plenty of tech companies are bringing them out or updating their original offerings. The main technologies typically used in these systems, however, have a key deficiency, Stonebraker pointed out. These traditional technologies include extract, transform, load systems and master data management systems.
“A dirty, little secret is that technology does not scale,” Stonebraker said.
ETL is based on the premise that someone really bright will come up with a global data model for all data sources a user wants. Then a human interviews each business unit to see what data they’ve got, how to get it in the global data model, load it into the data warehouse and so on. Processes that are that human intensive tend to not scale, according to Stonebraker. They typically wind up with 10 or 20 sources integrated in the data warehouse, he added.
Is that a sufficient number? Let’s look at a real-world company. Tamr customer Toyota Motor Europe has distributors in different countries (sometimes cantons). If someone buys a Toyota in Spain and then moves to France, the French company knows nothing about the car owner.
In total, TME has 250 separate customer databases with 40 million total records in 50 languages. The company is in the process of integrating them into a single customer database to solve this customer-servicing issue. Machine learning provides a plausible means to do this. “I’ve never seen an ETL system capable of dealing with that kind of scale,” Stonebraker said.
The reason MDM doesn’t scale is basically because it’s rules-based, Stonebraker explained. Another Tamr customer, General Electric Co., wants to do spend analytics. It had 20 million spend transactions from the year before last. It tried to classify all of those into a rules-based hierarchy.
“So GE wrote 500 rules, which is about the most any single human can get their arms around,” he said. “That classified 2 million of the 20 million transactions. You’ve now got 18 to go. And another 500 rules is not going to give you 2 million more.
That, he noted, is the law of diminishing returns. “You’re going to have to write a huge number of rules that no one can possibly understand,” Stonebraker said. “If you don’t use machine learning, you’re absolutely toast.”
Here’s the complete video interview with Stonebraker:
Machine learning isn’t a silver bullet, Stonebraker conceded. Becoming truly data-driven requires both technological and cultural adjustments. In fact, 77% of surveyed executives said business adoption of big data/AI initiatives is difficult for their organizations, according to a NewVantage Partners LLC study. That’s up from last year despite plenty of new software flooding the market.
These executives cited a number of obstacles holding back adoption, 95% of which were cultural or organizational, rather than technological. “Organizations … need a plan to get to production. Most don’t plan and treat big data as technology retail therapy,” Gartner Inc. analyst Nick Heudecker has said.
Still, technology counts and likely shapes culture to some degree and vice versa. The above cases show how a data scientist could spend upwards up 90% of the time sifting and sorting — rather than helping actual hybrids get serviced or gas turbines developed. Machine learning is the way forward if big data is going to be practical for real-world businesses, according to Stonebraker.
“You’ve got to replace humans with machine learning … people are understanding that, at scale, traditional data-integration technologies just don’t work,” he said.
Younger companies are figuring this out and building machine learning into the core of their products. “The traditional vendors, by and large, are 10 years behind the times, and if you want cutting-edge stuff, you’ve got to go to startups,” Stonebraker said.
Does this “cutting-edge” stuff provide an easy route to data monetization? Will it make up for the years spent in frustration wading through data swamps? We are entering a phase where data will be made “consumable” much more quickly, Palmer pointed out.
“Will this phase be the one that finally meets the high expectations that were set 20, 30 years ago with enterprise data warehousing?” he said, “I don’t know. But we’re certainly getting closer to it.”
THANK YOU