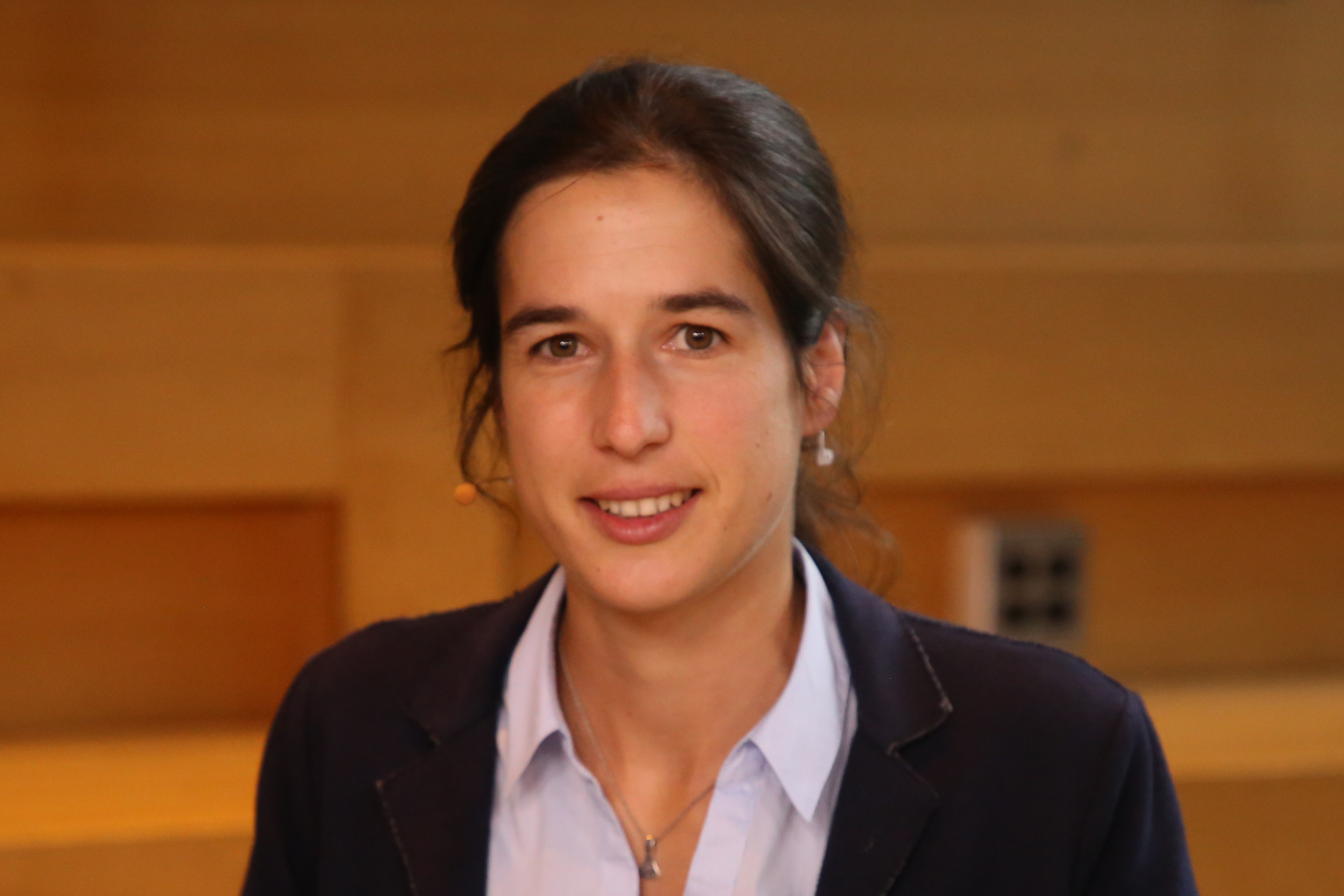
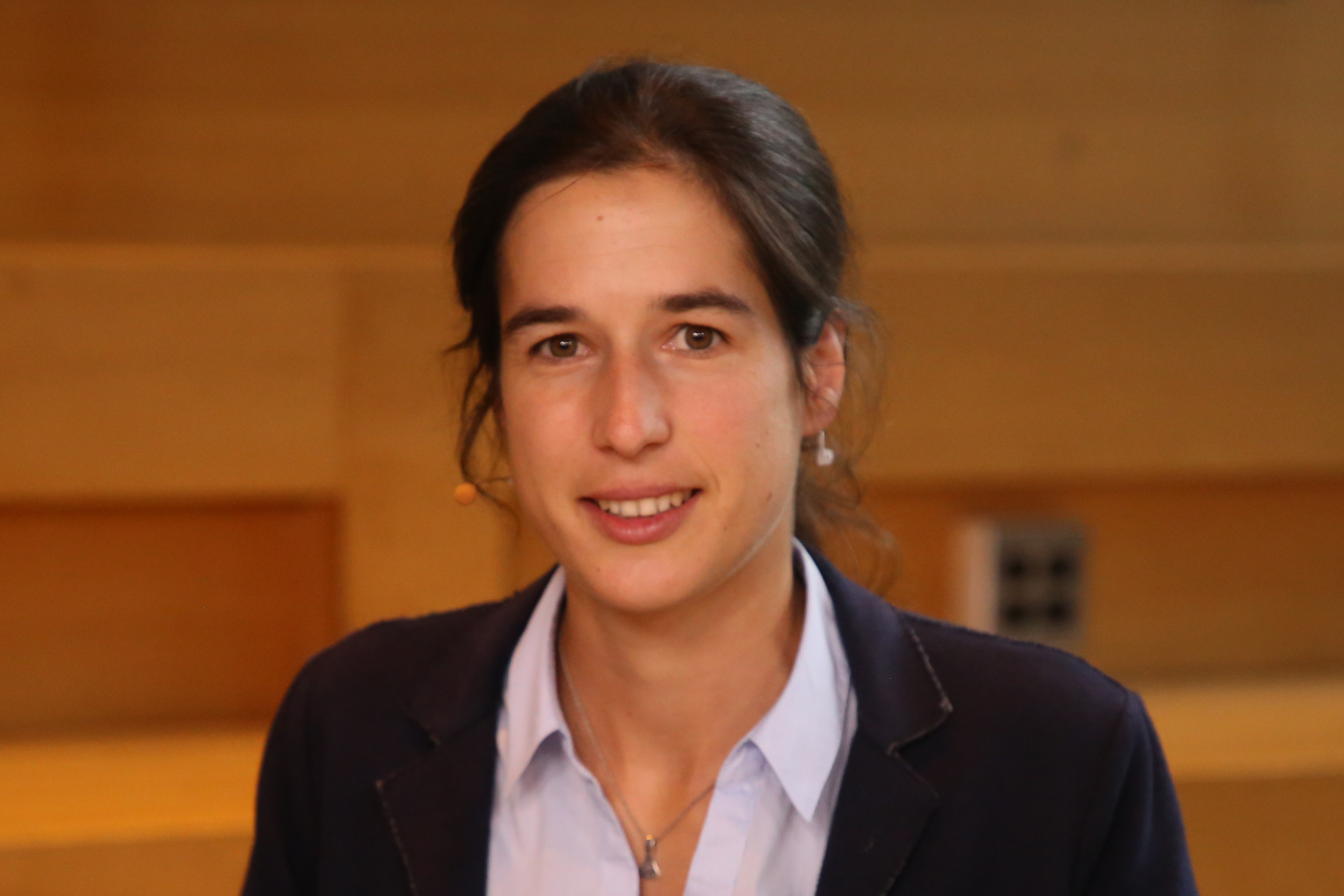
Data is a critical resource. Its insights drive operational and strategic decisions not only for big-data behemoths such as Google, Facebook and Amazon, but also a range of industries from jet engine manufacturers to major league basketball to agriculturalists who use data to increase crop yield.
Raw data as a resource is often compared to crude oil as a driver of economic change. Like crude oil, data is unusable in its natural state. The value is obtained only after refining the base product into a usable form. And as with oil, the quality of the output can vary.
But unlike petroleum-based products, data has no clear labeling system, meaning businesses are often blind as to whether they are operating on the data equivalent of 100-octane jet fuel or high-sulfur off-road diesel.
Statistics show that 84% of global chief executive officers are concerned about data standards, and flawed data costs U.S. businesses $15 million a year in losses. This has led to a proliferation of software tools to monitor data quality; some of which are of dubious quality themselves. Determining “how data quality measurement and monitoring is implemented in state-of-the-art data quality tools” has been documented in the just-released “Survey of Data Quality Measurement and Monitoring Tools.”
“The main motivation for this study was actually a very practical one,” said Lisa Ehrlinger (pictured), senior researcher at Johannes Kepler University and co-author of the study. “We spent the majority of time in [our] big-data projects on data quality measurement and improvement tasks. So, we [asked] what tools are out there on the market to automate these data quality tasks.”
Ehrlinger spoke with Dave Vellante and Paul Gillin, co-hosts of theCUBE, SiliconANGLE Media’s mobile livestreaming studio, during the MIT CDOIQ Symposium in Cambridge, Massachusetts. They discussed the research methods and the results of the study (see the full interview with transcript here).
This week, theCUBE spotlights Lisa Ehrlinger in its Women in Tech feature.
Ehrlinger has been at Johannes Kepler University in Linz, Austria, since her undergraduate days and holds both bachelor’s and master’s degrees in computer science from the university. She is currently working on her doctorate thesis on automated continuous data quality measurement under the supervision of Professor Dr. Wolfram Wöß from the Institute of Application-oriented Knowledge Processing at Johannes Kepler.
During her studies, Ehrlinger expanded her experience by working on information-technology projects for diverse employers. These include Oracle, software intelligence company Dynatrace LLC, the Roman Catholic Diocese of the city of Linz, Austria, and most recently the Software Competence Center Hagenburg.
In just the past four years, Ehrlinger has published her master’s thesis on “Data Quality Assessment on Schema-Level for Integrated Information Systems,” co-authored 10 additional research papers, and co-edited the conference proceedings for the Tenth International Conference on Advances in Databases, Knowledge, and Data Applications.
Ehrlinger was a featured speaker at the MIT CDOIQ Symposium, giving a talk inspired by her doctoral research titled “Automating Data Quality Measurement With Tools.”
Ehrlinger and her team identified 667 data quality tools on the market, and they then narrowed that number down to 13 for detailed testing and analysis based on their domain independence, non-specificity, and availability free or on a trial basis. Just over half (50.8%) of the tools were excluded because they were domain-specific; meaning they were dedicated to specific data types or proprietary tools.
“We just really wanted to find tools that are generally applicable for different kinds of data, for structured data, unstructured data, and so on,” Ehrlinger said.
Another 40% were excluded because they were dedicated to a specific management task, such as data visualization, integration or cleansing.
The tools selected had to offer three functionality areas identified by the research team as the most important: data profiling, quality metrics and quality monitoring: “Data profiling to get a first insight into data quality … data quality management in terms of dimensions, metrics and rules … [and] data quality monitoring over time,” Ehrlinger explained.
While the Gartner Magic Quadrant for Data Quality Tools is the best-known study in the field, it doesn’t look on specific measurement functionalities, according to Ehrlinger. Her research team took a full year to go hands-on with the tools, gaining firsthand experience using them.
Another difference between Ehrlinger’s team and the Gartner study was the range of tools evaluated. The final 13 tools selected by Ehrlinger included nine commercial and closed-source tools, of which four — Informatica Data Quality, Oracle Enterprise Data Quality, SAS Data Quality, and Talend Open Studio for Data Quality — were listed as leaders in Gartner’s Magic Quadrant.
The other five tools evaluated in the study were free and open-source, and only one of these — Talend — was mentioned by Gartner. The other four were OpenRefine, Aggregate Profiler, Moby DQ and Apache Griffin, “which has really nice monitoring capabilities but lacks some other features from these comprehensive tools,” Ehrlinger stated.
As well as functionality, customer service was considered in the overall evaluation stakes. “The focus was on the feature function, but of course we had to contact the customer support,” Ehrlinger said.
This was especially true for the commercial tools. “We had to ask them to provide us with some trial licenses, and there we perceived different feedback from those companies,” Ehrlinger said.
She also quizzed attendees at a data quality event on their customer experiences: “It was interesting to get feedback on single tools and verify our results, and it matched pretty good,” she said.
Winners in the customer service stakes were Informatica Data Quality and Experian Pandora. “We perceived a really close interaction with [Informatica] in terms of support, trial licenses, and specific functionality,” Ehrlinger said.
Other companies, such as IBM, did not score as highly. “They focus on big vendors,” she added.
One result that surprised Ehrlinger and her team was that many of the tools lacked automation. “We think that there is definitely more potential for automation,” she said.
Another area where the tools required improvement was detailed information. “We observed some tools that say … ‘we apply machine learning,’ and then you look into their documentation and find no information [on] which algorithm, which parameters, which thresholds,” Ehrlinger said. “If you want to assess the data quality, you really need to know what algorithm, and how is it attuned.”
This is especially important because the users of these tools generally have a high level of technical expertise. “He or she really needs to have the possibility to tune these algorithms to get reliable results and to know what’s going on and why, which records are selected,” she added.
Ehrlinger and her research team have already started their next study, titled “Knowledge Graph for Data Quality Assessment.” This project ties in with the current trend for enterprise-grade automation, tackling “two problems at once,” according to Ehrlinger.
“The first is to come up with a semantic representation of your data landscape in your company,” she said. “But not only the data landscape itself in terms of gathering metadata, but also to automatically improve or annotate this data schema with data profiles.”
Here’s the complete video interview, part of SiliconANGLE’s and theCUBE’s coverage of the MIT CDOIQ Symposium:
THANK YOU