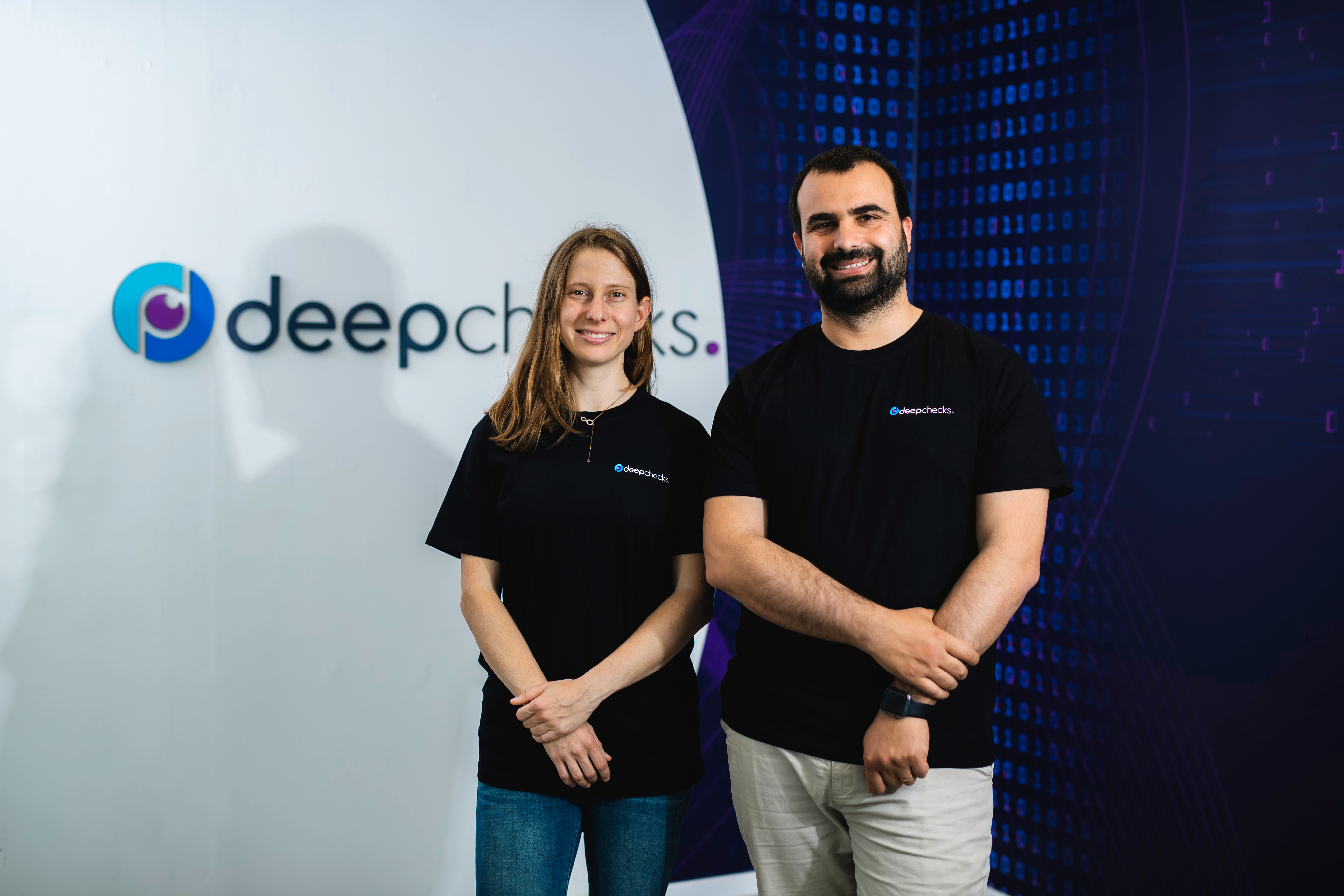
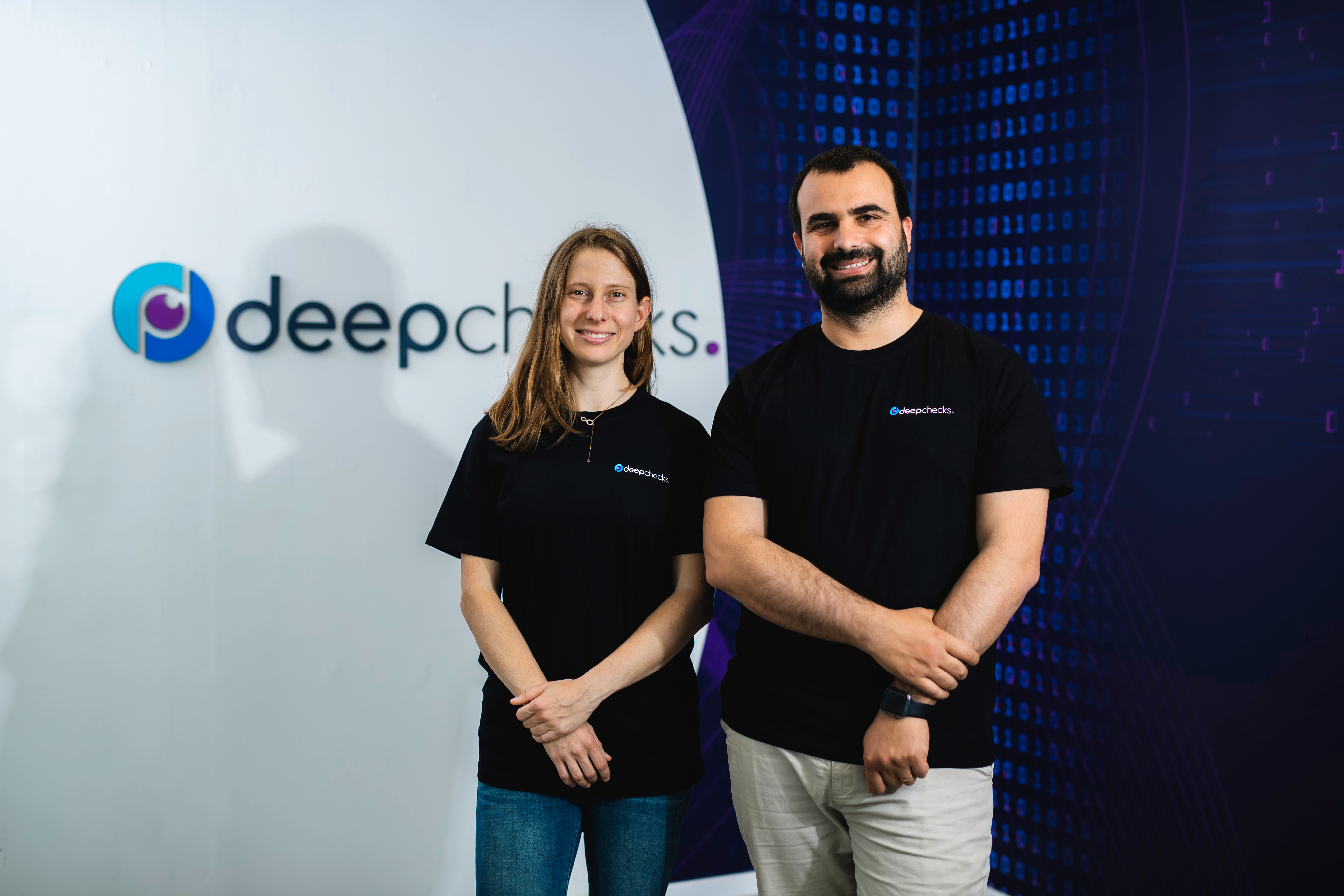
Continuous machine learning validation startup Deepchecks Ltd. has made its platform generally available today after closing on a $14 million seed funding round.
The round was led by Alpha Wave and included participation from Hetz Ventures and Grove Ventures.
Deepchecks was co-founded by Chief Executive Philip Tannor (pictured, right) and Chief Technology Officer Shir Chorev (left). It originally intended to be a traditional machine learning operations platform. However, they soon realized that a larger opportunity lay not in building machine learning models, but in validating them.
Machine learning today is all the rage, thanks to the rapid emergence of ChatGPT and other generative artificial intelligence models that are taking the technology world by storm. Suddenly, the focus has transitioned from long-term research projects to building actual products that are regularly deployed in production, similar to how software is deployed and continuously updated.
As Deepchecks explains, it took software development many years to establish the processes and tools required to deliver high-quality applications on time. Now, companies expect the same high quality machine learning models to be deployed and updated regularly, despite the technology being relatively new. Making things worse, machine learning development has more moving parts and is more opaque, which makes the process much less predictable.
Deepchecks says the only way to ensure high-quality machine learning models are regularly updated is to draw on the lessons learned from software development, and apply regular testing and validation. To that end, it has built a platform that helps MLOps teams gain visibility into their machine learning models at every step, from development to deployment to operation and production.
Tannor explained that DevOps teams would never deploy software that has not been systematically tested first. “Yet, this is what happens today with machine learning applications,” he said. “Deepchecks brings a new approach to MLOps, improving models by adding validation to every step of the machine learning lifecycle.”
With Deepcheck’s open-source platform, users can reuse and tailor specific components to holistically test machine learning models and the datasets used to train them. Its platform also includes monitoring tools, plus root cause analysis tools for production environments, accessible through a single, comprehensive user interface.
“Deepchecks introduces a community-driven MLOps framework that enables people from data scientists and developers to C-level executives to have a clear picture of how machine learning applications behave, from research to production,” said Chorev.
Constellation Research Inc. Vice President and Principal Analyst Andy Thurai said Deepchecks might be more focused, but it operates in a very crowded MLOps market, where its machine learning testing, validation, monitoring and root cause analysis tools are already widely available. “However, its automated CI/CD for ML models, which work by testing whether the model meets production KPIs regarding performance, train-test drift and data integrity, could be an intriguing option for some users,” he said.
That has proven to be the case so far, with Deepchecks’ open-source platform being downloaded more than 500,000 times by enterprises large and small, including Amazon Web Services Inc., Booking.com Inc. and Wix.com Ltd. The enterprise version of its platform, available today, offers additional security and collaboration features.
THANK YOU