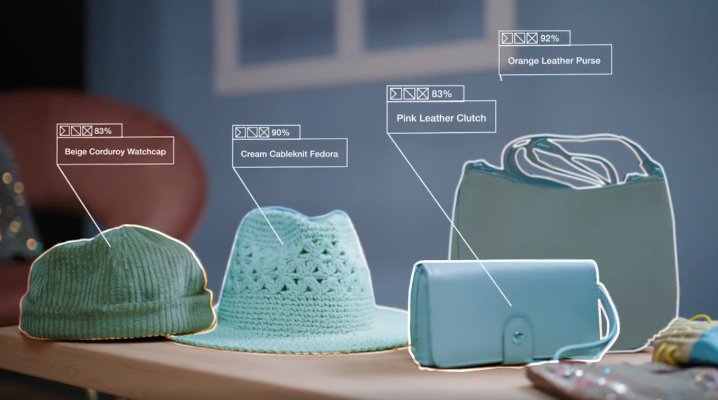
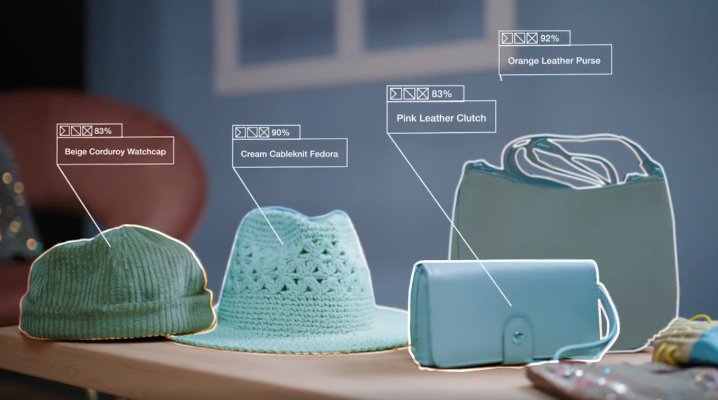
Facebook Inc. today debuted Dynabench, a research tool it hopes will allow computer scientists develop more powerful natural-language processing models.
To build cutting-edging neural networks that advance the state of the art, researchers need a way of comparing their models with those developed by peers. Accurate comparisons are a prerequisite to verifying that a new model is indeed better than existing entries into the field. This process is known as benchmarking.
With Dynabench, Facebook hopes to address shortcomings it sees in current benchmarking methods and facilitate the creation of more robust artificial intelligence software.
Researchers most commonly assess their models using test data sets, essentially collections of standardized questions. Several such tests datasets exist in the natural-language processing field. The issue is that, because of the rapid pace at which AI models are improving, tests can become outdated over time, leaving researchers without a reliable means of assessing a neural network’s accuracy and comparing it with existing ones.
Enter Dynabench. Facebook’s solution to the challenge is to crowdsource the benchmarking process partially by bringing human testers into the loop. The idea is that humans can more accurately assess a model’s accuracy than a set of pre-packaged test questions by coming up with harder, more creative challenges for the neural network.
Dynabench “measures how easily AI systems are fooled by humans, which is a better indicator of a model’s quality than current static benchmarks provide,” explained Facebook researchers Douwe Kiela and Adina Williams. “This metric will better reflect the performance of AI models in the circumstances that matter most: when interacting with people, who behave and react in complex, changing ways that can’t be reflected in a fixed set of data points.”
When an AI completes a round of testing, Dynabench identifies the questions that fooled the model and compiles them into a new test dataset. Researchers can use this dataset to help them build newer, more sophisticated models. Then, once a model is developed that can answer the questions the first AI couldn’t, Dynabench repeats the process and compiles another test dataset with even harder questions.
The goal is to create a “virtuous cycle of progress in AI research,” as Facebook’s Kiela and Williams put it.
Having a more reliable tool for assessing model accuracy could benefit not only researchers but also enterprises that use AI in their applications. If enterprise software engineers have a clearer view of how well different AI models handle a given task, they more effectively pick the AI most suitable for their application from the countless available models out there. That, in turn, can translate to a better user experience and fewer costly errors.
THANK YOU