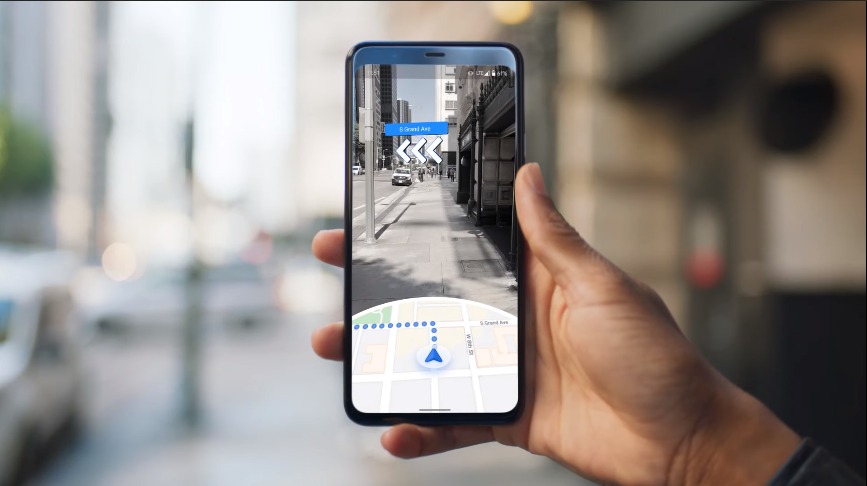
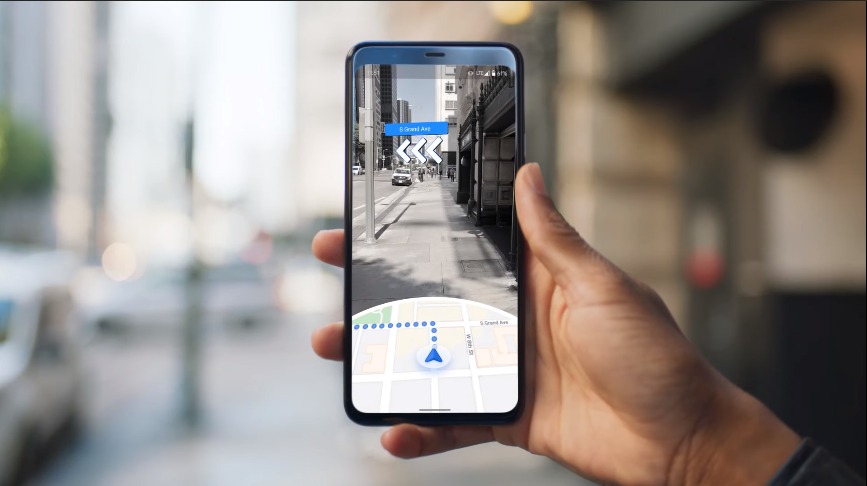
DeepMind, Alphabet Inc.’s artificial intelligence research unit, today detailed new machine learning technology it has developed to make Google Maps more useful.
Maps has more than a billion users worldwide who rely on the service to plan their travel routes. One of the service’s most central features is its ability to generate time of arrival estimates, helping drivers view key information such as how soon they need to depart to catch a train.
DeepMind teamed up with sister company Google LLC to reduce inaccuracies in time of arrival estimates. Their collaboration, the unit detailed this morning, has produced a double-digit reduction in the percentage of inaccuracies. In one case, prediction errors dropped by no less than 51%.
DeepMind achieved this improvement by implementing a so-called “graph” neural network in Maps to help with arrival time estimation. A graph is a data structure that stores data points and the relationships between them in the form of interconnected dots. This structure, DeepMind has found, lends itself well to capturing the interconnected nature of road systems.
“For example, think of how a jam on a side street can spill over to affect traffic on a larger road,” DeepMind engineers Oliver Lange and Luis Perez detailed in a blog post. Because the AI can capture an integrated view of multiple road segments and intersections, “the model gains the ability to natively predict delays at turns, delays due to merging, and the overall traversal time in stop-and-go traffic,” they explained.
The company had to overcome multiple technical challenges before it could start using its graph neural network to estimate arrival times. One of the main issues was how to train the AI.
Developing neural networks involves training them on sample data similar to the data that they’ll be expected to process in the real world. If an AI will be tasked with analyzing photos of giraffes, it needs to be trained on photos of giraffes.
But the process wasn’t as simple in the case of Maps because of differences in the way roads are built. An AI that is trained to estimate the duration of highway trips won’t necessarily be capable of doing the same for urban roads, and much smaller differences can cause accuracy issues as well.
DeepMind solved the challenge by taking advantage of its neural network’s graph structure. The unit’s engineers organized the road data that the AI processes to estimate arrival times into “Supersegments” also based on a graph structure, much like the AI itself. These Supersegments are sufficiently flexible that DeepMind’s neural network managed to overcome training data differences.
The AI research performed by companies such as Google in many cases not only helps improve their own products but also advances the field as a whole. DeepMind, and the AI research groups at other tech giants, often share their work with the world in academic papers.
THANK YOU