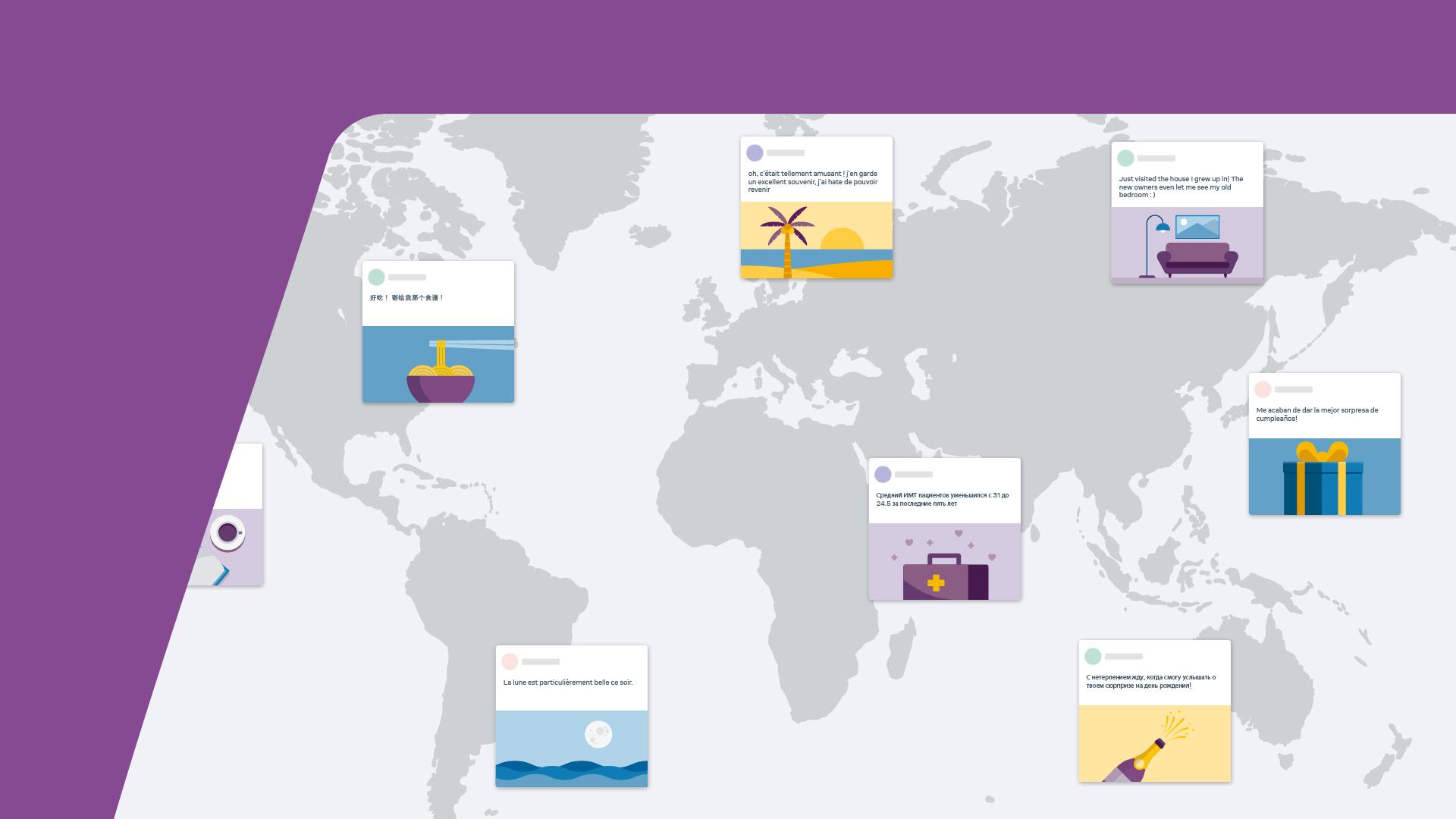
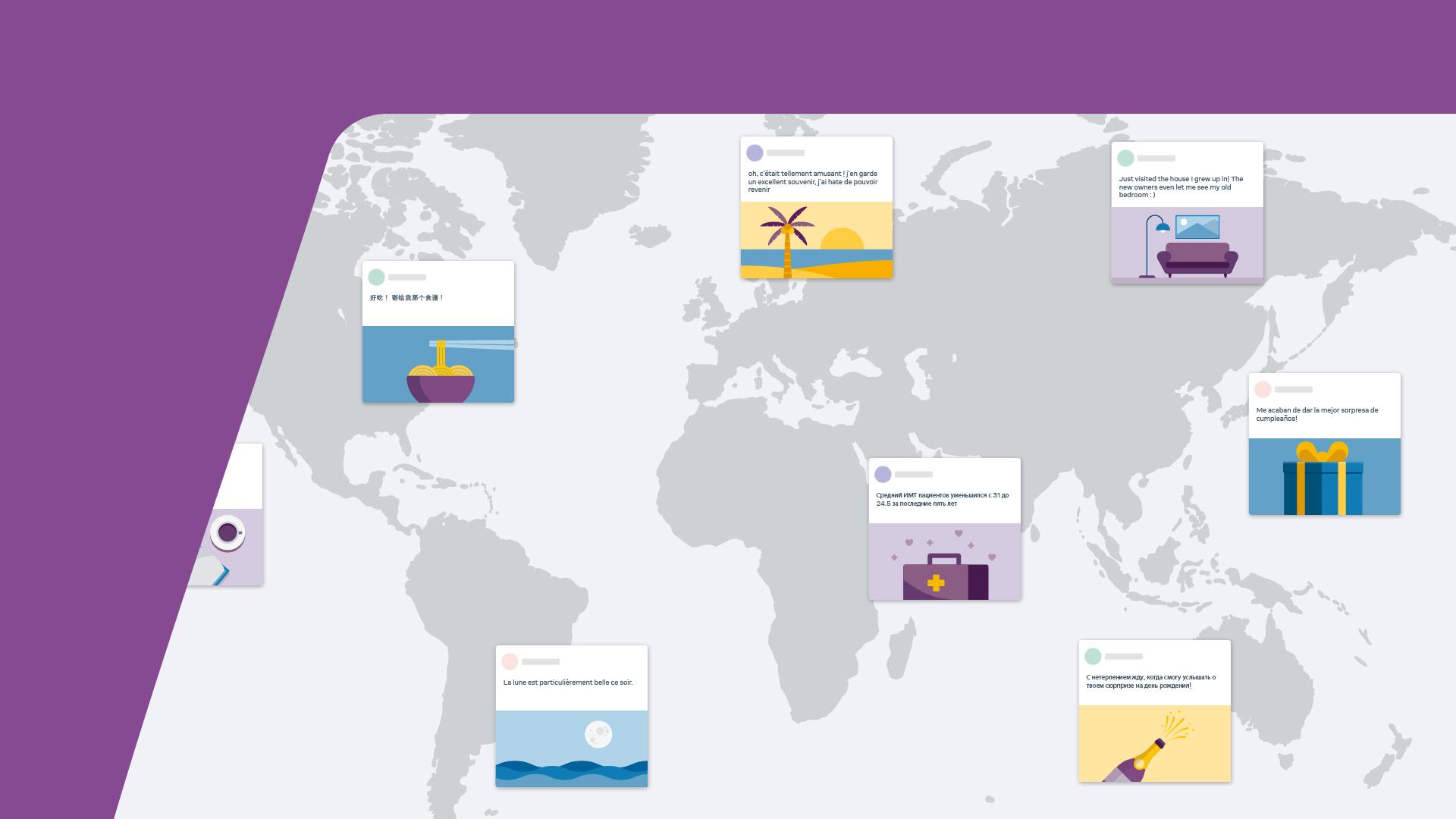
Facebook Inc. said today it has made substantial progress in using machine learning to generate more accurate translations between any two languages without relying on English-language data.
The company is open-sourcing its latest creation, M2M-100, which it says is the first multilingual machine translation model that can translate directly between any pair of 100 languages.
Up until now, most multilingual machine translation models have relied on the English language as a kind of intermediary when translating between two different languages, because of the wide availability of English training data. So, for example, when a model translates a sentence from French to Chinese, it would first translate the French into English, and from there into Chinese. These models work well enough in most cases but are often inaccurate when it comes to more complex sentences and phrases.
Facebook said M2M-100 can better preserve meaning by translating directly from Chinese to French, or between any of more than 100 language pairs, without using English as an intermediary.
Translating between so many different language pairs is not an easy task, since the models need access to lots of high-quality training data. In a blog post, Facebook AI researcher Angela Fan explained how she and her team set about creating a massive “many-to-many” dataset containing more than 7.5 billion sentences in 100 different languages. This data was gathered using open-source data mining tools such as ccAligned, ccMatrix and LASER, and then split into 14 distinct language groups based on parameters such as linguistic classification, geography and cultural similarities.
“People living in countries with languages of the same family tend to communicate more often and would benefit from high quality translations,” Fan said. “For instance, one group would include languages spoken in India, like Bengali, Hindi, Marathi, Nepali, Tamil or Urdu. We systematically mined all possible language pairs within each group.”
Within each of those 14 language groups, Facebook then identified one to three “bridge languages” for each one to serve as the basis of its translations into different language groups.
“We then mined parallel training data for all possible combinations of these bridge languages,” Fan said. “Using this technique, our training dataset ended up with 7.5 billion parallel sentences of data, corresponding to 2,200 directions. Since the mined data can be used to train two directions of a given language pair (e.g. en->fr and fr->en), our mining strategy helps us effectively sparsely mine to best cover all 100×100 (a total of 9,900) directions in one model.”
Fan’s team also used a technique known as “back translation” to create synthetic data to the supplement the already mined parallel data.
“Overall, the combination of our bridge strategy and back translated data improved performance on the 100 back translated directions by 1.7 BLEU on average compared to training on mined data alone,” Fan said. “With a more robust, efficient, and high-quality training set, we were well-equipped with a strong foundation for building and scaling our many-to-many model.”
Fan said the finished M2M-100 model is able to translate with much greater accuracy than the existing English-centric multilingual models that Facebook currently uses, outperforming those systems by 10 points on the widely used BLEU metric scale used to evaluate machine translations. Facebook ultimately wants to replace those models with M2M-100 in order to improve the quality of translations for its millions of users that speak low-resource languages.
“We’ll continue to improve our model by incorporating such cutting-edge research, exploring ways to deploy MT systems responsibly, and creating more specialized computation architectures necessary to bring this to production,” Fan said.
THANK YOU