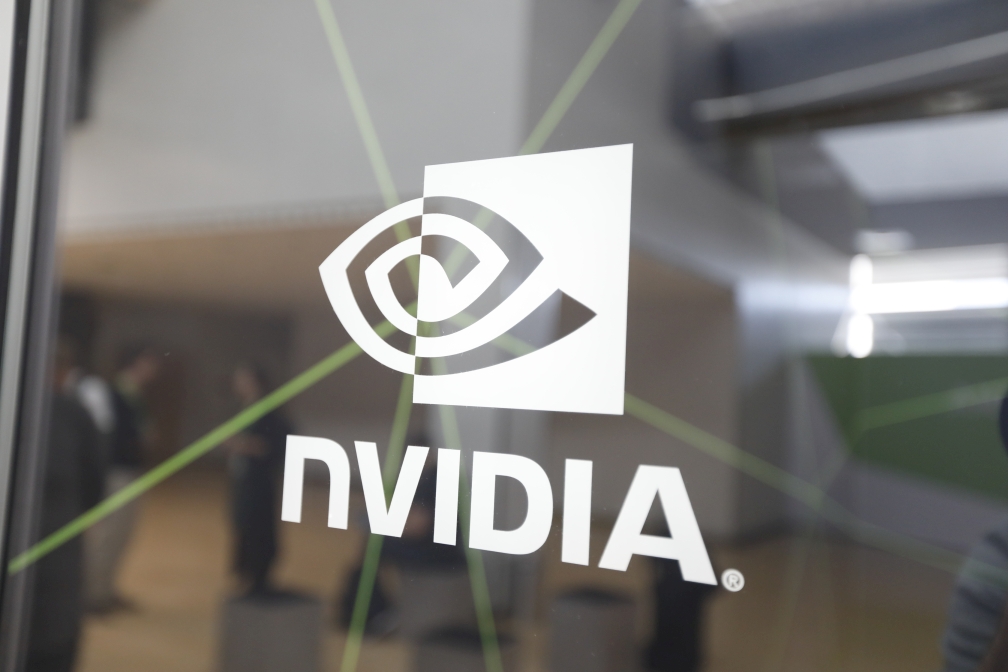
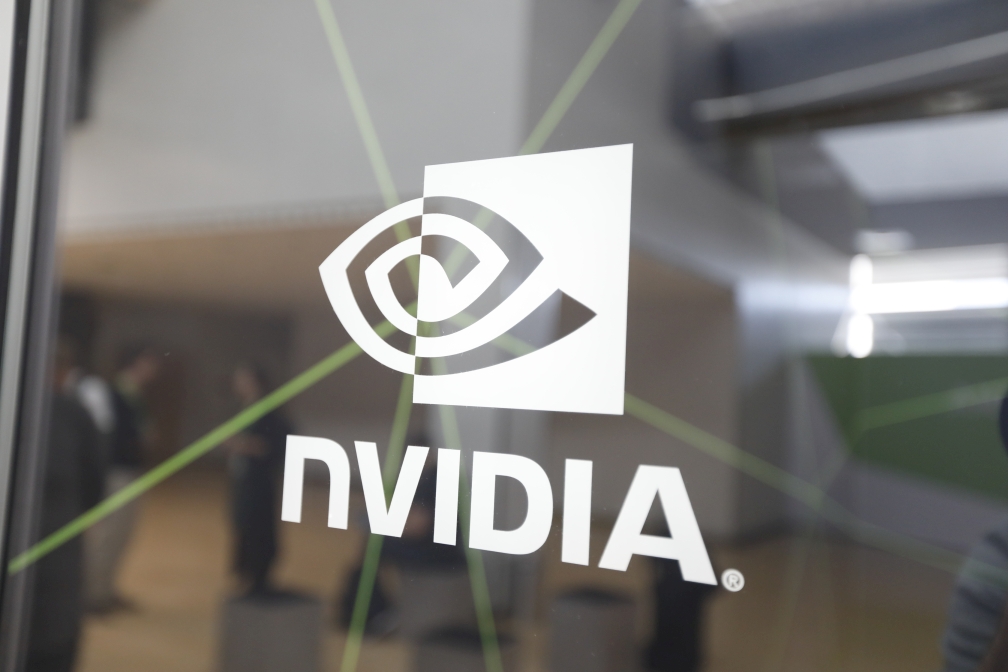
Nvidia Corp. today introduced a new version of its NeMo Megatron artificial intelligence development tool that will enable software teams to train neural networks faster.
In particular, the update promises to reduce the amount of time necessary to train advanced natural language processing models.
In 2020, AI research group OpenAI LLC debuted a sophisticated natural language processing model dubbed GPT-3. The model can perform a variety of tasks ranging from translating text to generating software code. OpenAI provides a commercial cloud service that enables companies to access multiple, specialized editions of GPT-3, as well as create their own custom versions.
NeMo Megatron, the AI development tool that Nvidia updated today, now includes features optimized for training GPT-3 models. Nvidia expects the features to facilitate an up to 30% reduction in training times.
“Training can now be done on 175 billion-parameter models using 1,024 NVIDIA A100 GPUs in just 24 days — reducing time to results by 10 days, or some 250,000 hours of GPU computing, prior to these new releases,” Nvidia researchers detailed in a blog post today.
The speedup is primarily the result of two features known as sequence parallelism and selective activation recomputation. According to Nvidia, each feature speeds up AI training in a different way.
AI models such as GPT-3 consist of software building blocks known as layers. Each layer performs one portion of the calculations that a neural network uses to turn data into insights. A common approach to speeding up AI training is to configure a neural network’s layers such that calculations can be carried out in parallel rather than one after one another, which saves time.
Sequence parallelism, the first capability added to NeMo Megatron today, uses the same approach to speed up processing. According to Nvidia, the new capability can parallelize calculations that could only be performed one after another before, thereby increasing performance. It also reduces the need to carry out the same calculations multiple times.
Selective activation recomputation, the other new capability in NeMo Megatron, further reduces the number of calculations that have to be repeated. It does so by optimizing computing operations known as activations that AI models use to process data. If the calculations involved in an activation have to be redone, NeMo Megatron can now do so more efficiently than before, which will reduce AI training times.
Another major enhancement to NeMo Megatron that Nvidia detailed today is the introduction of a hyperparameter optimization tool. Hyperparameters are configuration settings that software teams define for an AI model during development to optimize its performance. Using Nvidia’s new tool, software teams can automate some of the manual work involved in the task.
Developers can specify what latency or throughput levels an AI model should achieve and have the new tool automatically find the hyperparameters necessary to meet requirements. According to Nvidia, the feature is particularly useful for optimizing the AI training process. The company says that, in an internal test, its researchers managed to increase training throughput for a GPT-3 model by as much as 30%.
“We arrived at the optimal training configuration for a 175B GPT-3 model in under 24 hours,” Nvidia’s researchers detailed. “Compared with a common configuration that uses full activation recomputation, we achieve a 20%-30% throughput speed-up.”
THANK YOU